Continuous monitoring of land disturbance based on Landsat time series
Remote Sensing of Environment(2020)
摘要
We developed a new algorithm for COntinuous monitoring of Land Disturbance (COLD) using Landsat time series. COLD can detect many kinds of land disturbance continuously as new images are collected and provide historical land disturbance maps retrospectively. To better detect land disturbance, we tested different kinds of input data and explored many time series analysis techniques. We have several major observations as follows. First, time series of surface reflectance provides much better detection results than time series of Top-Of-Atmosphere (TOA) reflectance, and with some adjustments to the temporal density, time series from Landsat Analysis Ready Data (ARD) is better than it is from the same Landsat scene. Second, the combined use of spectral bands is always better than using a single spectral band or index, and if all the essential spectral bands have been employed, the inclusion of other indices does not further improve the algorithm performance. Third, the remaining outliers in the time series can be removed based on their deviation from model predicted values based on probability-based thresholds derived from normal or chi-squared distributions. Fourth, model initialization is pivotal for monitoring land disturbance, and a good initialization stability test can influence algorithm performance substantially. Fifth, time series model estimation with eight coefficients model, updated for every single observation, based on all available clear observations achieves the best result. Sixth, a change probability of 0.99 (chi-squared distribution) with six consecutive anomaly observations and a mean included angle < 45° to confirm a change provide the best results, and the combined use of temporally-adjusted Root Mean Square Error (RMSE) and minimum RMSE is recommended. Finally, spectral changes (or “breaks”) contributed from vegetation regrowth should be excluded from land disturbance maps. The COLD algorithm was developed and calibrated based on all these lessons learned above. The accuracy assessment shows that COLD results were accurate for detecting land disturbance, with an omission error of 27% and a commission error of 28%.
更多查看译文
关键词
COLD,Land disturbance,Change detection,Time series,Landsat,Near real-time
AI 理解论文
溯源树
样例
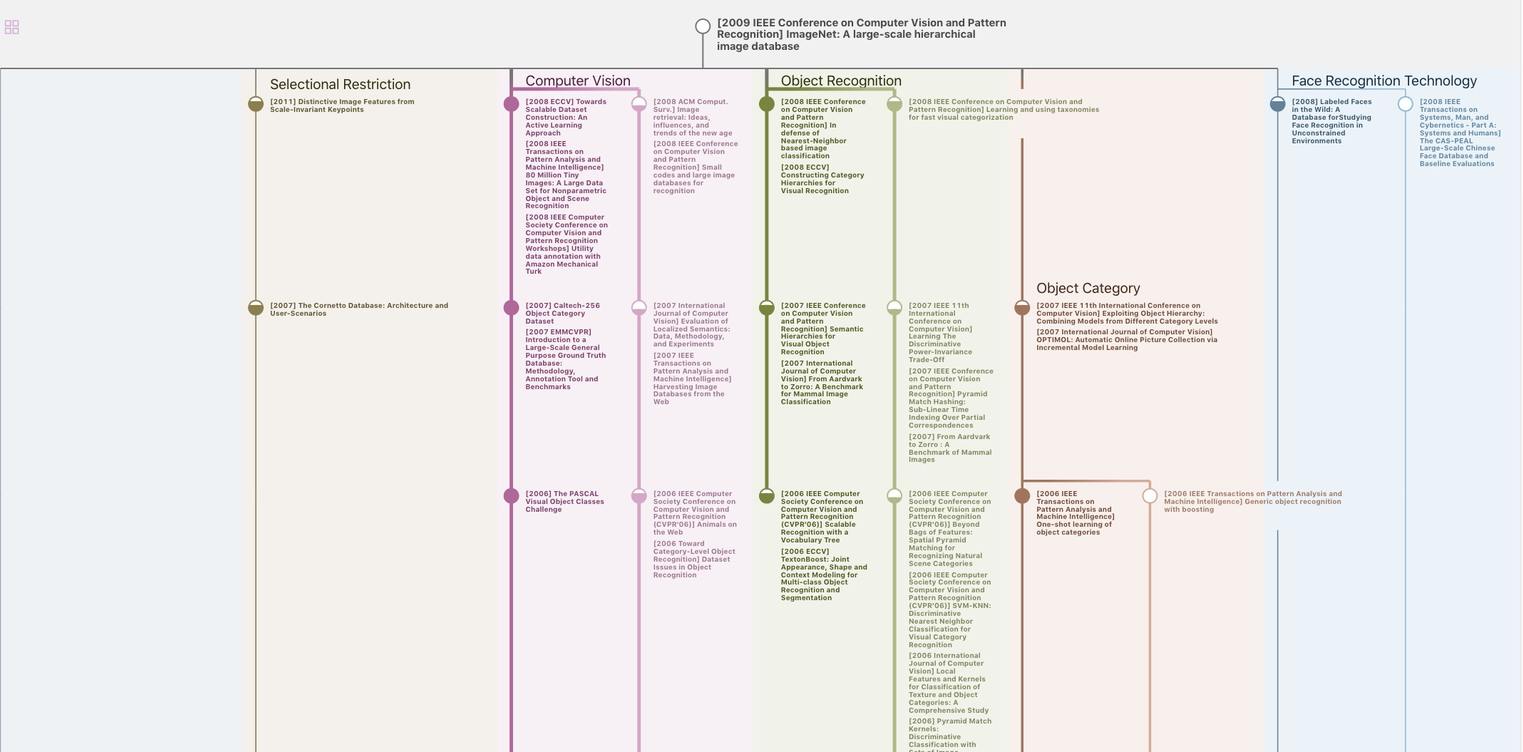
生成溯源树,研究论文发展脉络
Chat Paper
正在生成论文摘要