Speech Emotion Recognition Using Capsule Networks
2019 IEEE INTERNATIONAL CONFERENCE ON ACOUSTICS, SPEECH AND SIGNAL PROCESSING (ICASSP)(2019)
摘要
Speech emotion recognition (SER) is a fundamental step towards fluent human-machine interaction. One challenging problem in SER is obtaining utterance-level feature representation for classification. Recent works on SER have made significant progress by using spectrogram features and introducing neural network methods, e.g., convolutional neural networks (CNNs). However the fundamental problem of CNNs is that the spatial information in spectrograms is not captured, which are basically position and relationship information of low-level features like pitch and formant frequencies. This paper presents a novel architecture based on the capsule networks (CapsNets) for SER. The proposed system can take into account the spatial relationship of speech features in spectrograms, and provide an effective pooling method for obtaining utterance global features. We also introduce a recurrent connection to CapsNets to improve the model's time sensitivity. We compare the proposed model to previous published results based on combined CNN-long short-term memory (CNN-LSTM) models on the benchmark corpus IEMOCAP over four emotions, i. e., neutral, angry, happy and sad. Experimental results show that our model achieves better results than the baseline system on weighted accuracy (WA) (72.73% vs. 68.8%) and unweighted accuracy (UA) (59.71% vs. 59.4%), which demonstrates the effectiveness of CapsNets for SER.
更多查看译文
关键词
Speech Emotion Recognition, Capsule Networks, Spatial Relationship Information, Recurrent Connection, Utterance-level Features
AI 理解论文
溯源树
样例
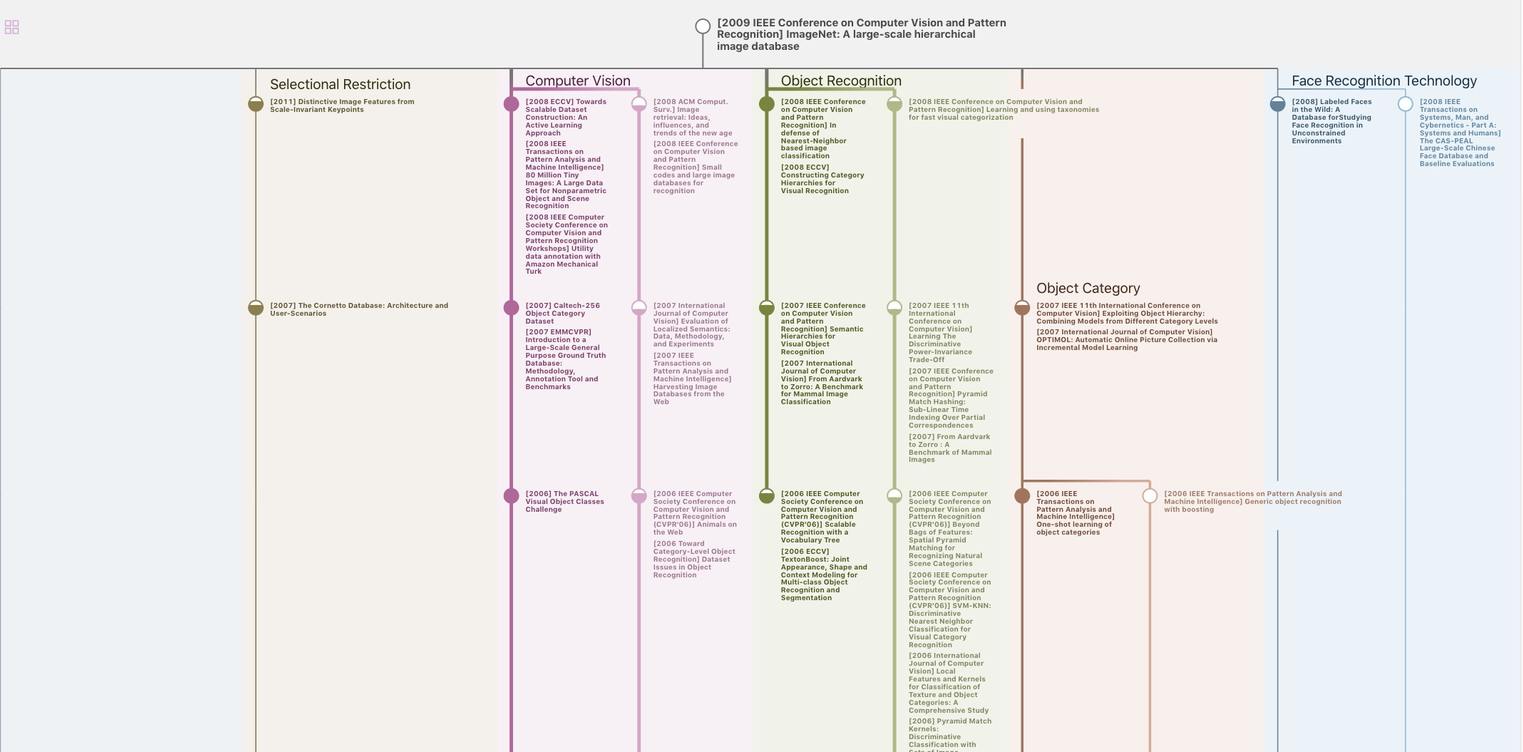
生成溯源树,研究论文发展脉络
Chat Paper
正在生成论文摘要