Intelligent Fault Identification Based on Multi-Source Domain Generalization Towards Actual Diagnosis Scenario
IEEE Transactions on Industrial Electronics(2020)
摘要
The data-driven diagnosis methods based on conventional machine-learning techniques have been widely developed in recent years. However, the assumption of conventional methods that the training and test data should be identically distributed is usually unsatisfied in actual diagnosis scenario. While there are several existing works that have been studied to construct diagnosis models by transfer learning methods, most of them are only focused on learning from a single source. Actually, how to discover effective and general diagnosis knowledge from multiple related source domains and further generalize the learned knowledge to new target tasks is crucial to data-driven fault diagnosis. To this end, this paper proposes a novel intelligent fault identification method based on multiple source domains. First, the method describes the discriminant structure of each source domain as a point of Grassmann manifold using local Fisher discriminant analysis. Through preserving the within-class local structure, local Fisher discriminant analysis can learn effective discriminant directions from multimodal fault data. Second, the mean subspace of source domains is computed on the Grassmann manifold through Karcher mean. The mean subspace can be viewed as a representation of the general diagnosis structure that can facilitate the construction of the diagnosis model for the target domain. Experiments on bearing fault diagnosis tasks verify the effectiveness of the proposed method.
更多查看译文
关键词
Fault diagnosis,Manifolds,Training,Task analysis,Neural networks,Data models,Knowledge transfer
AI 理解论文
溯源树
样例
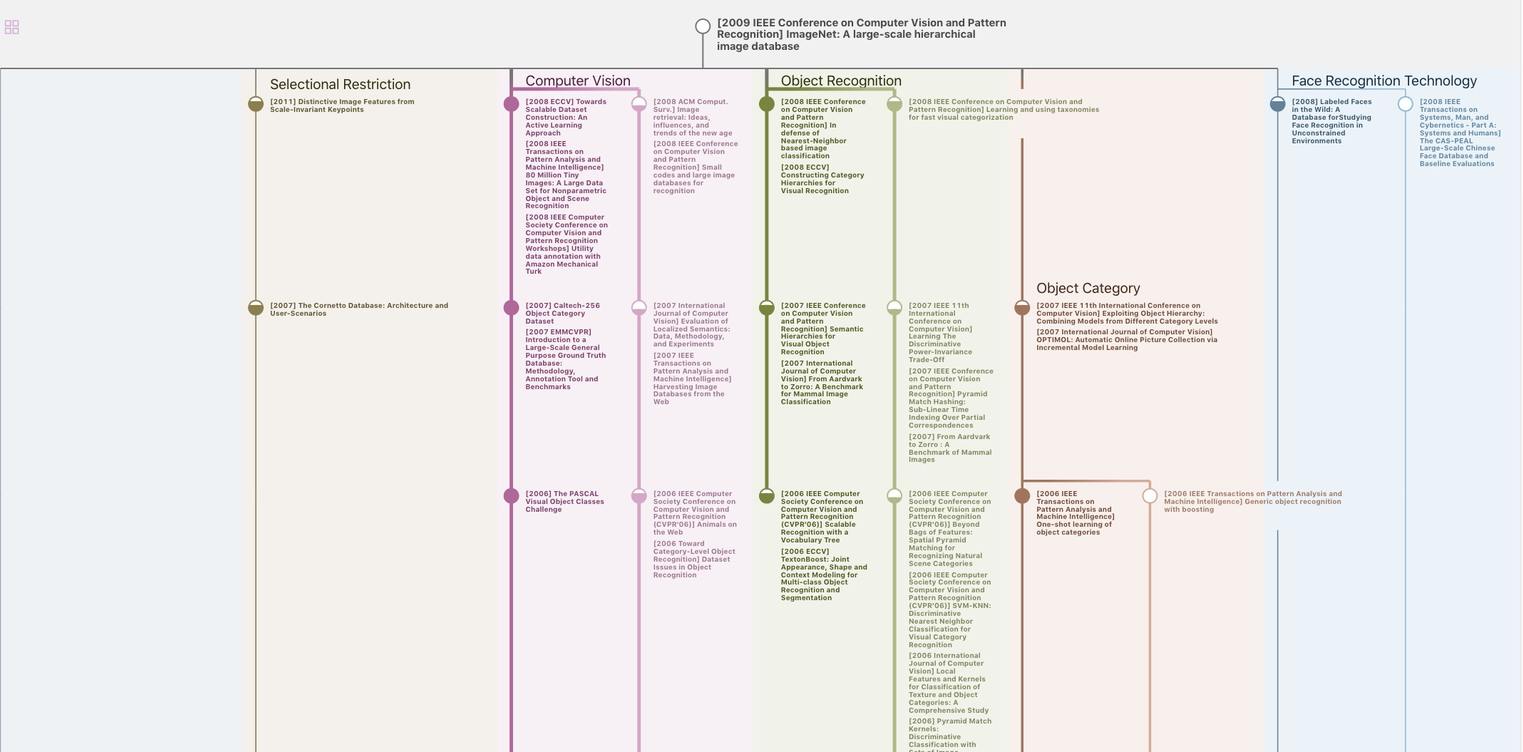
生成溯源树,研究论文发展脉络
Chat Paper
正在生成论文摘要