Segmentation of dust storm areas on Mars images using principal component analysis and neural network
Progress in Earth and Planetary Science(2019)
Abstract
We present a method for automated segmentation of dust storm areas on Mars images observed by an orbiter. We divide them into small patches. Normal basis vectors are obtained from the many small patches by principal component analysis. We train a classifier using coefficients of these basis vectors as feature vectors. All patches in test images are categorized into one of the dust storm, cloud, and surface classes by the classifier. Each pixel may be included in several dust storm patches. The pixel is classified as a dust storm or the other classes based on the number of dust storm patches that include the pixel. We evaluate the segmentation method by the receiver operator characteristic curve and the area under the curve (AUC). AUC for dust storm is 0.947–0.978 if dust storm areas determined by our visual inspection are assumed to be ground truth. Precision, recall, and F -measure for dust storm are 0.88, 0.84, and 0.86, respectively, if we remove false negative pixels efficiently and maintain the size of true positive dust storms using two different threshold values. The tuning parameters of the classifier used in this study are determined so that the accuracy for dust storm is maximized. We can also tune the classifier for cloud segmentation by changing the parameters.
MoreTranslated text
Key words
Mars, Dust storm, Segmentation, Machine learning, Principal component analysis
AI Read Science
Must-Reading Tree
Example
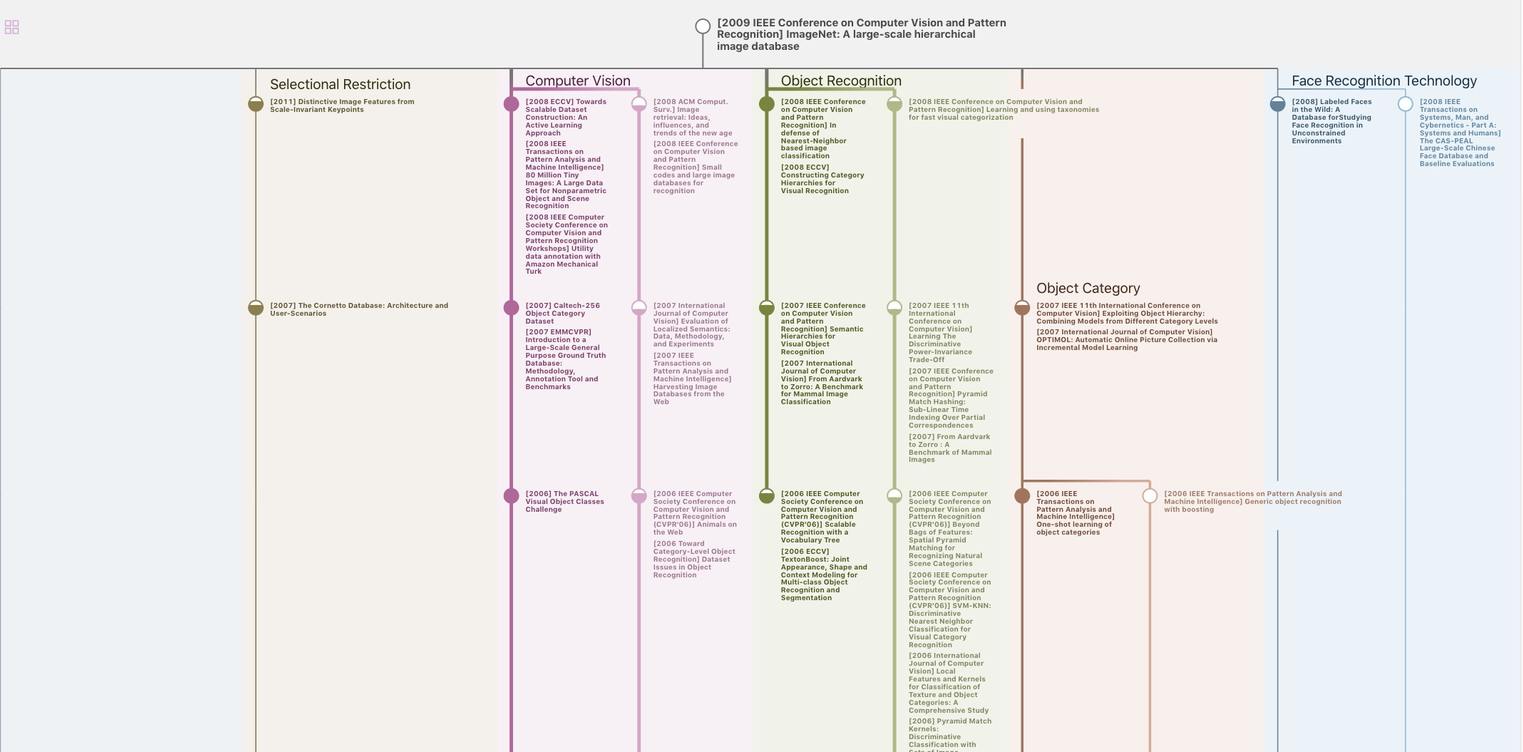
Generate MRT to find the research sequence of this paper
Chat Paper
Summary is being generated by the instructions you defined