A Generalized Locally Linear Factorization Machine with Supervised Variational Encoding
IEEE Transactions on Knowledge and Data Engineering(2020)
摘要
Factorization Machines (FMs) learn weights for feature interactions, and achieve great success in many data mining tasks. Recently, Locally Linear Factorization Machines (LLFMs) have been proposed to capture the underlying structures of data for better performance. However, one obvious drawback of LLFM is that the local coding is only operated in the original feature space, which limits the model to be applied to high-dimensional and sparse data. In this work, we present a generalized LLFM (GLLFM) which overcomes this limitation by modeling the local coding procedure in a latent space. Moreover, a novel Supervised Variational Encoding (SVE) technique is proposed such that the distance can effectively describe the similarity between data points. Specifically, the proposed GLLFM-SVE trains several local FMs in the original space to model the higher order feature interactions effectively, where each FM associates to an anchor point in the latent space induced by SVE. The prediction for a data point is computed by a weighted sum of several local FMs, where the weights are determined by local coding coordinates with anchor points. Actually, GLLFM-SVE is quite flexible and other Neural Network (NN) based FMs can be easily embedded into this framework. Experimental results show that GLLFM-SVE significantly improves the performance of LLFM. By using NN-based FMs as local predictors, our model outperforms all the state-of-the-art methods on large-scale real-world benchmarks with similar number of parameters and comparable training time.
更多查看译文
关键词
Frequency modulation,Encoding,Artificial neural networks,Euclidean distance,Optimization,Computational modeling
AI 理解论文
溯源树
样例
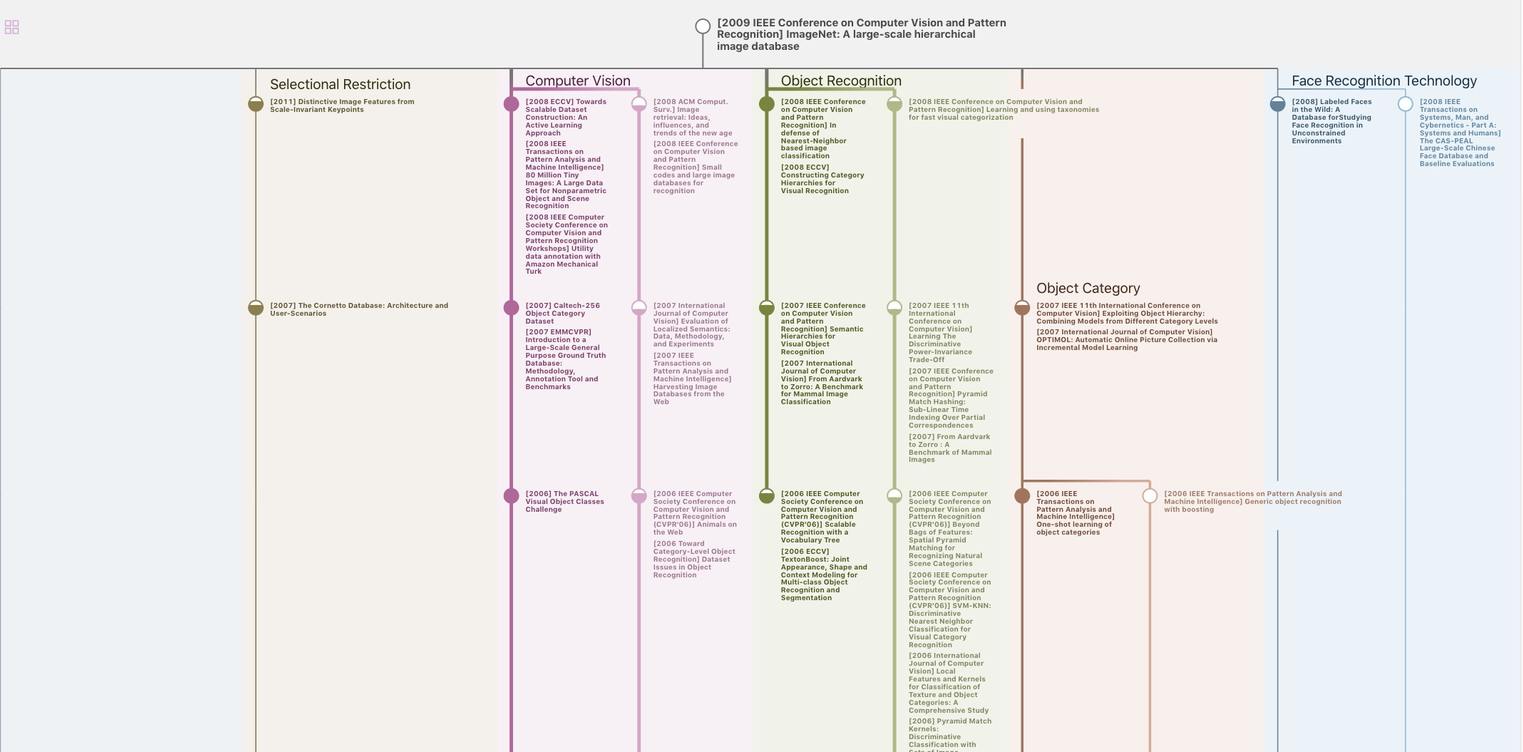
生成溯源树,研究论文发展脉络
Chat Paper
正在生成论文摘要