Real-Time 3D Head Pose Tracking Through 2.5D Constrained Local Models with Local Neural Fields
International Journal of Computer Vision(2019)
摘要
Tracking the head in a video stream is a common thread seen within computer vision literature, supplying the research community with a large number of challenging and interesting problems. Head pose estimation from monocular cameras is often considered an extended application after the face tracking task has already been performed. This often involves passing the resultant 2D data through a simpler algorithm that best fits the data to a static 3D model to determine the 3D pose estimate. This work describes the 2.5D constrained local model, combining a deformable 3D shape point model with 2D texture information to provide direct estimation of the pose parameters, avoiding the need for additional optimization strategies. It achieves this through an analytical derivation of a Jacobian matrix describing how changes in the parameters of the model create changes in the shape within the image through a full-perspective camera model. In addition, the model has very low computational complexity and can run in real-time on modern mobile devices such as tablets and laptops. The point distribution model of the face is built in a unique way, so as to minimize the effect of changes in facial expressions on the estimated head pose and hence make the solution more robust. Finally, the texture information is trained via local neural fields—a deep learning approach that utilizes small discriminative patches to exploit spatial relationships between the pixels and provide strong peaks at the optimal locations.
更多查看译文
关键词
Head pose estimation, 2.5D CLM, LNF, Real-time
AI 理解论文
溯源树
样例
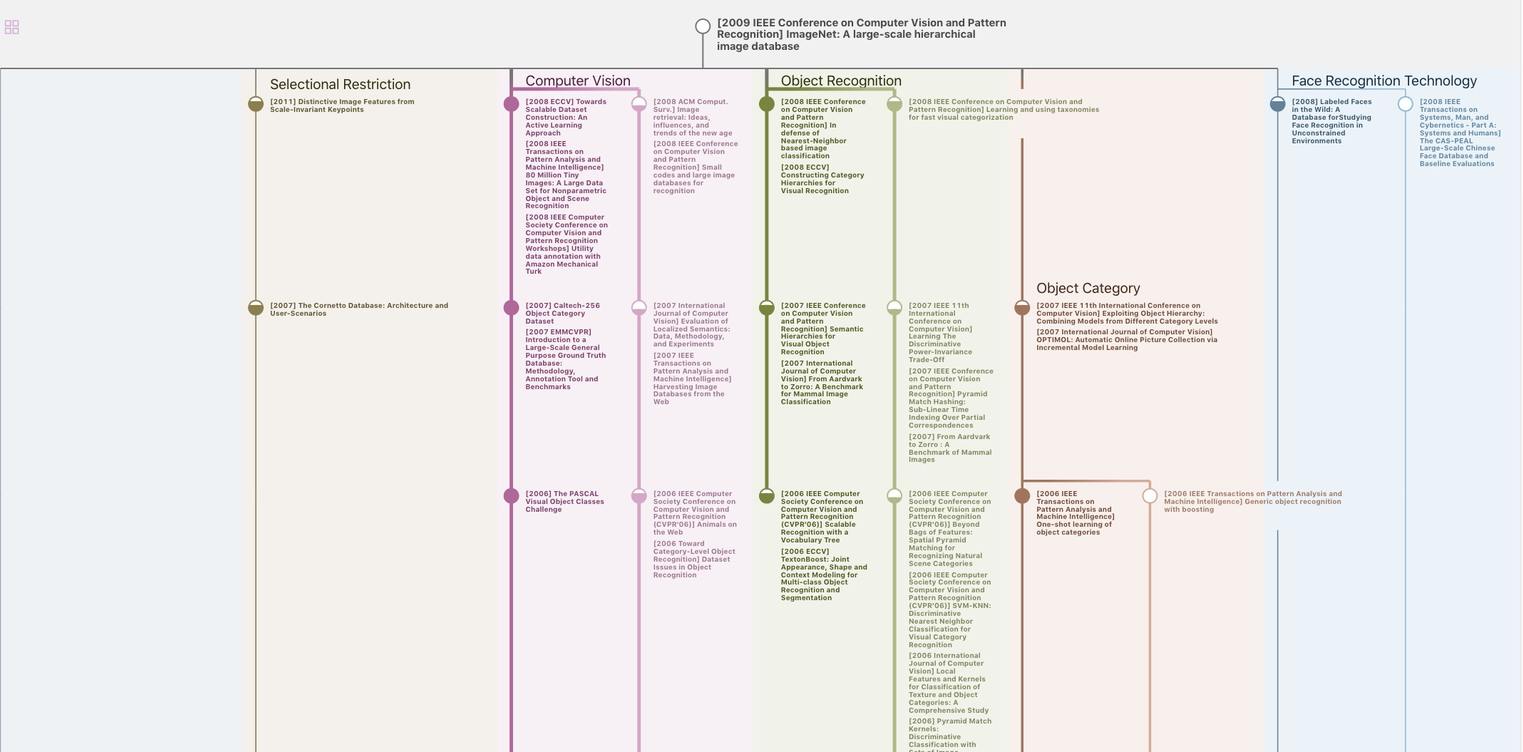
生成溯源树,研究论文发展脉络
Chat Paper
正在生成论文摘要