Extraction of Electromyographic Signals for Multiple-Hand-Motion Recognition via Sparse Representation Technique
2018 CHINESE AUTOMATION CONGRESS (CAC)(2018)
摘要
In order to improve recognition accuracies of hand multi-movement patterns, we investigate a feature extraction method based on sparse representation technique. As a new tool, sparse Bayesian learning (SBL) algorithm is adopted to reveal the hidden nonstationary characteristics of surface electromyography (sEMG) during multiple different wrist, hand and finger movements. In the experiment on classifying these movements for fifteen participants, the feature we proposed has the optimal class separability. It can effectively distinguish multiple different hand movements. The average correct recognition rate of ten movements is up to 89.40%. Meanwhile, Davies-Bouldlin index (DBI) is applied to evaluate the performance of proposed method for identifying hand multi-movement patterns. The experiment results suggest that the SBL based method is an effective tool to capture the hidden characteristics of sEMG signals during ten different movements. Using the proposed method, the performance of myoelectric pattern recognition is enhanced.
更多查看译文
关键词
surface electromyography (sEMG), multiple-hand-motion recognition, time-varying sparsity, sparse Bayesian learning (SBL)
AI 理解论文
溯源树
样例
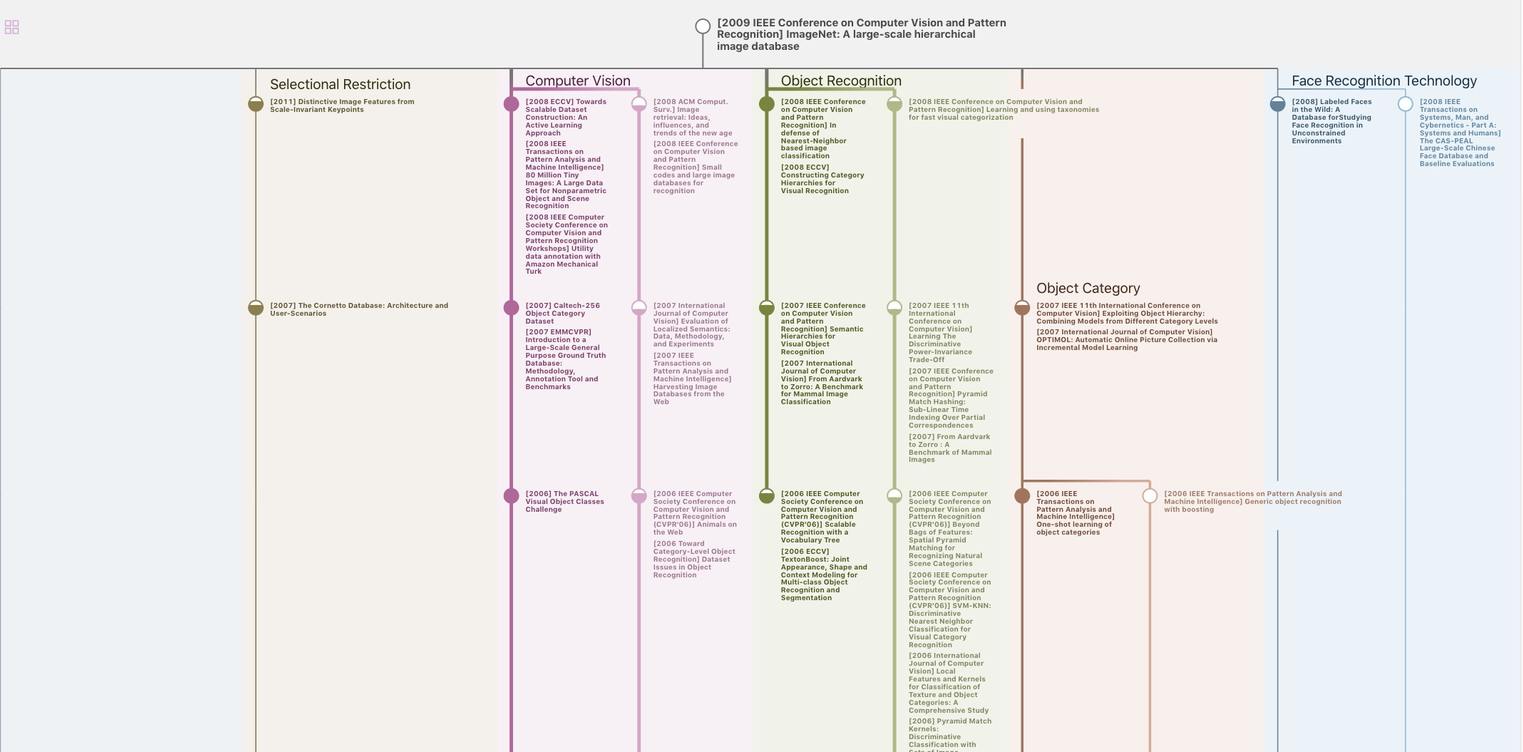
生成溯源树,研究论文发展脉络
Chat Paper
正在生成论文摘要