Cooperative population coding facilitates efficient sound source separability by adaptation to spatial statistics
bioRxiv(2019)
摘要
Our sensory environment changes constantly. Accordingly, neural systems continually adapt to the concurrent stimulus statistics to remain sensitive over a wide range of conditions. Such dynamic range adaptation (DRA) is assumed to increase both the effectiveness of the neuronal code and perceptual sensitivity. However, direct demonstrations of DRA-based neuronal processing that also produces perceptional benefits are lacking. Here we investigated the impact of DRA on spatial in the rodent brain and the perception of human listeners. Naturalistic spatial stimulation with dynamically changing source locations elicited prominent DRA already on the initial spatial processing stage, the Lateral Superior Olive (LSO) of gerbils of either sex. Surprisingly, on the level of individual neurons, DRA diminished spatial tuning due to large response variability across trials. However, when considering single-trial population averages of multiple neurons, DRA enhanced the efficiency specifically for the concurrently most probable source locations. Intrinsic LSO population imaging of energy consumption combined with pharmacology revealed that a slow-acting LSO gain control mechanism distributes activity across a group of neurons during DRA, thereby enhancing population efficiency. Strikingly, such efficient cooperative coding also improved neuronal source separability specifically for the locations that were most likely to occur. These location-specific enhancements in neuronal were paralleled by human listeners exhibiting a selective improvement in spatial resolution. We conclude that, contrary to canonical models of sensory encoding, the primary motive of early spatial processing is efficiency optimization of neural populations for enhanced source separability in the concurrent environment.
更多查看译文
AI 理解论文
溯源树
样例
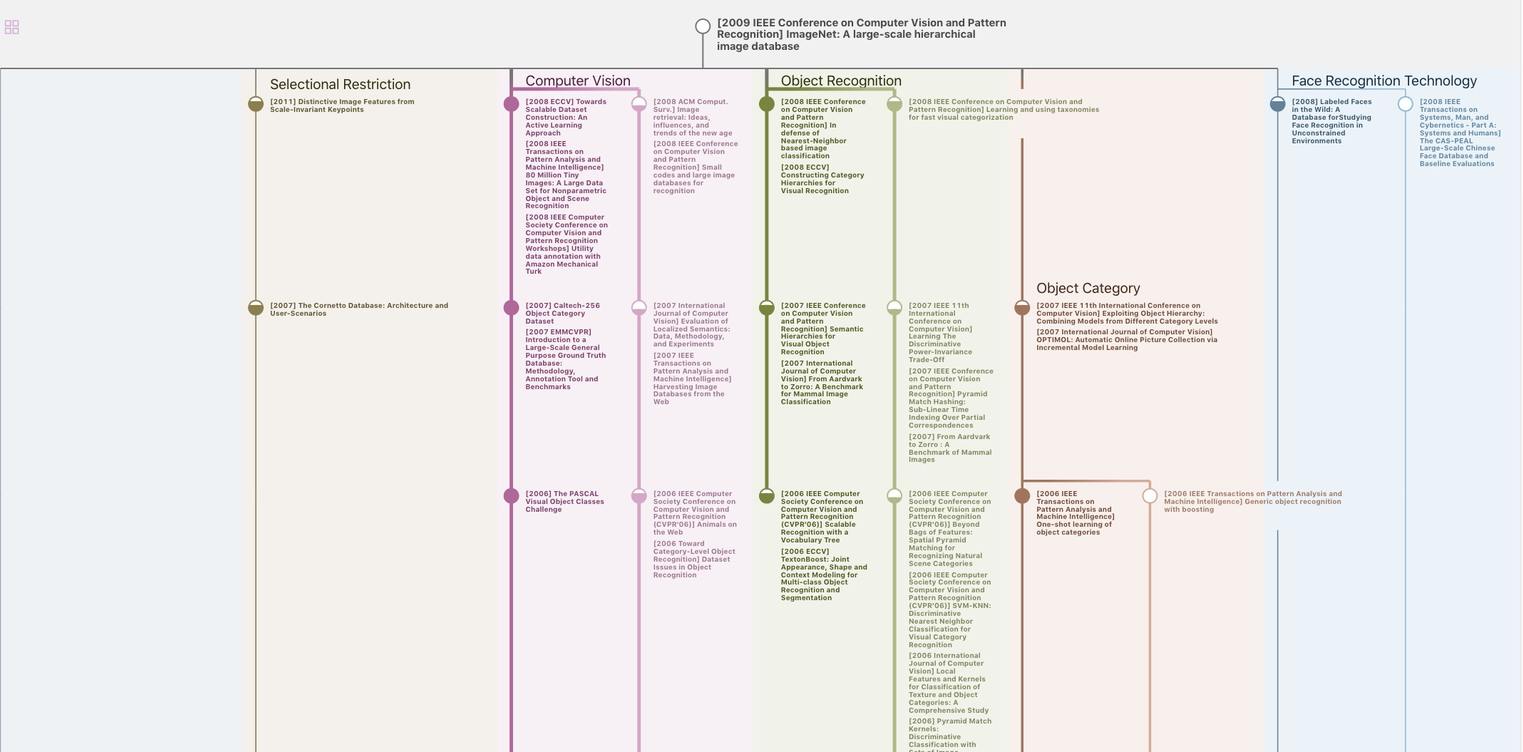
生成溯源树,研究论文发展脉络
Chat Paper
正在生成论文摘要