Deep neural network for unsteady aerodynamic and aeroelastic modeling across multiple Mach numbers
Nonlinear Dynamics(2019)
Abstract
Aerodynamic reduced-order model (ROM) is a useful tool to predict nonlinear unsteady aerodynamics with reasonable accuracy and very low computational cost. The efficacy of this method has been validated by many recent studies. However, the generalization capability of aerodynamic ROMs with respect to different flow conditions and different aeroelastic parameters should be further improved. In order to enhance the predicting capability of ROM for varying operating conditions, this paper presents an unsteady aerodynamic model based on long short-term memory (LSTM) network from deep learning theory for large training dataset and sampling space. This type of network has attractive potential in modeling temporal sequence data, which is well suited for capturing the time-delayed effects of unsteady aerodynamics. Different from traditional reduced-order models, the current model based on LSTM network does not require the selection of delay orders. The performance of the proposed model is evaluated by a NACA 64A010 airfoil pitching and plunging in the transonic flow across multiple Mach numbers. It is demonstrated that the model can accurately capture the dynamic characteristics of aerodynamic and aeroelastic systems for varying flow and structural parameters.
MoreTranslated text
Key words
Unsteady aerodynamic, Transonic flow, Long short-term memory network, Limit-cycle oscillation, Reduced-order model
AI Read Science
Must-Reading Tree
Example
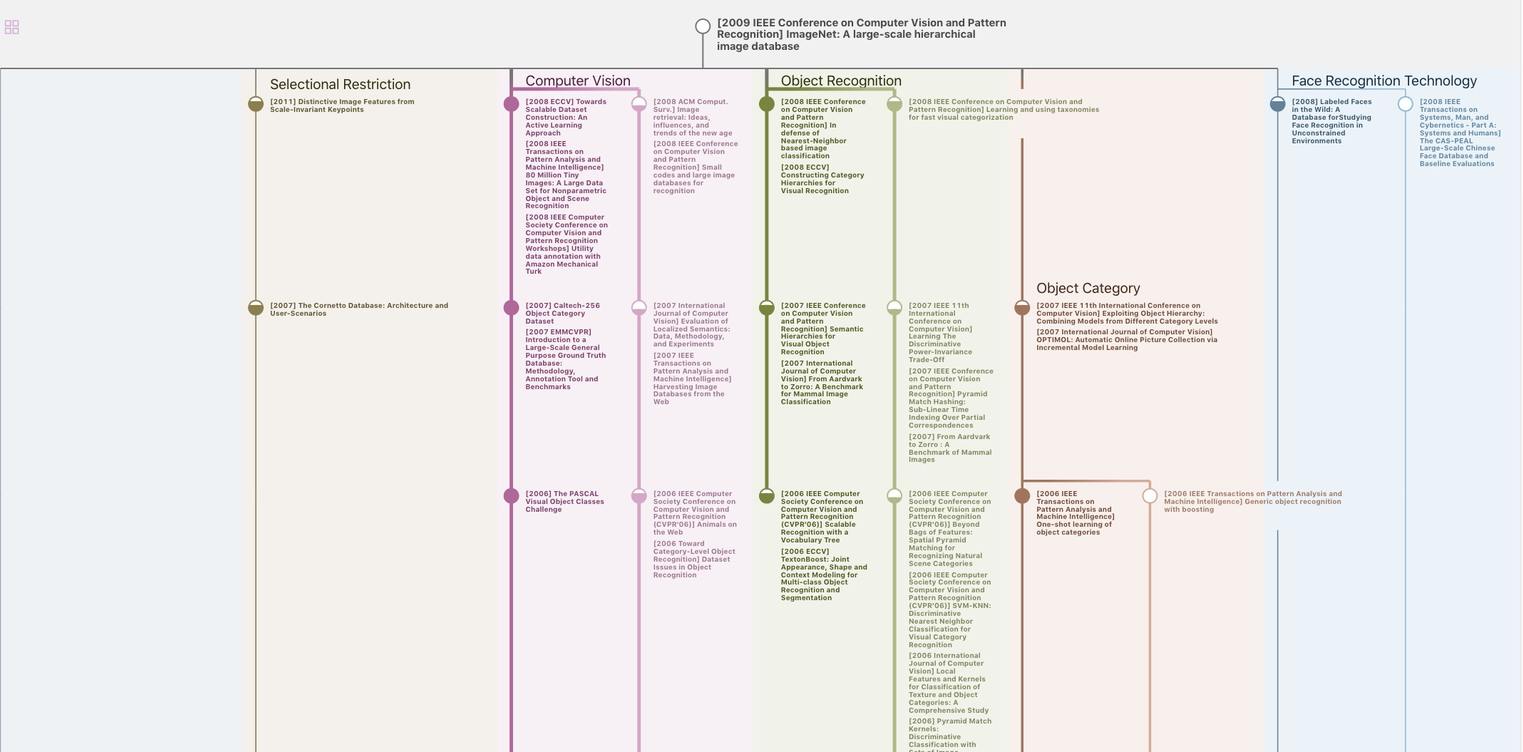
Generate MRT to find the research sequence of this paper
Chat Paper
Summary is being generated by the instructions you defined