End-to-End Trained Sparse Coding Network with Spatial Pyramid Pooling for Image Classification
Neural Processing Letters(2019)
摘要
Spatial pyramid matching using sparse coding (ScSPM) has become an efficient method and a benchmark in image classification. However, since it is unsupervised, the trained dictionary may be suboptimal. To further improve classification accuracy, in this paper we propose a sparse coding network with spatial pyramid pooling based on the end-to-end deep learning approach. In our new system, the minimization problem in sparse coding can be modeled as a feed-forward neural network and image features can be extracted by the deep convolutional network. By minimizing the final classifier loss using the end-to-end deep learning method, the sparse coding network can be trained in a supervised way. Our proposed model is tested on three image databases and in terms of classification accuracy, it significantly outperforms ScSPM. Compared with other image classification approaches based on deep learning, it can also achieve a noticeable improvement.
更多查看译文
关键词
Sparse coding network, Spatial pyramid pooling, Image classification, Deep convolutional network
AI 理解论文
溯源树
样例
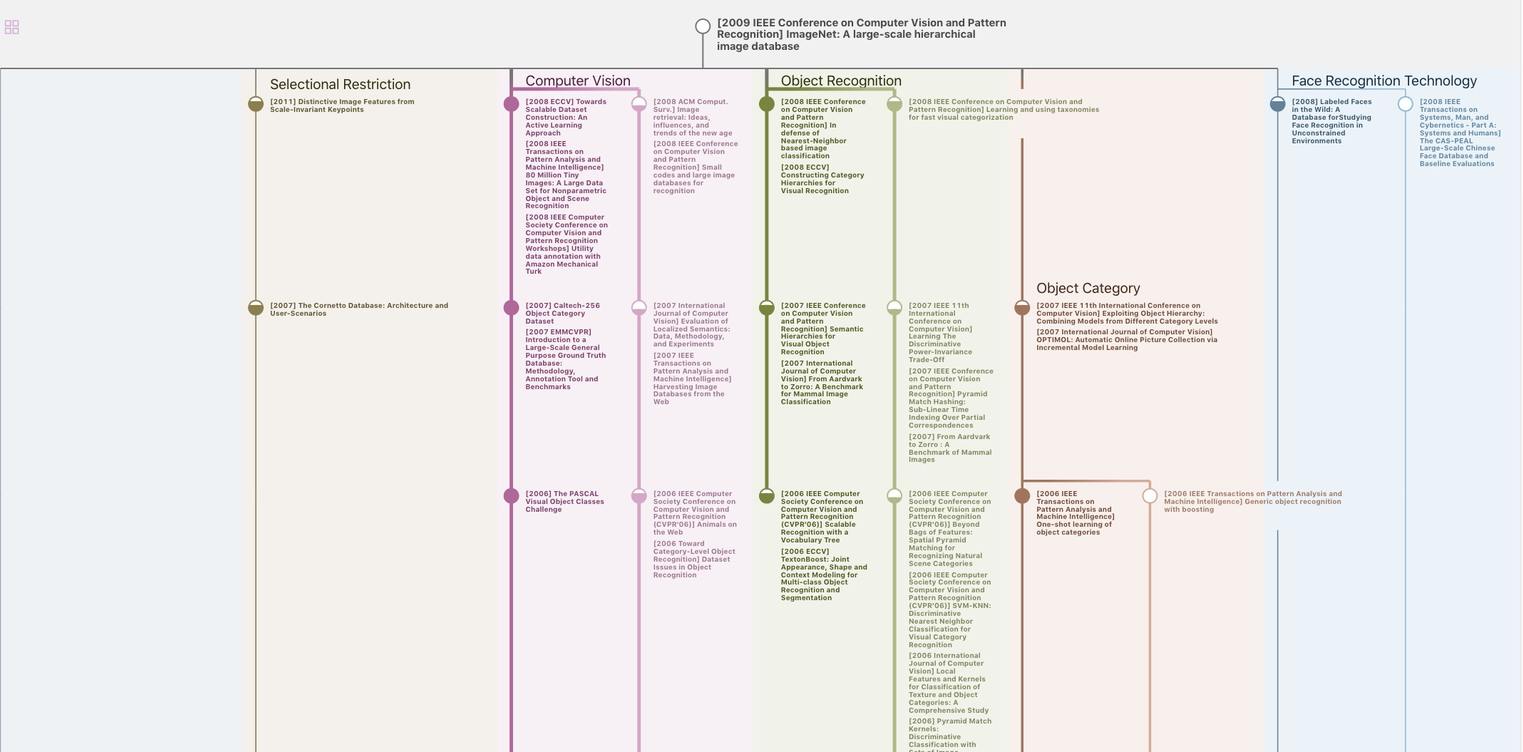
生成溯源树,研究论文发展脉络
Chat Paper
正在生成论文摘要