A nonlinear and explicit framework of supervised manifold-feature extraction for hyperspectral image classification
Neurocomputing(2019)
摘要
Hyperspectral remote sensing has drawn great research interests in earth observation, since massive and contiguous spectrum can provide rich information of ground objects. However, such numerous bands also pose great challenges to efficient processing of hyperspectral images (HSI). Manifold learning has been widely used in feature extraction of HSI data to find intrinsic and compact representations of original spectrum. Nevertheless, lack of explicit and nonlinear mapping is still a critical limitation. In this paper, we propose a supervised learning framework for manifold-learning based HSI feature extraction and classification, which provides a nonlinear and explicit mapping for fast and efficient feature learning. We also introduce two concrete learning methods that are induced from this framework, which can extract different intrinsic topology of HSI data to achieve nonlinear dimensionality reduction. Experiments conducted on benchmark data sets demonstrate that high classification accuracy can be obtained with the proposed framework, which consistently outperforms linear mappings for manifold learning methods.
更多查看译文
关键词
Hyperspectral images,Manifold learning,Nonlinear mapping,Supervised learning
AI 理解论文
溯源树
样例
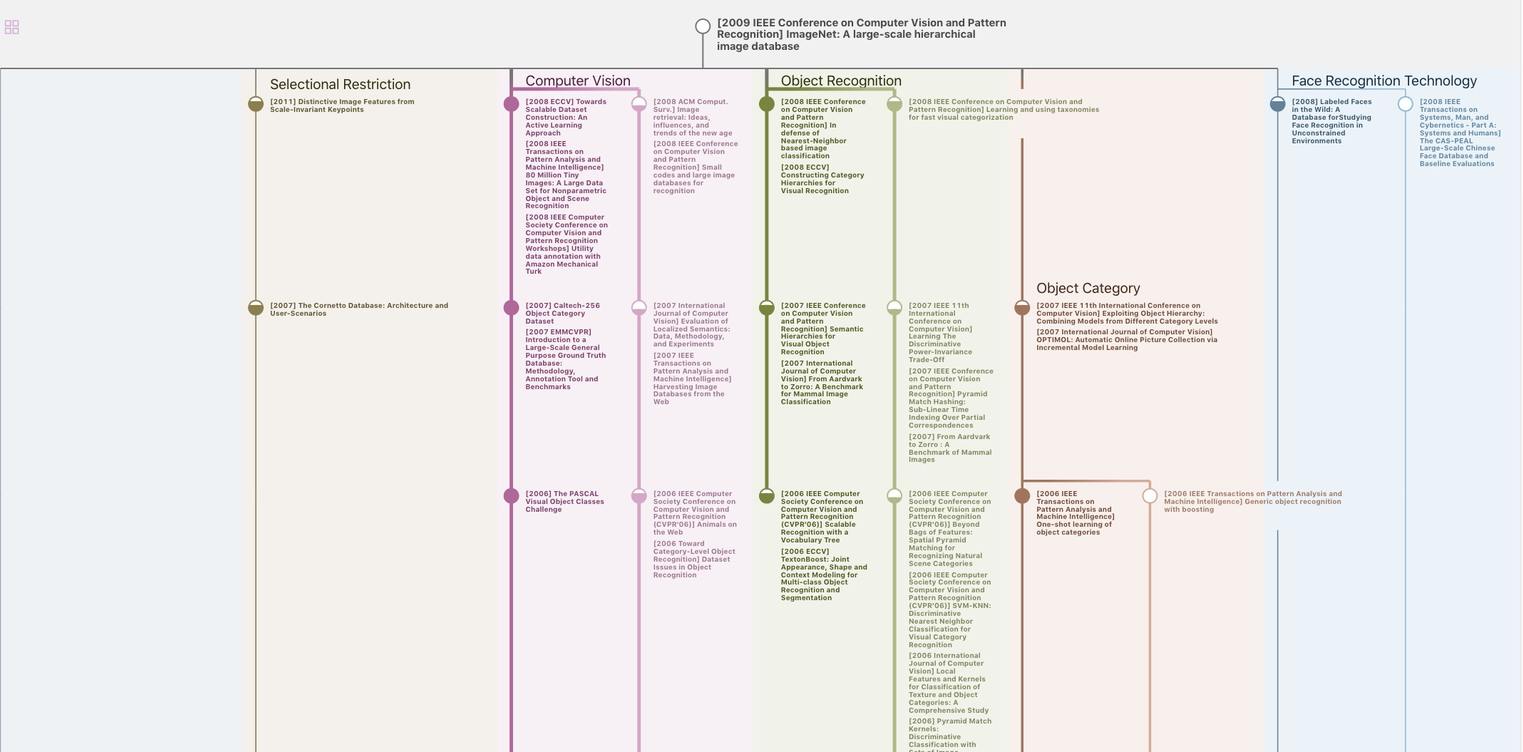
生成溯源树,研究论文发展脉络
Chat Paper
正在生成论文摘要