Efficiently mining cohesion-based patterns and rules in event sequences
Data Mining and Knowledge Discovery(2019)
摘要
Discovering patterns in long event sequences is an important data mining task. Traditionally, research focused on frequency-based quality measures that allow algorithms to use the anti-monotonicity property to prune the search space and efficiently discover the most frequent patterns. In this work, we step away from such measures, and evaluate patterns using cohesion — a measure of how close to each other the items making up the pattern appear in the sequence on average. We tackle the fact that cohesion is not an anti-monotonic measure by developing an upper bound on cohesion in order to prune the search space. By doing so, we are able to efficiently unearth rare, but strongly cohesive, patterns that existing methods often fail to discover. Furthermore, having found the occurrences of cohesive itemsets in the input sequence, we use them to discover the representative sequential patterns and the dominant partially ordered episodes, without going through the computationally expensive candidate generation procedures typically associated with sequential pattern and episode mining. Experiments show that our method efficiently discovers important patterns that existing state-of-the-art methods fail to discover.
更多查看译文
关键词
Cohesive itemsets,Sequential patterns,Episodes,Association rules
AI 理解论文
溯源树
样例
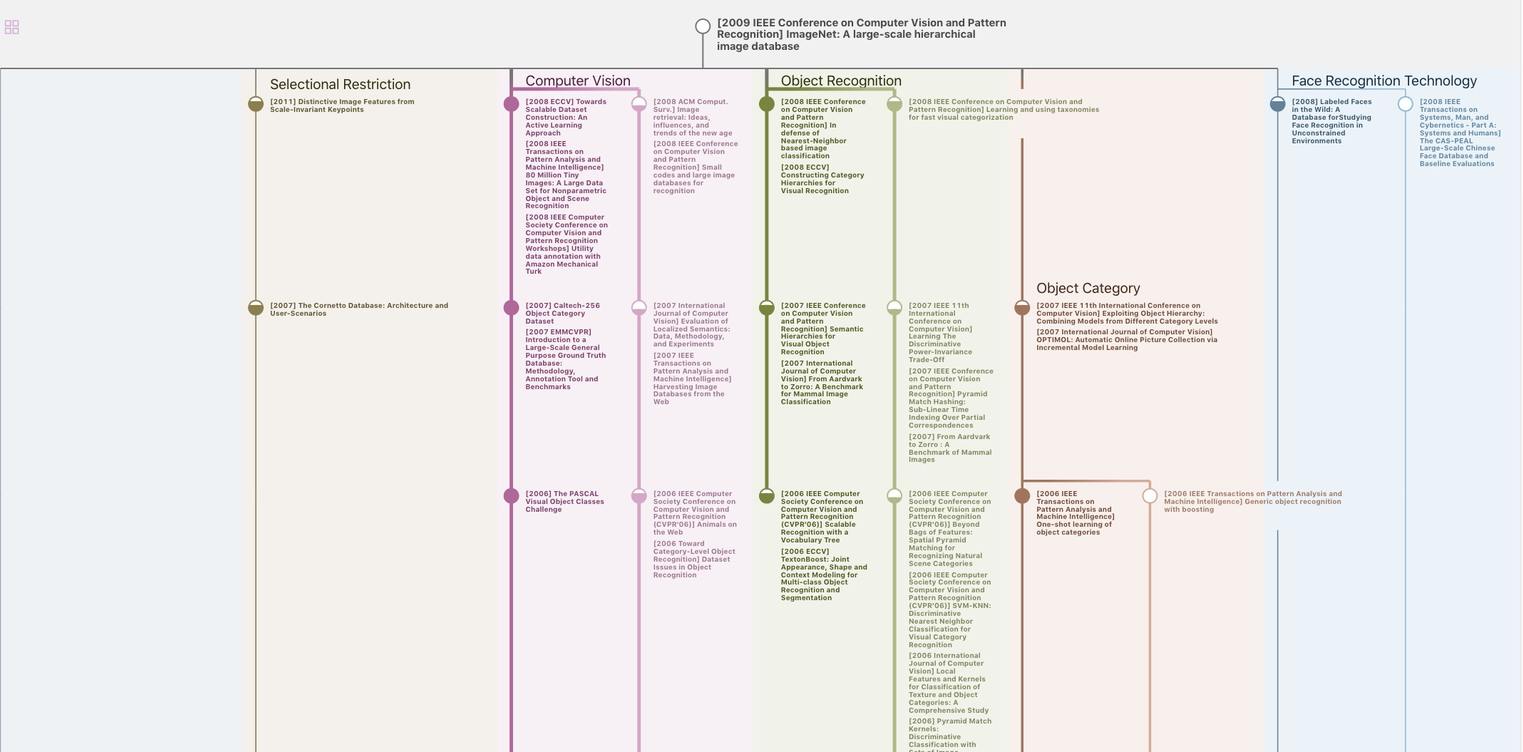
生成溯源树,研究论文发展脉络
Chat Paper
正在生成论文摘要