Disagreement-Based Combinatorial Pure Exploration: Sample Complexity Bounds and an Efficient Algorithm.
COLT(2019)
摘要
We design new algorithms for the combinatorial pure exploration problem the multi-arm bandit framework. In this problem, we are given $K$ distributions and a collection of subsets $mathcal{V} 2^{[K]}$ of these distributions, and we would like to find the subset $v in mathcal{V}$ that has largest mean, while collecting, a sequential fashion, as few samples from the distributions as possible. In both the fixed budget and fixed confidence settings, our algorithms achieve new sample-complexity bounds that provide polynomial improvements on previous results some settings. Via an information-theoretic lower bound, we show that no approach based on uniform sampling can improve on ours any regime, yielding the first interactive algorithms for this problem with this basic property. Computationally, we show how to efficiently implement our fixed confidence algorithm whenever $mathcal{V}$ supports efficient linear optimization. Our results involve precise concentration-of-measure arguments and a new algorithm for linear programming with exponentially many constraints.
更多查看译文
AI 理解论文
溯源树
样例
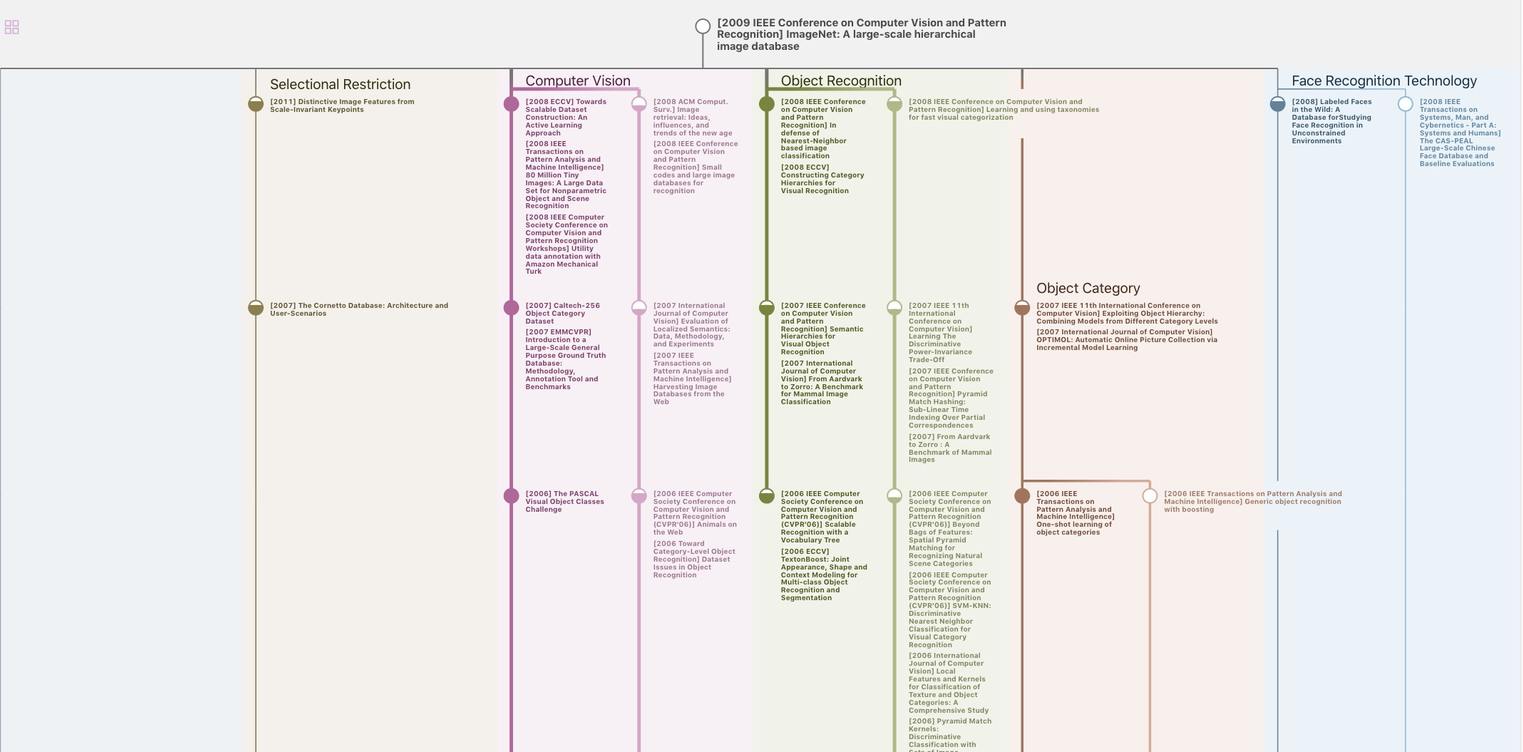
生成溯源树,研究论文发展脉络
Chat Paper
正在生成论文摘要