Exponential Collapse of Social Beliefs over Weakly-connected Heterogeneous Networks
IEEE International Conference on Acoustics, Speech, and Signal Processing(2019)
Abstract
We consider a distributed social learning problem where a network of agents is interested in selecting one among a finite number of hypotheses. The data collected by the agents might be heterogeneous, meaning that different sub-networks might observe data generated by different hypotheses. For example, some sub-networks might be receiving (or even intentionally generating) data from a fake hypothesis and will bias the rest of the network via social influence. This work focuses on a two-step diffusion algorithm where each agent: i) first updates individually its belieffunction using its private data; ii) then computes a new belief function by exponentiating a linear combination of the log-beliefs of its neighbors. We obtain analytical formulas that reveal how the agents' detection capability and the network topology interplay to influence the asymptotic beliefs of the agents. Some interesting behaviors arise, such as the “mind-control” effect or the “truth-is-somewhere-in-between” effect.
MoreTranslated text
Key words
Social learning,weakly-connected networks,Bayesian update,diffusion strategy
AI Read Science
Must-Reading Tree
Example
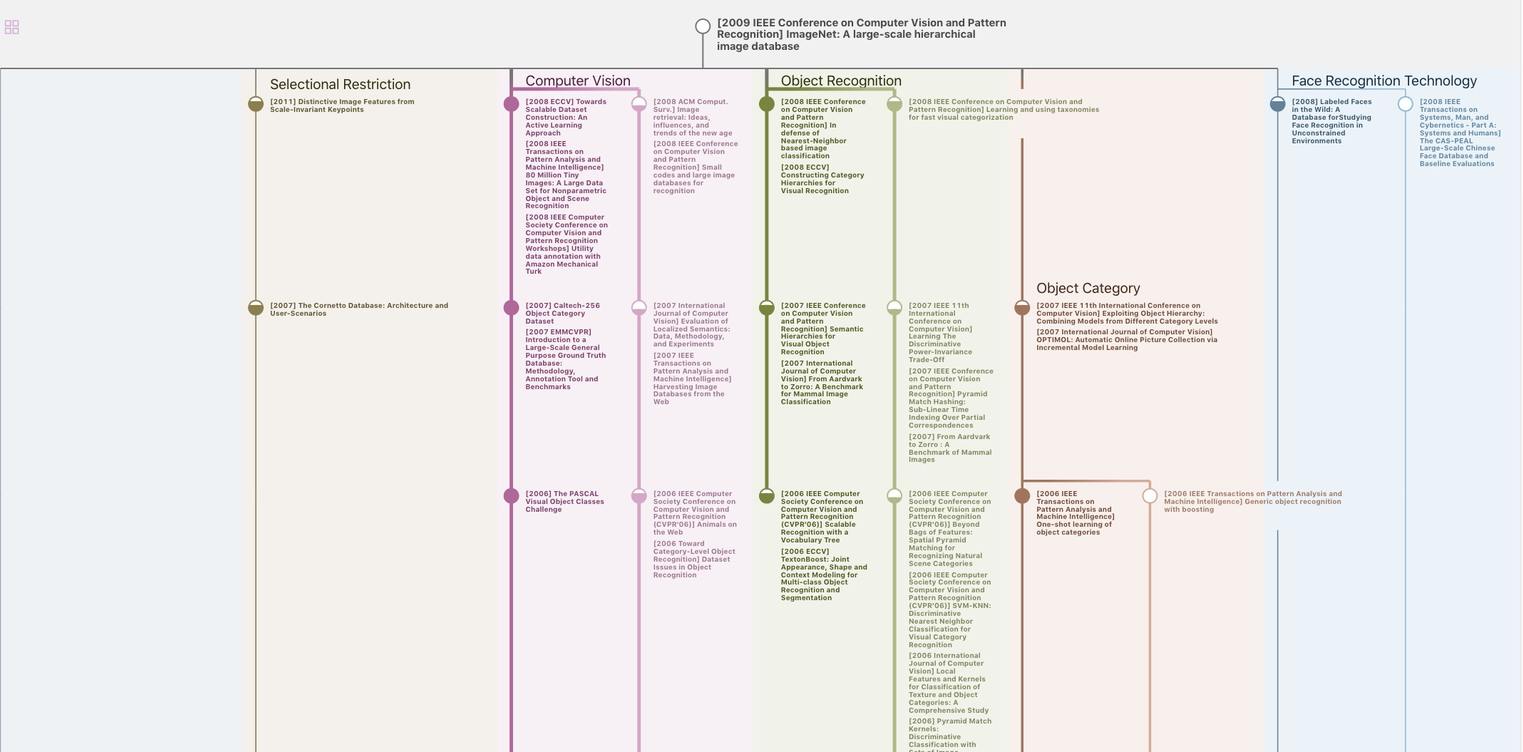
Generate MRT to find the research sequence of this paper
Chat Paper
Summary is being generated by the instructions you defined