On-chip epilepsy detection: Where machine learning meets patient-specific healthcare
2017 International SoC Design Conference (ISOCC)(2017)
摘要
Detecting epileptic seizure from EEG/iEEG is challenging due to each patient's onset pattern being very different from each other. To detect such onsets (in real time), the hardware must learn each patient's ictal pattern from the EEG/iEEG traces. This paper describes feature extraction and usage of Support Vector Machine (SVM)-based classifiers to achieve a patient-specific seizure detection System-on-Chip (SoC). Linear SVM (LSVM), Non-Linear SVM (NLSVM), and the Dual Detector Architecture (D
2
A)-SVM are described and compared. We show that when there are a limited number of training sets, the D
2
A-SVM classifier performs well while minimizing the hardware cost. The SoC is implemented and verified.
更多查看译文
关键词
Electroencephalography (EEG),epilepsy,invasive EEG (iEEG),machine learning,seizure,support-vector machine (SVM)
AI 理解论文
溯源树
样例
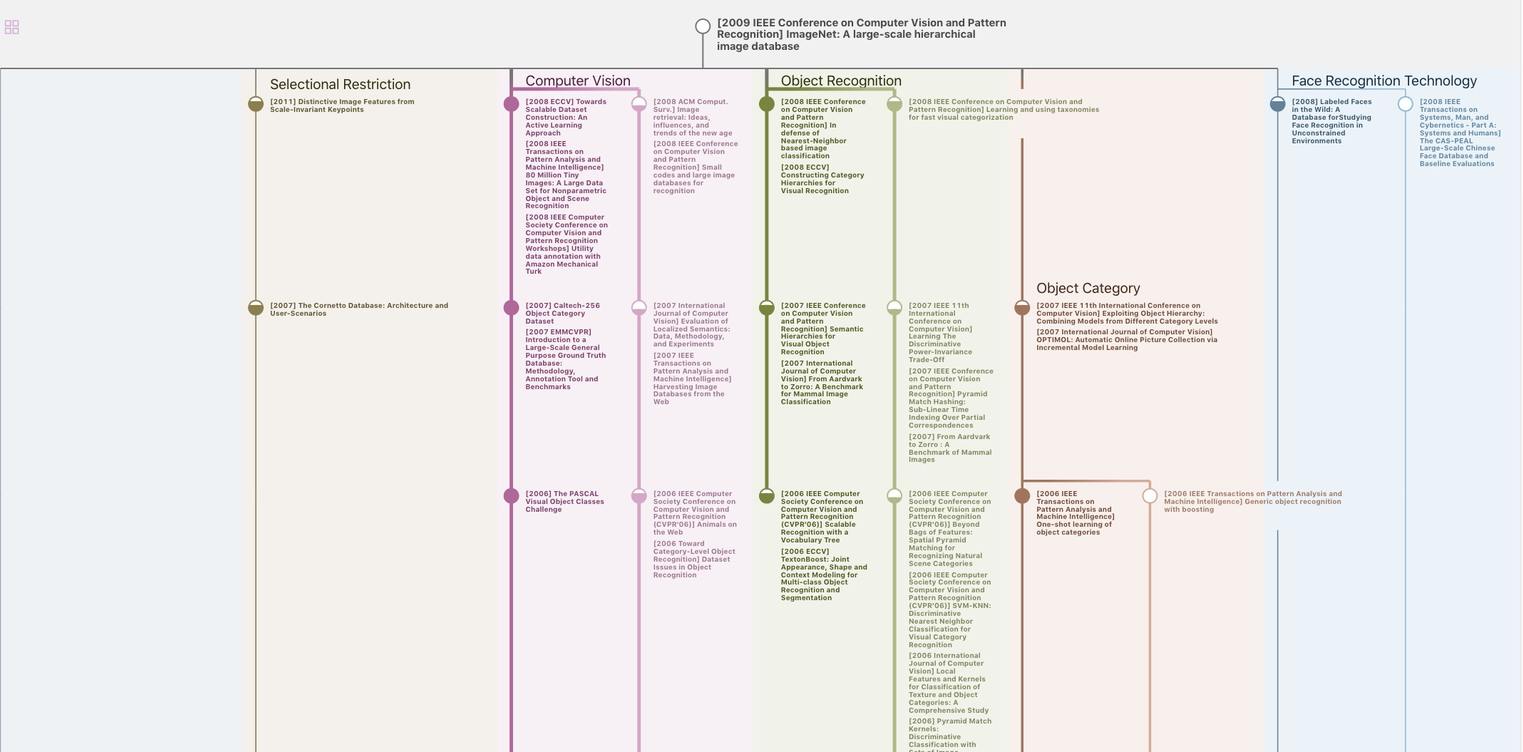
生成溯源树,研究论文发展脉络
Chat Paper
正在生成论文摘要