Fully Data-Driven Convolutional Filters With Deep Learning Models For Epileptic Spike Detection
2019 IEEE INTERNATIONAL CONFERENCE ON ACOUSTICS, SPEECH AND SIGNAL PROCESSING (ICASSP)(2019)
摘要
Epilepsy is a chronic disorder that causes unprovoked, recurrent-seizures. Characteristic spikes are often observed in the electroencephalogram (EEG) of epileptic patients in order to diagnose the disorder. Several methods have been investigated to automatically detect such spikes. The most common methods employ sub-band decomposition with discrete wavelet transform (DWT) or other filters to preprocess the EEG data before feeding it into a machine learning model. This paper introduces a fully data-driven method that automatically determines EEG frequency bands of interest. The raw signal is fed into a convolutional layer to detect suitable frequency bands, followed by a feedforward convolutional neural network (CNN) model or recurrent neural network (RNN) models for epileptic spike and non-spike classification. Fitting data of six patients, annotated by an epilepsy specialist, resulted in a convolutional layer with a frequency characteristic similar to bandpass filters. This result strongly justifies limiting the bandwidth of a signal, as done in previous studies. Moreover, results of the cross-subject validation indicate that a classical support vector machine with fixed preprocessing achieves comparable performance in the classification with fully data-driven models.
更多查看译文
关键词
epilepsy, spike detection, data-driven preprocessing, deep neural networks, electroencephalogram (EEG)
AI 理解论文
溯源树
样例
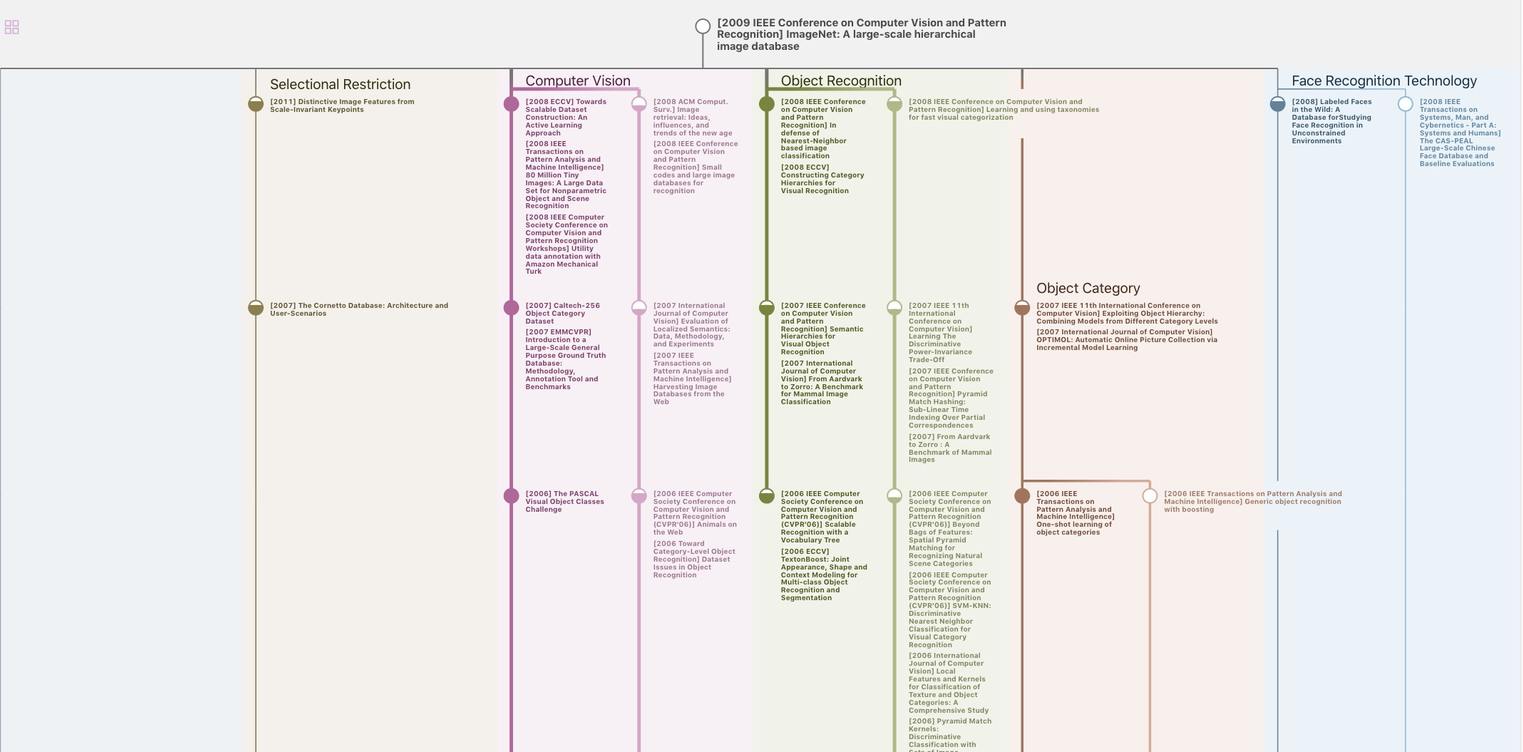
生成溯源树,研究论文发展脉络
Chat Paper
正在生成论文摘要