An analysis of spectral variability in hyperspectral imagery: a case study of stressed oil palm detection in Colombia
INTERNATIONAL JOURNAL OF REMOTE SENSING(2019)
摘要
Hyperspectral images have acquired an increasing interest in the scientific and civil community to detect or classify materials by their spectral signature in remote sensing applications. However, spectral signatures can vary because of lighting or atmospheric conditions. This phenomenon is known as spectral variability, and ignoring this variability is an important source of error in the analysis of hyperspectral images. Therefore, this work focuses on analysing different causes and effects of the spectral variability in hyperspectral images, by studying the latest advances in this area, including approaches that do not address the phenomenon and methods that address spectral variability. Experiments are executed with five different approaches to compare their accuracy in the estimation of abundances in synthetic data generated with spectral variability. Specifically, a case study is developed on hyperspectral data of oil palm crops in Colombia, which exhibit high spectral variability. The results of identification of stressed oil palms are compared by mapping the abundances of three classes (diseased oil palm, healthy oil palm, and background), evaluating the performance of the different approaches in terms of the root mean squared error (RMSE), reconstruction error (RE), and spectral angle mapping (SAM). Results show that the coordinate descent algorithm-mismodelling effects (CDA-ME) present the best performance, outperforming in up to 70% the other approaches with and without spectral variability since it can jointly model changes of illumination, effects of variability and outlier values present in the hyperspectral images.
更多查看译文
AI 理解论文
溯源树
样例
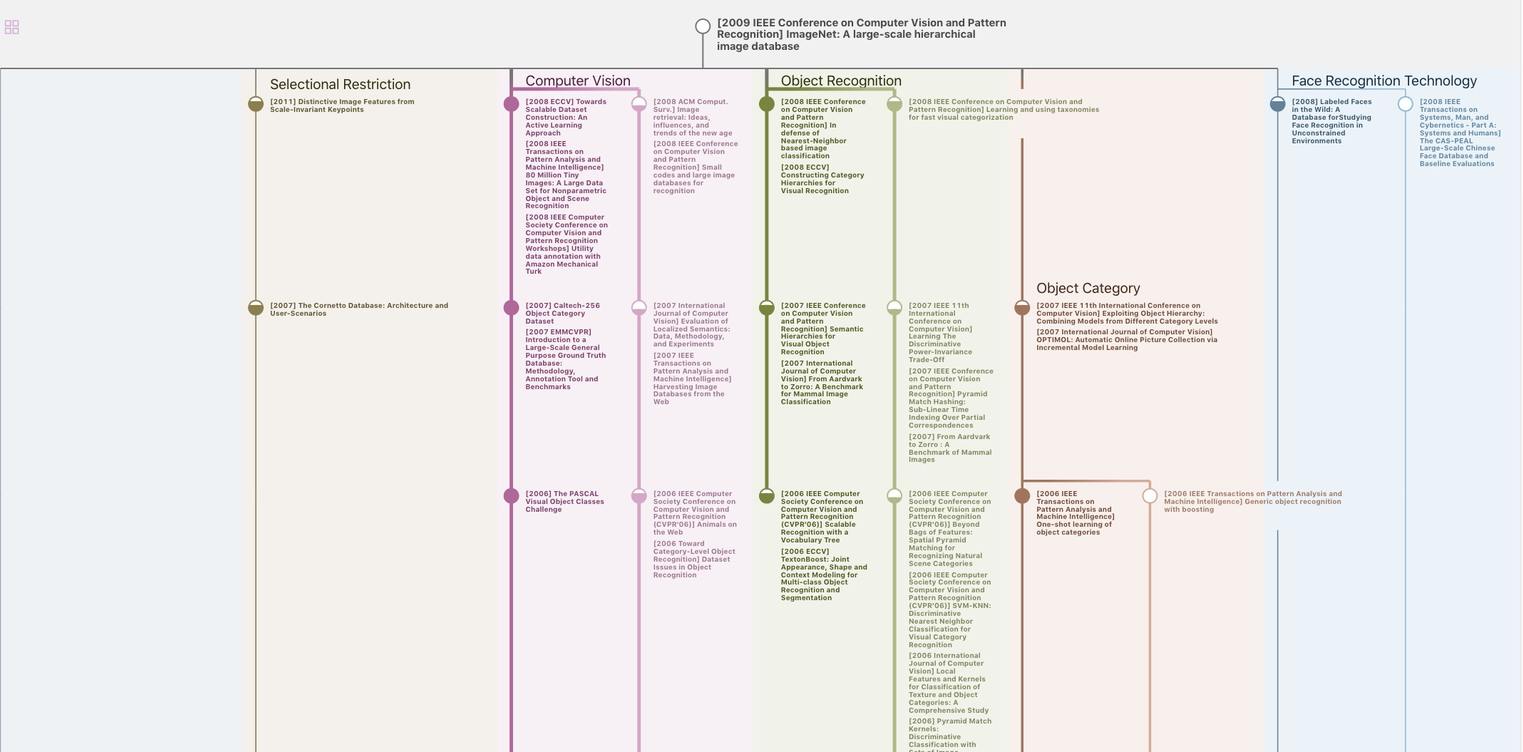
生成溯源树,研究论文发展脉络
Chat Paper
正在生成论文摘要