Cascaded Iterative Training Model and Parallel Multi-Classifiers for Visual Object Tracking
IEEE ACCESS(2019)
摘要
Because of the superiority in distinguishing the target from the background by learning mechanism, discriminative classifier attracts a great deal of attention in target detection and tracking systems. Generally, a discriminative classifier is learned from the initial image patch. However, the other algorithms, using only one image frame inhibit the performance of the classifier. A novel training strategy of cascade iteration, which is based on the multiple image frames, is used to initialize the discriminative classifier for the purpose of weakening the initialization limitation and improving the algorithms' adaptability and robustness. Furthermore, to cope with the changes in natural images, online learning is usually adopted to enhance the performance of classifiers, but it easily leads to tracking failures, especially in the case of full occlusion. In order to attenuate the infiuence of full occlusion, a multi-classifiers parallel tracking algorithm is proposed by designing the multi-classifiers and inducing extra constraints. The experiments are performed on the visual tracking benchmark, and the proposed algorithm is more robust while the target is fully occluded. The simulation results show that the proposed algorithm exhibits better performance compared to the state-of-the-art trackers, especially with KCF, TLD, and STRUCK.
更多查看译文
关键词
Visual tracking,cascade iteration,multiple classifiers,parallel tracking,correlation filters
AI 理解论文
溯源树
样例
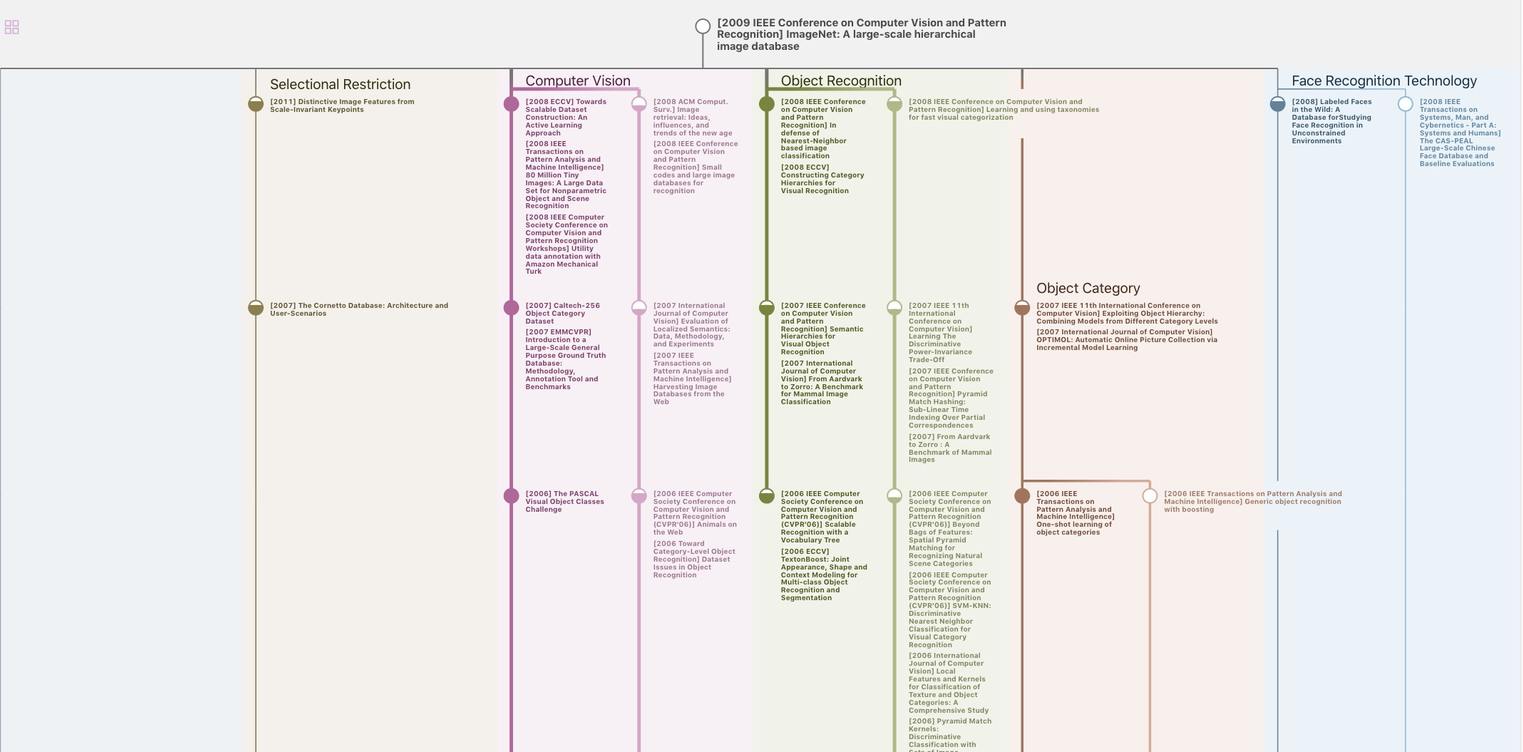
生成溯源树,研究论文发展脉络
Chat Paper
正在生成论文摘要