Concept Drift Based Multi-dimensional Data Streams Sampling Method.
pacific-asia conference on knowledge discovery and data mining(2019)
Abstract
A summary can immensely reduce the time and space complexity of an algorithm. This concept is considered a research hotspot in the field of data stream mining Data streams are characterized as having continuous data arrival, rapid speed, large scale, and cannot be completely stored in memory simultaneously. A summary is often formed in the memory to approximate the database query or data mining task. A sampling technique is a commonly used method for constructing data stream summaries. Traditional simple random sampling algorithms do not consider the conceptual drift of data distributions that change over time. Therefore, a challenging task is sampling the summary of the data distribution in multi-dimensional data streams of a concept drift. This study proposes a sampling algorithm that ensures the consistency of the data distribution with the data streams of the concept drift. First, probability statistics is used on the data stream cells in the reference window to obtain data distribution. A probability sampling is performed on the basis of this distribution. Second, the sliding window is used to continuously detect whether the data distribution has changed. If the data distribution does not change, then the original sampling data are maintained. Otherwise, the data distribution in the statistical window is restated to form a new sampling probability. The proposed algorithm ensures that the data distribution in the data profile is continually consistent with the population distribution. We compare our algorithm with the state-of-the-art algorithms on synthetic and real data sets. Experimental results demonstrate the effectiveness of our algorithm.
MoreTranslated text
Key words
Data stream clustering, Sampling, Summary
AI Read Science
Must-Reading Tree
Example
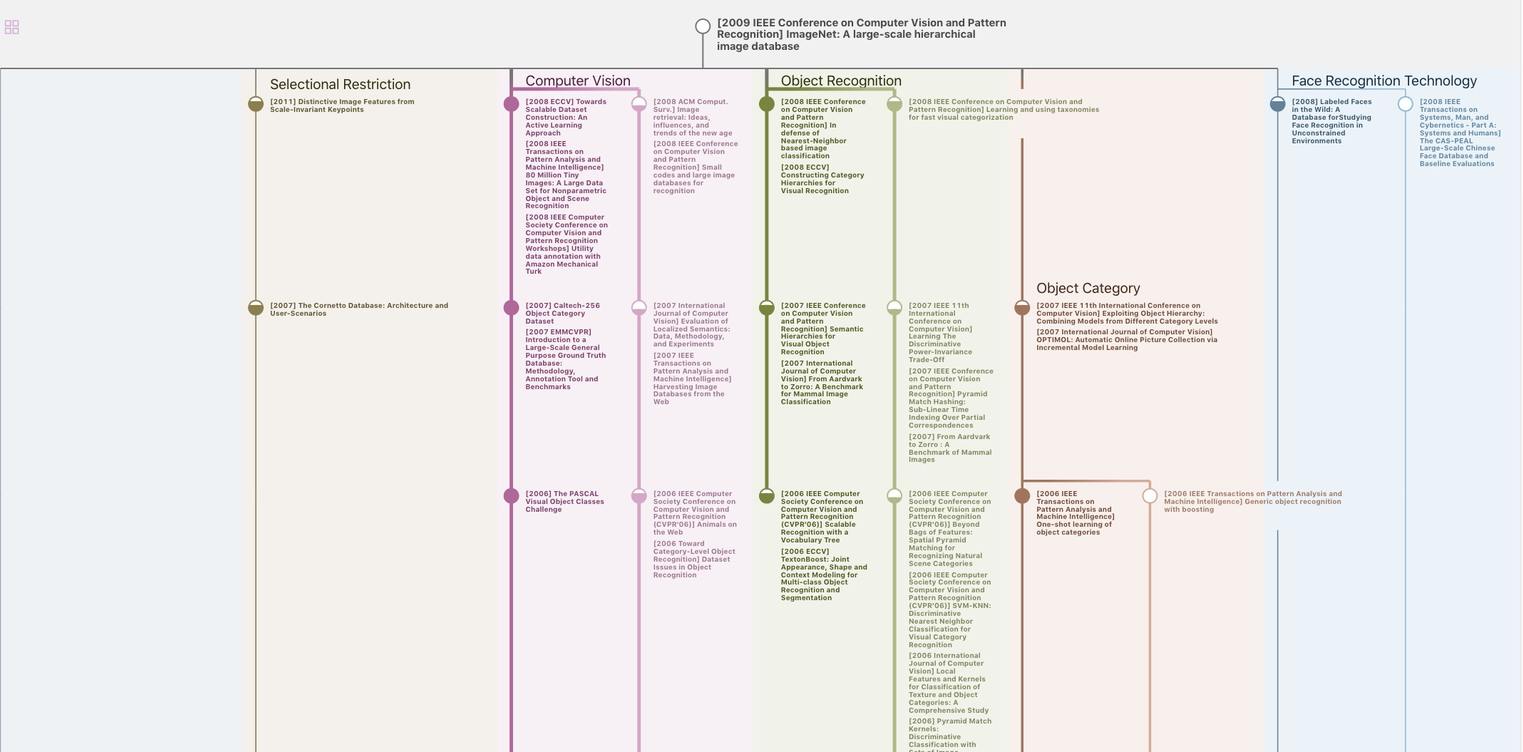
Generate MRT to find the research sequence of this paper
Chat Paper
Summary is being generated by the instructions you defined