Gridded Monthly Rainfall Estimates derived from Historical Atoll Observations
JOURNAL OF ATMOSPHERIC AND OCEANIC TECHNOLOGY(2019)
Abstract
To provide an analysis tool for areal rainfall estimates, 1 degrees gridded monthly sea level rainfall estimates have been derived from historical atoll rainfall observations contained in the Pacific Rainfall (PACRAIN) database. The PACRAIN database is a searchable repository of in situ rainfall observations initiated and maintained by the University of Oklahoma and supported by a research grant from the National Oceanic and Atmospheric Administration (NOAA)/Climate Program Office/Ocean Observing and Monitoring. The gridding algorithm employs ordinary kriging, a standard geostatistical technique, and selects for nonnegative estimates and for local estimation neighborhoods yielding minimum kriging variance. This methodology facilitates the selection of fixed-size neighborhoods from available stations beyond simply choosing the closest stations, as it accounts for dependence between estimator stations. The number of stations used for estimation is based on bias and standard error exhibited under cross estimation. A cross validation is conducted, comparing estimated and observed rains, as well as theoretical and observed standard errors for the ordinary kriging estimator. The conditional bias of the kriging estimator and the predictive value of kriging standard errors, with respect to observed standard errors, are discussed. Plots of the gridded rainfall estimates are given for sample El Nino and La Nina cases and standardized differences between the estimates produced here and the merged monthly rainfall estimates published by the Global Precipitation Climatology Project (GPCP) are shown and discussed.
MoreTranslated text
Key words
Pacific Ocean,Rainfall,Interpolation schemes,Statistical techniques
AI Read Science
Must-Reading Tree
Example
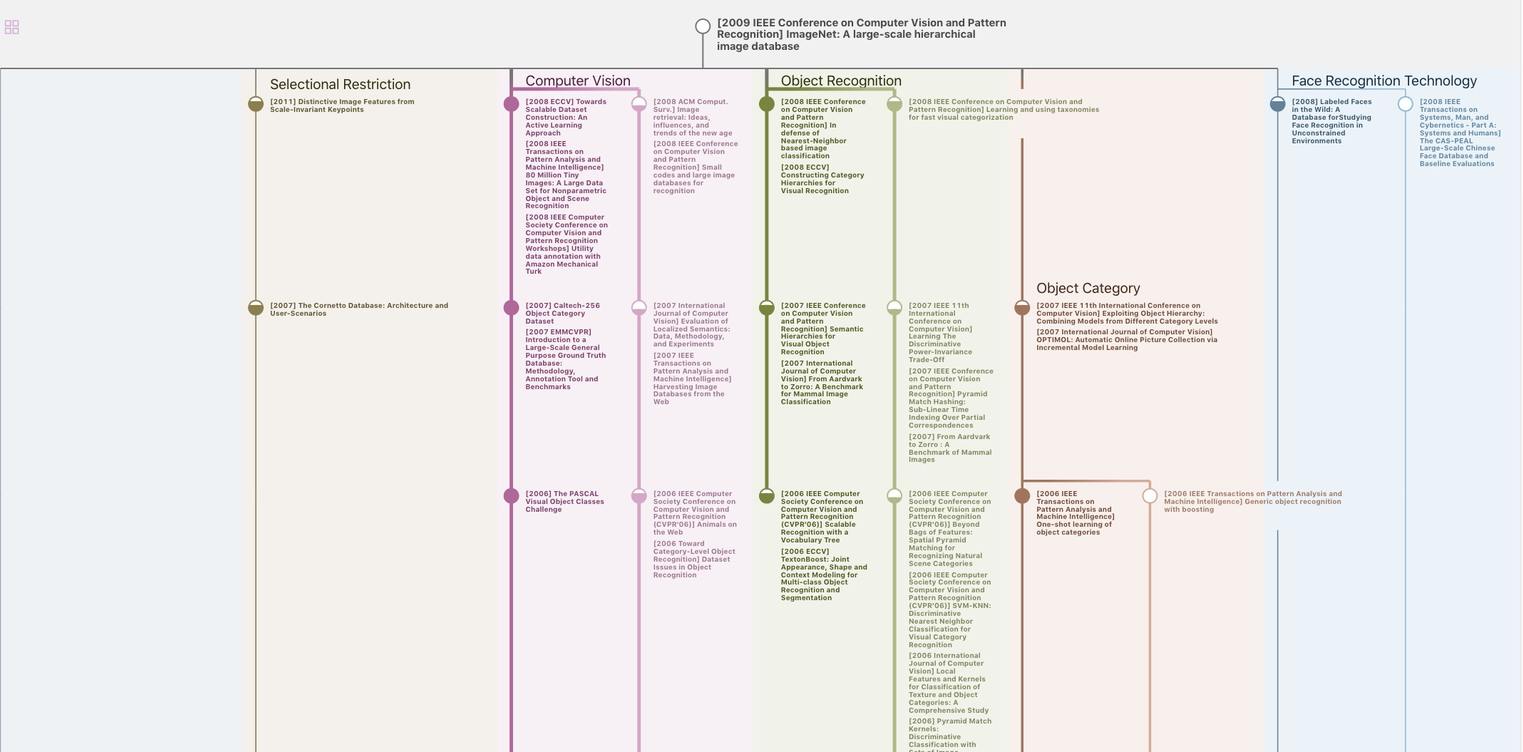
Generate MRT to find the research sequence of this paper
Chat Paper
Summary is being generated by the instructions you defined