Bayesian non-crossing quantile regression for regularly varying distributions
JOURNAL OF STATISTICAL COMPUTATION AND SIMULATION(2019)
Abstract
Quantile regression is a very important statistical tool for predictive modelling and risk assessment. For many applications, conditional quantile at different levels are estimated separately. Consequently the monotonicity of conditional quantiles can be violated when quantile regression curves cross each other. In this paper, we propose a new Bayesian multiple quantile regression based on heavy tailed distribution for non-crossing. We consider a linear quantile regression model for simultaneous Bayesian estimation of multiple quantiles based on a regularly varying assumptions. The numerical and competitive performance of the proposed method is illustrated by simulation.
MoreTranslated text
Key words
Quantile regression,non-crossing curves,regularly varying distributions,heavy tailed behaviour,conditional quantile curves
AI Read Science
Must-Reading Tree
Example
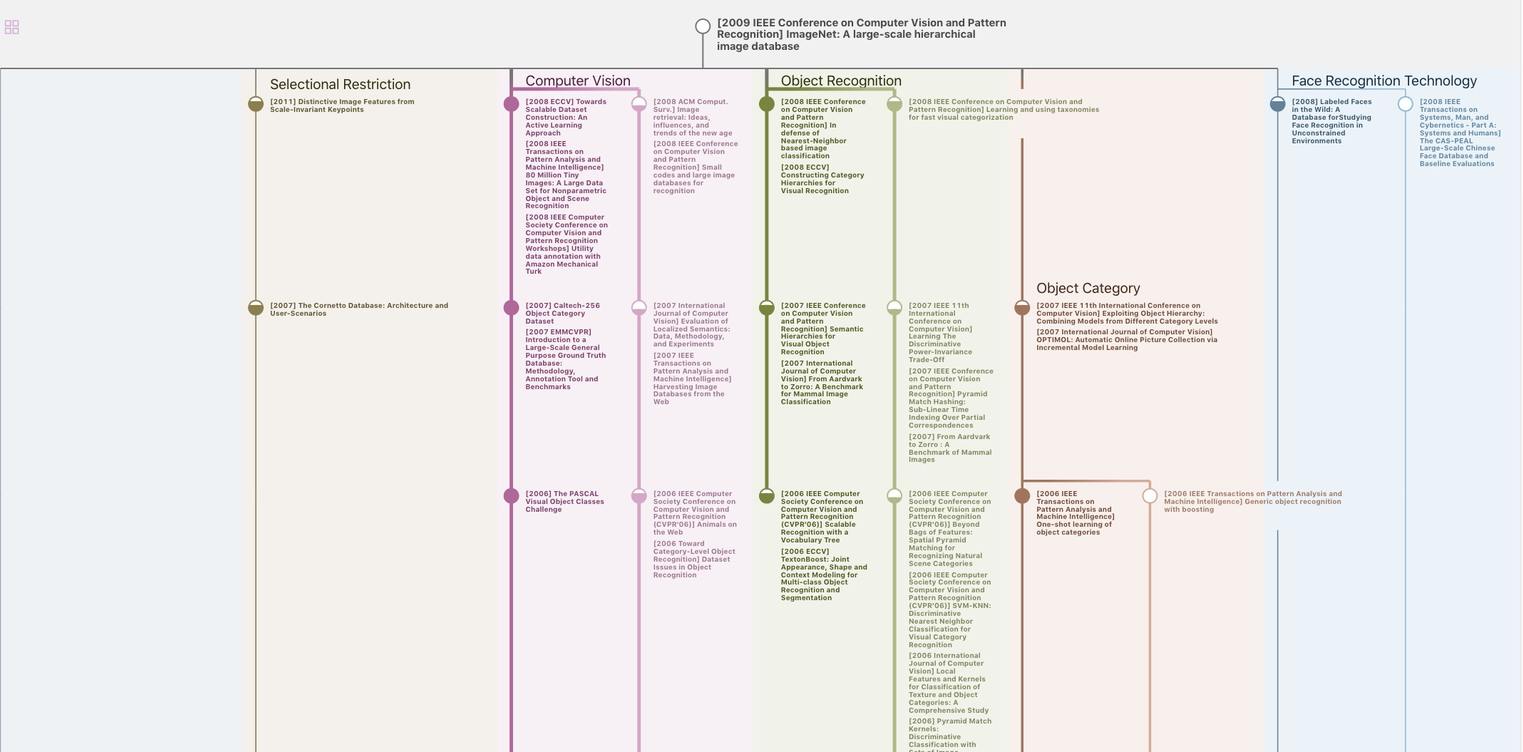
Generate MRT to find the research sequence of this paper
Chat Paper
Summary is being generated by the instructions you defined