Constrained non-negative matrix factorization algorithm combined with spatial homogeneous area analysis for hyperspectral unmixing
JOURNAL OF APPLIED REMOTE SENSING(2019)
摘要
The phenomenon of mixed pixels is serious in hyperspectral images because of its low spatial resolution; so, unmixing mixed pixels is very important for further application of hyperspectral images. The geometrical description in the characteristic space and the sparseness of the abundance matrix are the most important characteristics of the hyperspectral data, as well as the spatial construction information. Traditional algorithms always consider one characteristic and neglect the other. We propose the endmember initials determined by spatial homogeneous area analysis-minimum volume and L-1/2 regularization sparseness-constrained non-negative matrix factorization algorithm, considering all the above characteristics. In estimating the initial endmember matrix, spatial homogeneous area analysis is performed on endmember pixels calculated by vertex component analysis to achieve the goal of accelerating the algorithm's convergence. At the same time, we join the minimum volume endmember constraint and L-1/2 regularization abundance constraint of stronger sparseness as the final model of our algorithm. The experimental results of both the synthetic and the real data show that, compared with some classical algorithms, the proposed algorithm has a higher accuracy in hyperspectral unmixing. (C) 2019 Society of Photo-Optical Instrumentation Engineers (SPIE)
更多查看译文
关键词
hyperspectral unmixing,non-negative matrix factorization,spatial homogeneous area analysis,minimum volume,L-1/2 regularization
AI 理解论文
溯源树
样例
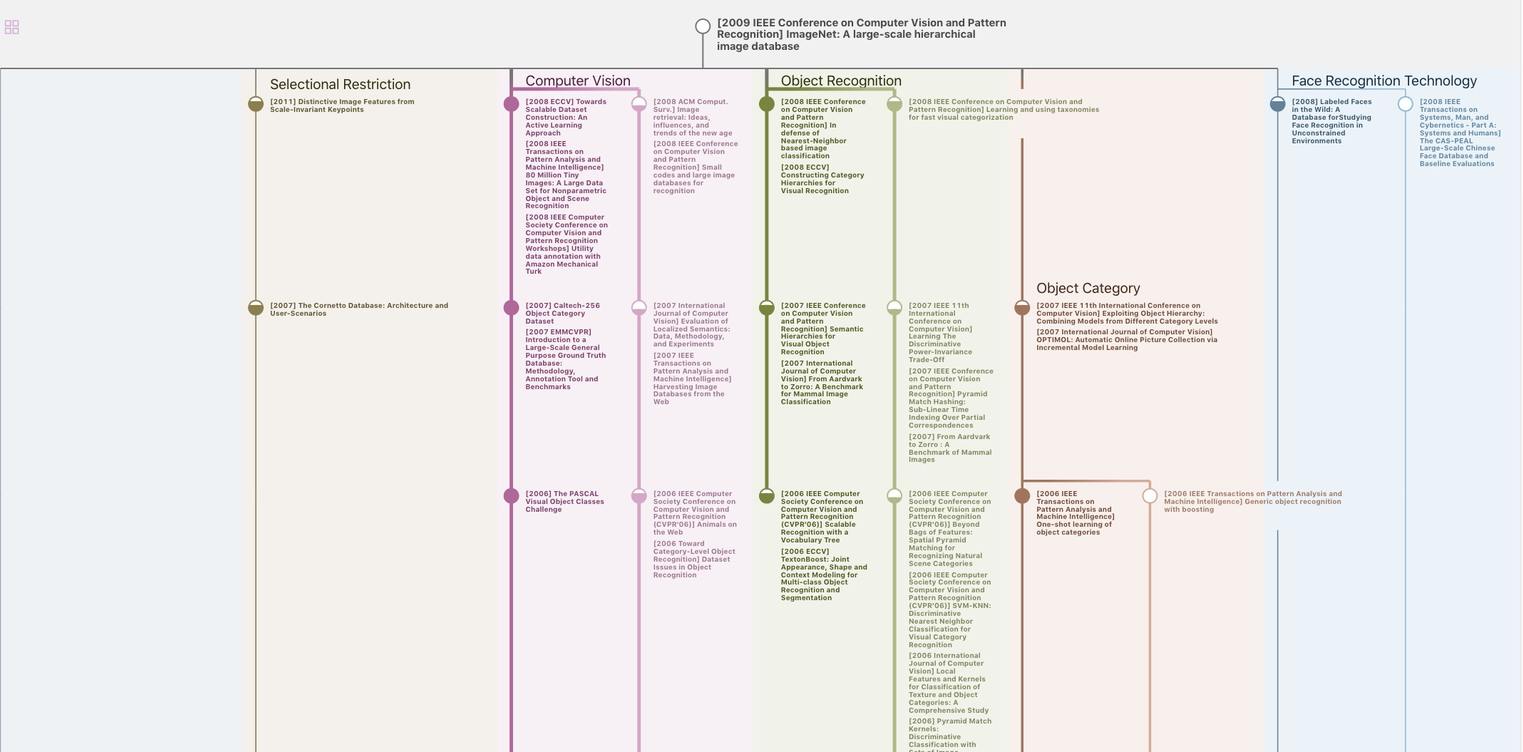
生成溯源树,研究论文发展脉络
Chat Paper
正在生成论文摘要