Gender-Specific Multi-Task Micro-Expression Recognition Using Pyramid CGBP-TOP Feature
CMES-COMPUTER MODELING IN ENGINEERING & SCIENCES(2019)
摘要
Micro-expression recognition has attracted growing research interests in the field of compute vision. However, micro-expression usually lasts a few seconds, thus it is difficult to detect. This paper presents a new framework to recognize micro-expression using pyramid histogram of Centralized Gabor Binary Pattern from Three Orthogonal Panels (CGBP-TOP) which is an extension of Local Gabor Binary Pattern from Three Orthogonal Panels feature. CGBP-TOP performs spatial and temporal analysis to capture the local facial characteristics of micro-expression image sequences. In order to keep more local information of the face, CGBP-TOP is extracted based on pyramid sub-regions of the micro-expression video frame. The combination of CGBP-TOP and spatial pyramid can represent well and truly the facial movements of the micro-expression image sequences. However, the dimension of our pyramid CGBP-TOP tends to be very high, which may lead to high data redundancy problem. In addition, it is clear that people of different genders usually have different ways of micro-expression. Therefore, in this paper, in order to select the relevant features of micro-expression, the gender-specific sparse multi-task learning method with adaptive regularization term is adopted to learn a compact subset of pyramid CGBP-TOP feature for micro-expression classification of different sexes. Finally, extensive experiments on widely used CASME II and SMIC databases demonstrate that our method can efficiently extract micro-expression motion features in the micro-expression video clip. Moreover, our proposed approach achieves comparable results with the state-of-the-art methods.
更多查看译文
关键词
Micro-expression recognition,feature extraction,spatial pyramid,multi-task learning,regularization
AI 理解论文
溯源树
样例
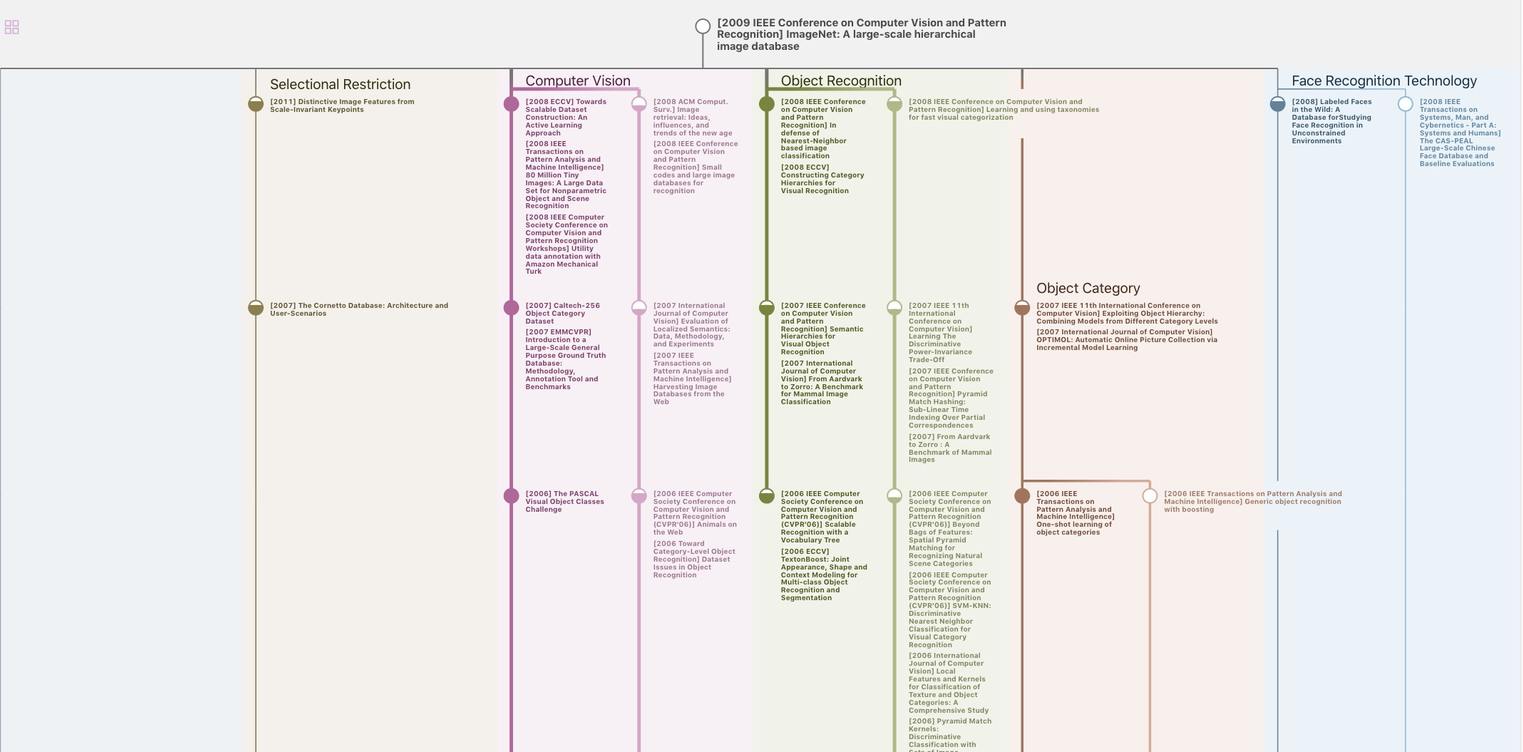
生成溯源树,研究论文发展脉络
Chat Paper
正在生成论文摘要