Thompson Sampling Algorithms for Cascading Bandits
22ND INTERNATIONAL CONFERENCE ON ARTIFICIAL INTELLIGENCE AND STATISTICS, VOL 89(2019)
摘要
Motivated by efficient optimization for online recommender systems, we revisit the cascading bandit model proposed by Kveton et al.(2015). While Thompson sampling (TS) algorithms have been shown to be empirically superior to Upper Confidence Bound (UCB) algorithms for cascading bandits, theoretical guarantees are only known for the latter, not the former. In this paper, we close the gap by designing and analyzing a TS algorithm, TS-Cascade, that achieves the state-of-the-art regret bound for cascading bandits. In complement, we derive a nearly matching regret lower bound, with information-theoretic techniques and judiciously constructed cascading bandit instances. Finally, we consider a linear generalization of the cascading bandit model, which allows efficient learning in large cascading bandit problem instances. We introduce a TS algorithm, which enjoys a regret bound that depends on the dimension of the linear model but not the number of items. Our paper establishes the first theoretical guarantees on TS algorithms for stochastic combinatorial bandit problem model with partial feedback. Numerical experiments demonstrate the superiority of our TS algorithms compared to existing UCB alogrithms.
更多查看译文
关键词
Multi-armed bandits,Thompson sampling,Cascading bandits,Linear bandits,Regret minimization
AI 理解论文
溯源树
样例
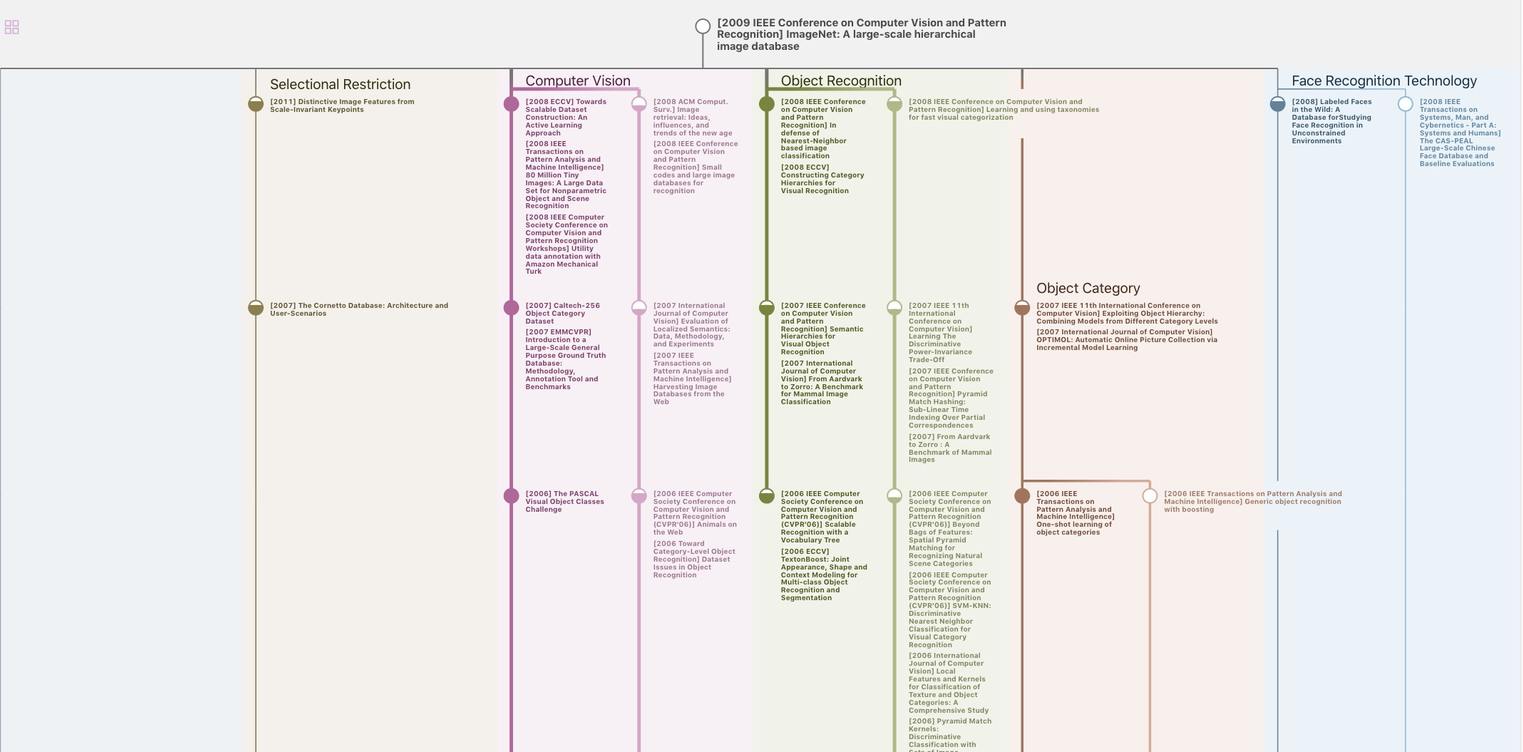
生成溯源树,研究论文发展脉络
Chat Paper
正在生成论文摘要