Nonnegative Tensor-Based Linear Dynamical Systems for Recognizing Human Action from 3D Skeletons
MATHEMATICAL PROBLEMS IN ENGINEERING(2019)
摘要
Recently, skeleton-based action recognition has become a very important topic in the field of computer vision. It is a challenging task to accurately build a human action model and precisely distinguish similar human actions. In this paper, an action (skeleton sequence) is represented as a third-order nonnegative tensor time series to capture the original spatiotemporal information of the action. As a linear dynamical system (LDS) is an efficient tool for encoding the spatiotemporal data in various disciplines, this paper proposes a nonnegative tensor-based LDS (nLDS) to model the third-order nonnegative tensor time series. Nonnegative Tucker decomposition (NTD) is utilized to estimate the parameters of the nLDS model. These parameters are used to build extended observability sequence O-infinity(T) for the action, which implies that O-infinity(T) can be considered as the feature descriptor of the action. To avoid the limitations introduced by approximating O-infinity(T) with a finite-order matrix, we represent an action as a point on infinite Grassmann manifold comprising the orthonormalized extended observability sequences. The classification task can be performed by dictionary learning and sparse coding on the infinite Grassmann manifold. The experimental results on the MSR-Action3D, UTKinect-Action, and G3D-Gaming datasets demonstrate that the proposed approach achieves a better performance in comparison with the state-of-the-art methods.
更多查看译文
AI 理解论文
溯源树
样例
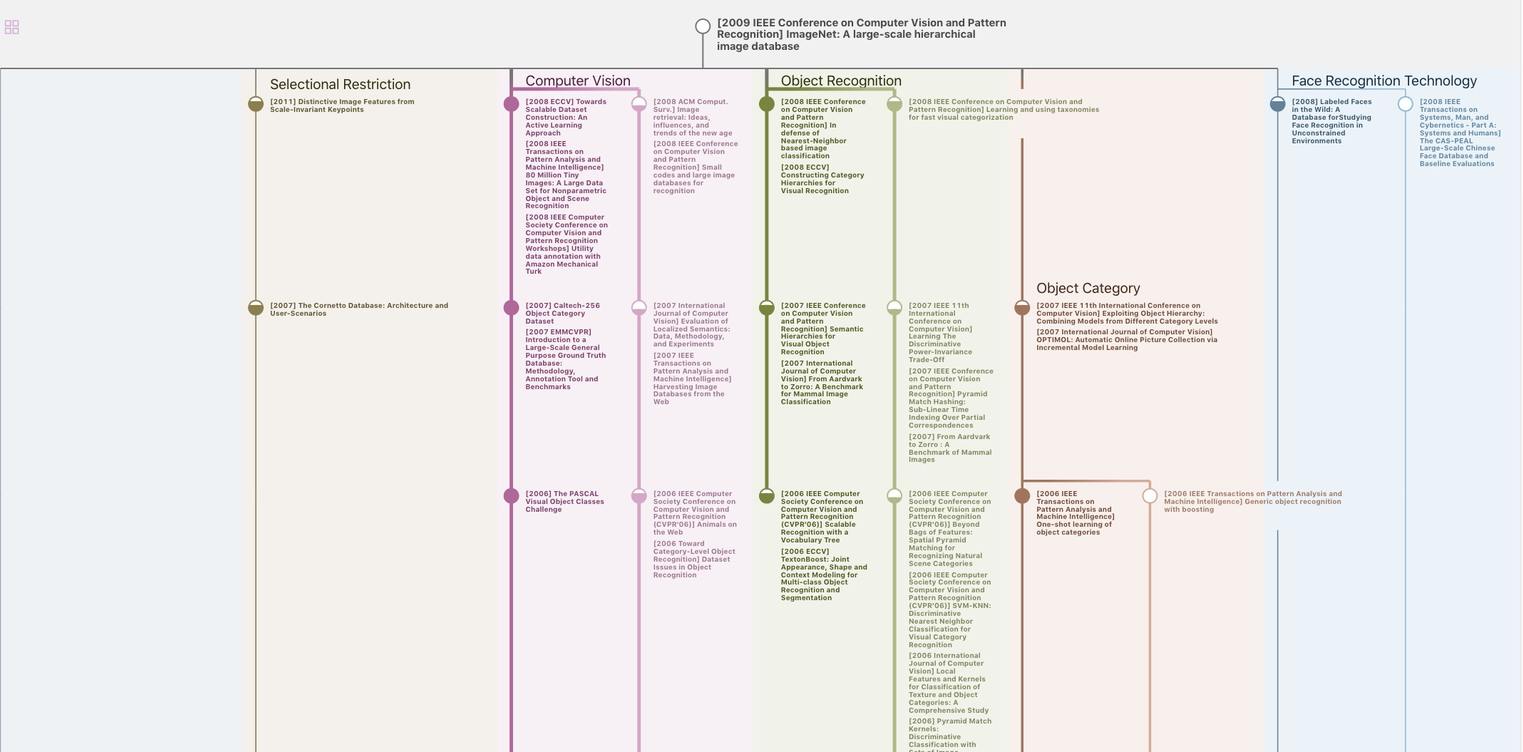
生成溯源树,研究论文发展脉络
Chat Paper
正在生成论文摘要