Deep Learning For Sleep Stage Classification
2018 CHINESE AUTOMATION CONGRESS (CAC)(2018)
Abstract
Scoring of sleep stages plays an important role in the diagnosis of sleep-related diseases. Scoring by visual inspection is time-consuming and heavily depends on the experience of experts. Thus, there is an urgent need for an automatic sleep stage classification system. This paper proposes a novel compact convolutional neural network (C-CNN) using only single-channel EEG signal. Compared with traditional machine learning approaches based on hand-engineered features, our approach provides an end-to-end solution that requires almost no prior knowledge and preprocessing while achieving better performance. Experiments on the expanded Sleep-EDF database verified its effectiveness and efficiency. In addition, we notice the issue of class imbalance in sleep stages, and a class-imbalance metric, the balanced classification accuracy (BCA), is introduced. At the cost of a little drop in accuracy, which is still higher than existing classification methods, the introduction of class-imbalance weights can significantly increase the BCA metric and result in a higher recall for each sleep stage. This paper also proposes a recurrent neural network based on the attention mechanism and bidirectional long short-term memory (LSTM), which provides better performance than C-CNN but requires more training time.
MoreTranslated text
Key words
Sleep stage classification, convolutional neural network, attention, long short-term memory, class imbalance
AI Read Science
Must-Reading Tree
Example
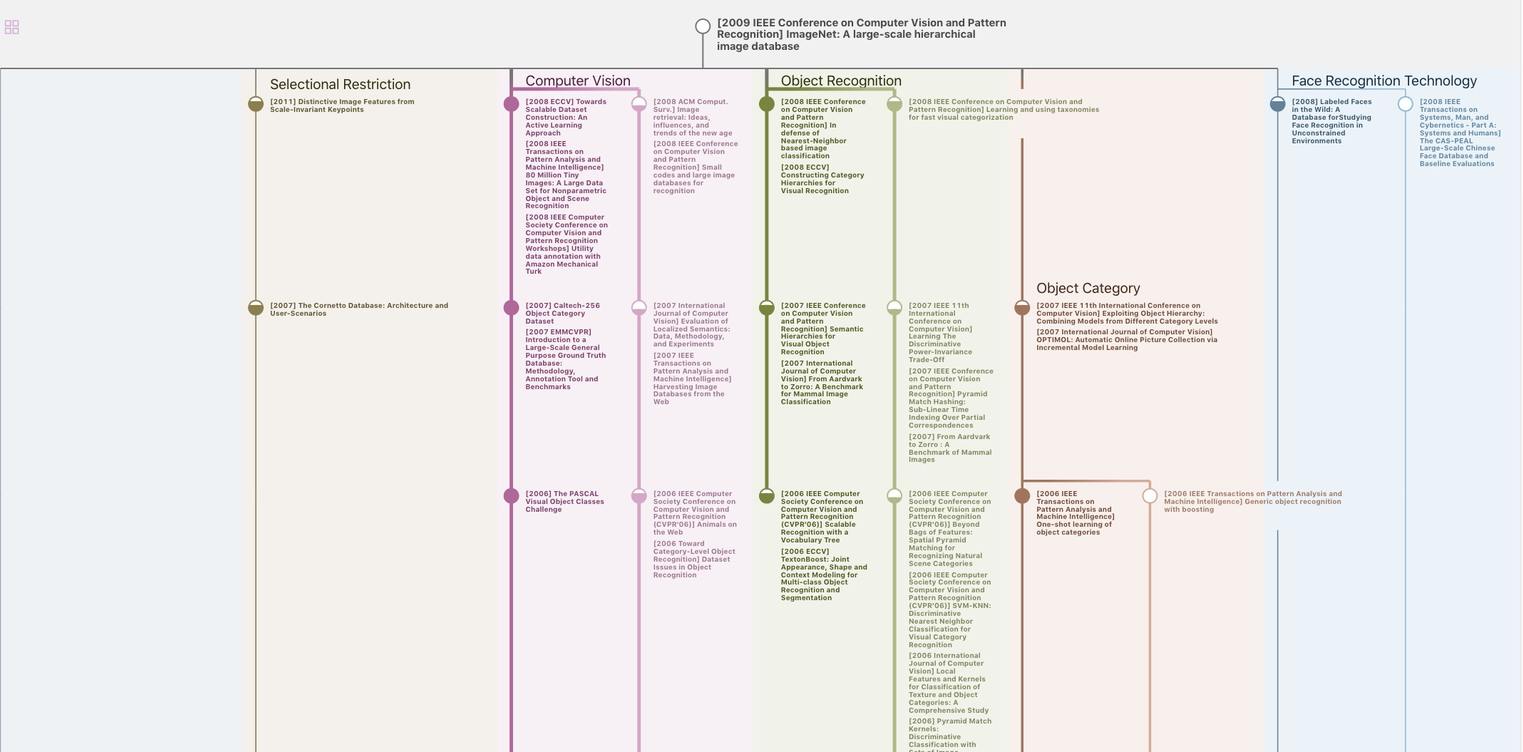
Generate MRT to find the research sequence of this paper
Chat Paper
Summary is being generated by the instructions you defined