Leveraging Image-To-Image Translation Generative Adversarial Networks For Face Aging
2019 IEEE INTERNATIONAL CONFERENCE ON ACOUSTICS, SPEECH AND SIGNAL PROCESSING (ICASSP)(2019)
摘要
Here, face images of a specific age class are translated to images of different age classes in an unsupervised manner that enables training on independent sets of images for each age class. In order to learn pairwise translations between age classes, we adopt the UNsupervised Image-to-image Translation framework that employs Variational AutoEncoders and Generative Adversarial Networks. By mapping face images of different age classes to shared latent representations, the most personalized and abstract facial characteristics are preserved. To effectively diffuse age class information, a pyramid of local, neighbour, and global encoders is employed so that the latent representations progressively cover an increased age range. The proposed framework is applied to the FGNET aging database and compared to state-of-the-art techniques and the ground truth. Appealing experimental results demonstrate the ability of the proposed method to efficiently capture both intense and subtle aging effects.
更多查看译文
关键词
face aging, adversarial training, latent space, image-to-image-translation, pyramid
AI 理解论文
溯源树
样例
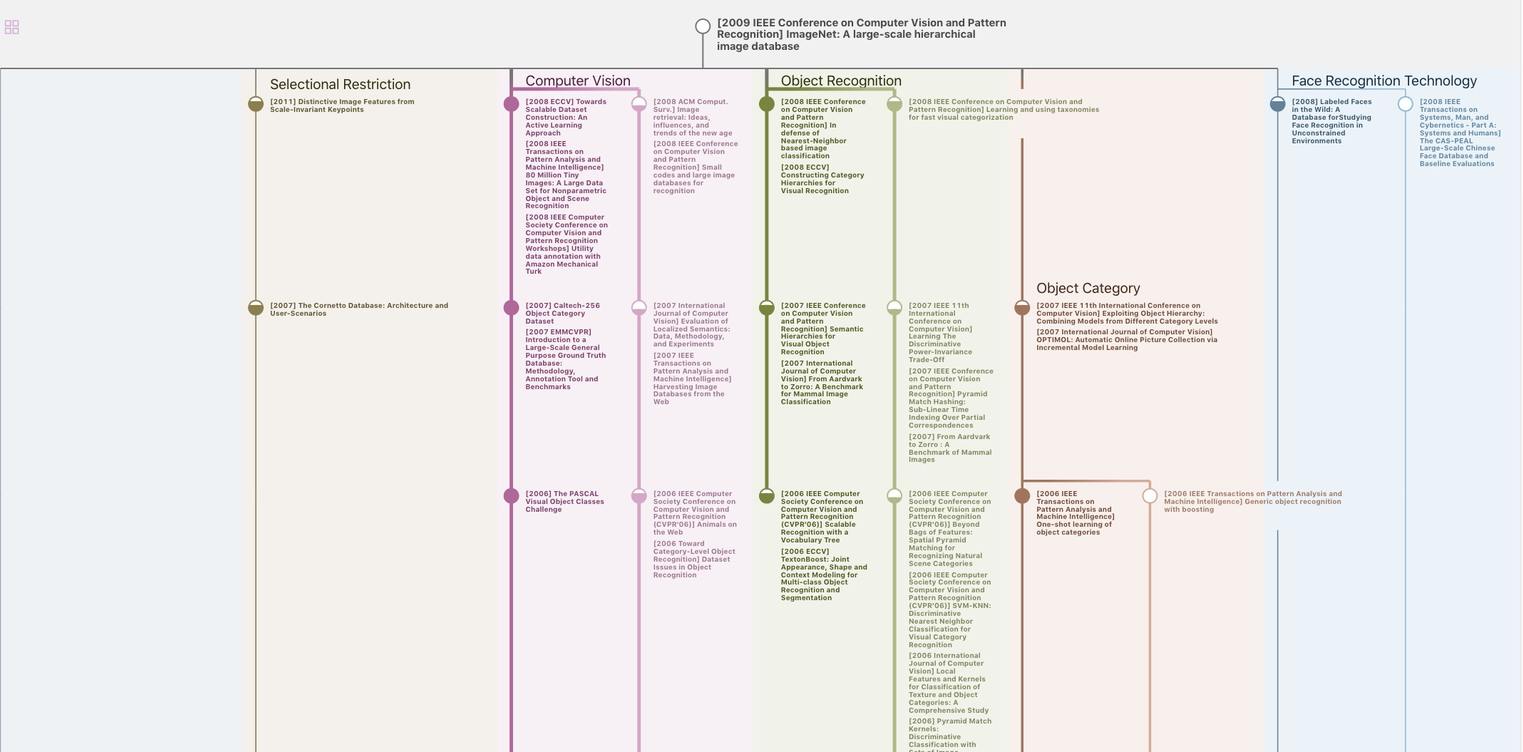
生成溯源树,研究论文发展脉络
Chat Paper
正在生成论文摘要