Unsupervised Anomaly Detection for Intricate KPIs via Adversarial Training of VAE
ieee international conference computer and communications(2019)
摘要
To ensure the reliability of the Internet-based application services, KPIs (Key Performance Monitors) are closely monitored in real time and the anomalies presented in the KPIs must be discovered in time. While anomaly detection for the seasonal smooth service-level KPIs (e.g., number of transactions per minute) have been solved reasonably well in the literature, the intricate KPIs at the machine level (e.g., the number of I/O requests on a server monitored per second) has been little studied. These intricate KPIs are prevalent and important, but exhibit non-Gaussian noises and complex data distribution that are hard to model. In this paper, we propose an adversarial training method in the Bayesian network based on partition analysis with solid theoretical proof. Based on it, we propose the first unsupervised anomaly detection algorithmBuzz for intricate KPIs with high performance. Its best F-scores on the data from a global Internet company range from 0.92 to 0.99, significantly outperforming a state-of-art VAE-based unsupervised approach without adversarial training and a state-of-art supervised approach.
更多查看译文
关键词
Training,Anomaly detection,Microsoft Windows,Monitoring,Bayes methods,Neural networks,Solids
AI 理解论文
溯源树
样例
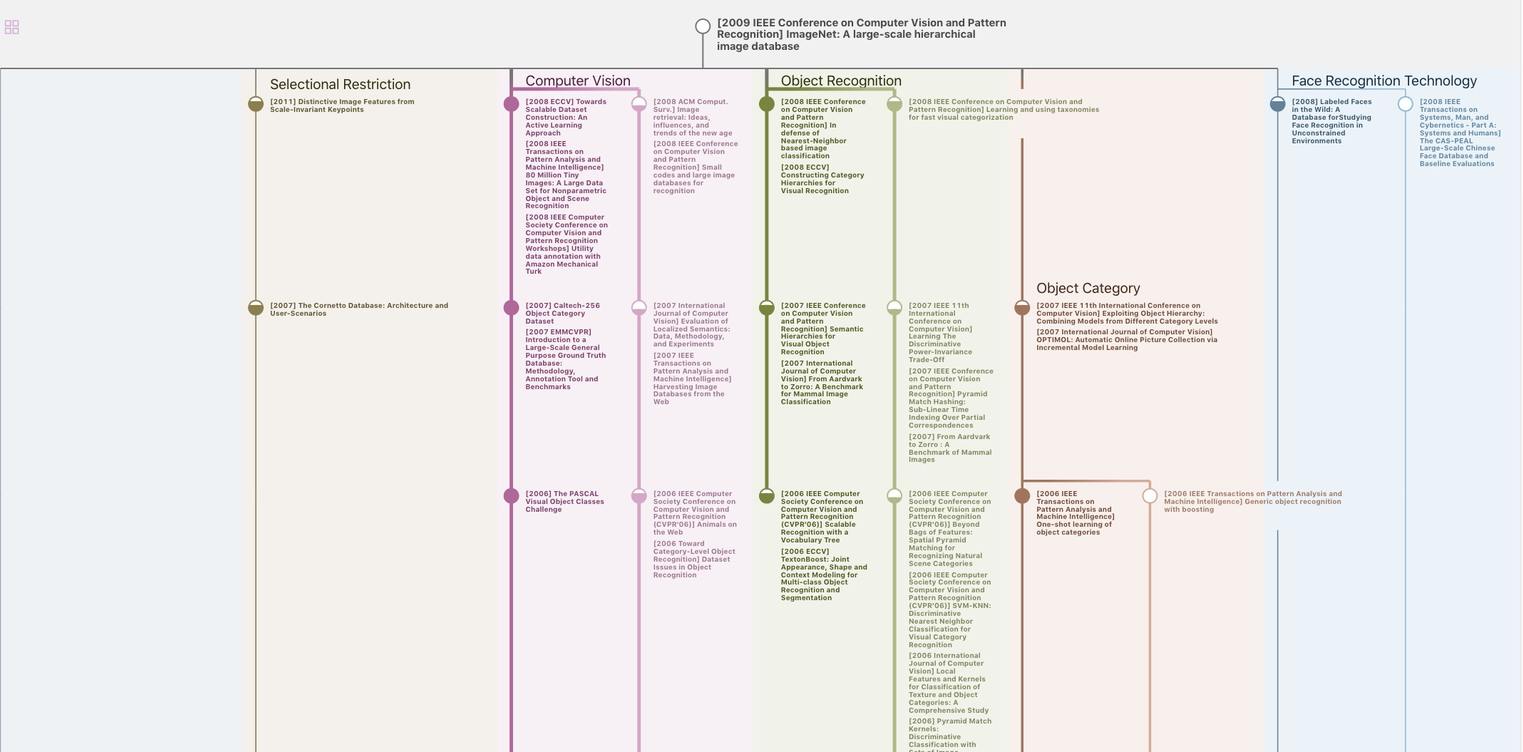
生成溯源树,研究论文发展脉络
Chat Paper
正在生成论文摘要