Cycle-Gans For Domain Adaptation Of Acoustic Features For Speaker Recognition
2019 IEEE INTERNATIONAL CONFERENCE ON ACOUSTICS, SPEECH AND SIGNAL PROCESSING (ICASSP)(2019)
摘要
It is well known that domain mismatch between the training and evaluation data hinders the performance of any machine learning system. Various factors contribute to domain mismatch. In speaker recognition systems, it mainly occurs due to the mismatch in recording conditions and language. Most speaker recognition corpora are telephone speech. Meanwhile, a few evaluation data sets like Speakers In The Wild ( SITW) are microphone speech. In this work, we explore domain adaptation at acoustic feature level by learning feature mappings between domains using cycle consistent generative adversarial networks ( cycle-GANs), without any parallel data between domains. Microphone features mapped to telephone domain are used to evaluate speaker recognition system trained only on telephone data. We achieved 9.37% and 2.82% relative improvement in equal error rate ( EER) and detection cost function ( DCF) on SITW eval set.
更多查看译文
关键词
Unsupervised domain adaptation, speaker recognition, cycle-GANs, generative adversarial neural networks (GANs)
AI 理解论文
溯源树
样例
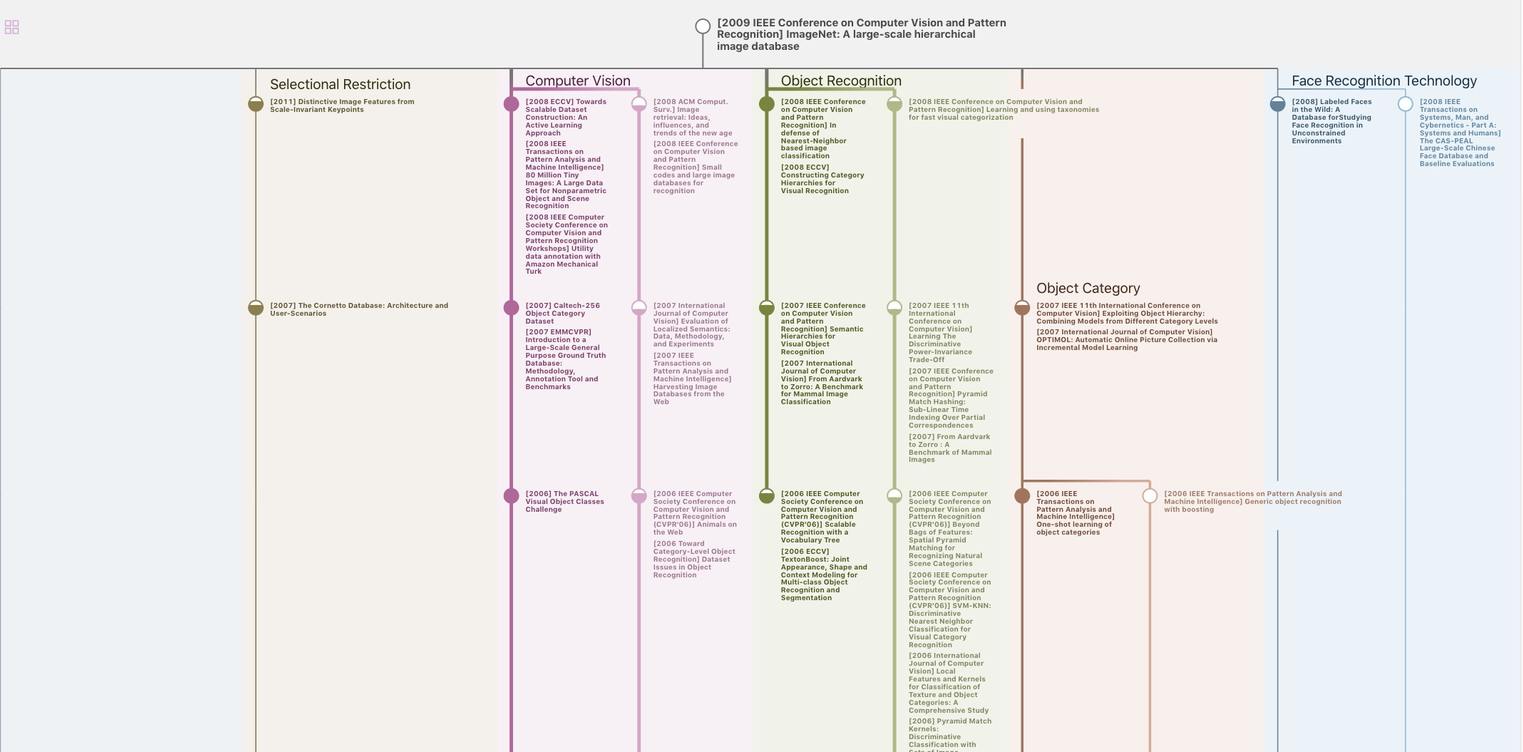
生成溯源树,研究论文发展脉络
Chat Paper
正在生成论文摘要