Simultaneous Super-Resolution and Segmentation Using a Generative Adversarial Network: Application to Neonatal Brain MRI
2019 IEEE 16TH INTERNATIONAL SYMPOSIUM ON BIOMEDICAL IMAGING (ISBI 2019)(2019)
Abstract
Brest, France The analysis of clinical neonatal brain MRI remains challenging due to low anisotropic resolution of the data. In most pipelines, images are first re-sampled using interpolation or single image super-resolution techniques and then segmented using (semi-)automated approaches. Image reconstruction and segmentation are then performed separately. In this paper, we propose an end-to-end generative adversarial network for simultaneous high-resolution reconstruction and segmentation of brain MRI data. This joint approach is first assessed on the simulated low-resolution images of the high-resolution neonatal dHCP dataset. Then, the learned model is used to enhance and segment real clinical low-resolution images. Results demonstrate the potential of our proposed method with respect to practical medical applications.
MoreTranslated text
Key words
Super-resolution, segmentation, 3D generative adversarial networks, neonatal brain MRI
AI Read Science
Must-Reading Tree
Example
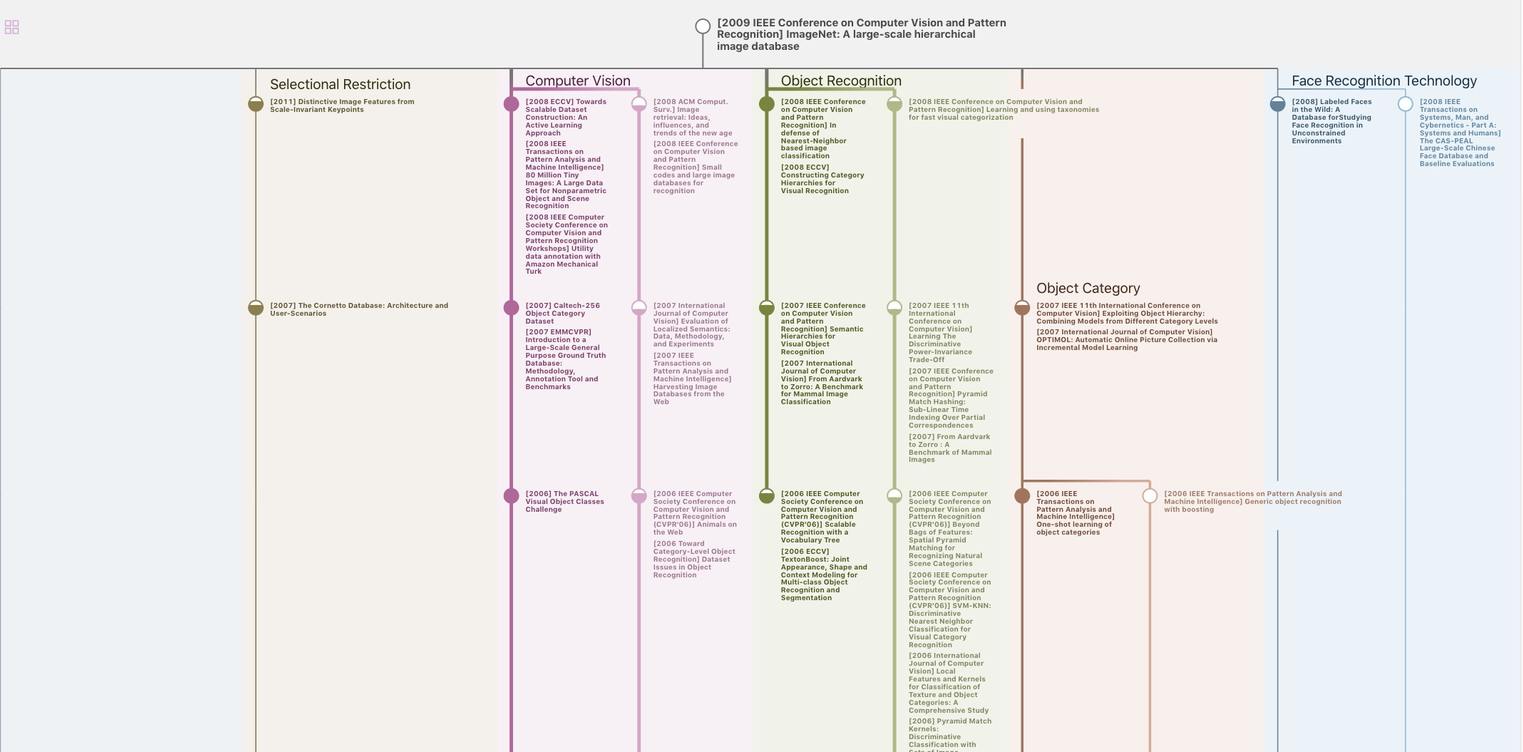
Generate MRT to find the research sequence of this paper
Chat Paper
Summary is being generated by the instructions you defined