Functional logistic discrimination with sparse PCA and its application to the structural MRI
Behaviormetrika(2019)
摘要
We propose a functional classification method with high-dimensional image predictors using a combination of logistic discrimination and basis expansions with sparse principal component analysis (PCA). Our model is an extension of the existing functional generalized linear models with image predictors using functional principal component regression to L1-regularized principal components. This extension enables us to create a more flexible prognostic region that does not depend on the shape of basis functions. Monte Carlo simulations were conducted to examine the method’s efficiency when compared with several possible classification techniques. Our method was shown to be the best in terms of both sensitivity and specificity for detecting the shape of interests and classifying groups. In addition, our model was applied to data on Alzheimer’s disease. Our model detected the prognostic brain region and was used to classify early-stage Alzheimer patients efficiently, based on three-dimensional structural magnetic resonance imaging (sMRI).
更多查看译文
关键词
Functional data analysis, Regularized logistic classification, Sparse PCA, Radial basis expansions, 62H30, 62H25, 62P10
AI 理解论文
溯源树
样例
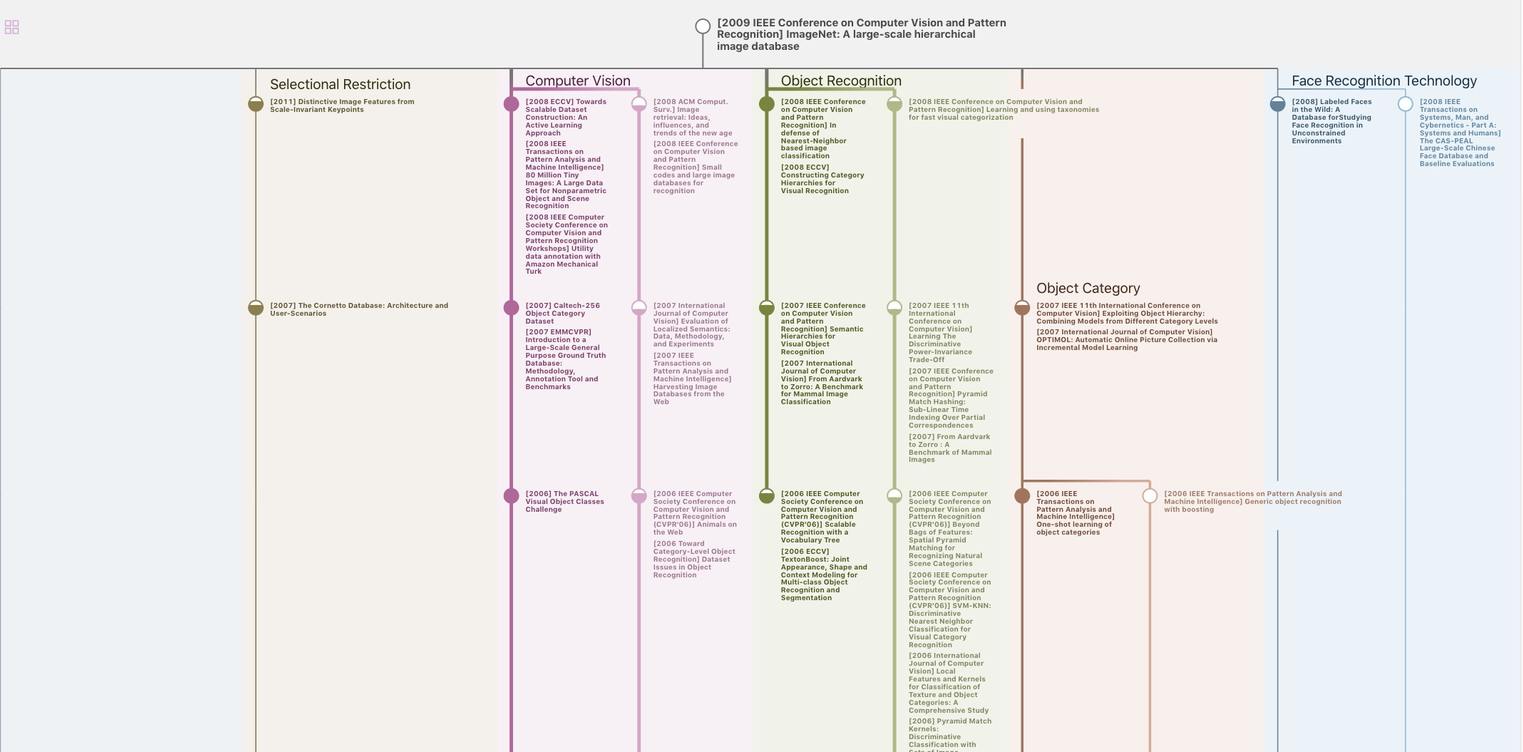
生成溯源树,研究论文发展脉络
Chat Paper
正在生成论文摘要