Computational analysis of molecular networks using spectral graph theory, complexity measures and information theory
bioRxiv(2019)
摘要
Molecular networks are modeled as directed multigraphs, so-called network motifs. Spectral graph theory, reciprocal link and complexity measures were utilized to quantify network motifs. It was found that graph energy, reciprocal link and cyclomatic complexity can optimally specify network motifs with some degree of degeneracy. Biological networks are built up from a finite number of motif patterns; hence, a graph energy cutoff exists and the Shannon entropy of the motif frequency distribution is not maximal. Also, frequently found motifs are irreducible graphs. Network similarity was quantified by gauging their motif frequency distribution functions using Jensen-Shannon entropy. This method allows us to determine the distance between two networks regardless of their node identities and network sizes. This study provides a systematic approach to dissect the complex nature of biological networks. Our novel method is different from any other approach. The findings support the view that there are organizational principles underlying molecular networks.
更多查看译文
关键词
network motifs,cancer networks,signal transduction networks,cellular processes,graph theory,information theory,graph energy,network complexity,entropy,Jensen-Shannon entropy
AI 理解论文
溯源树
样例
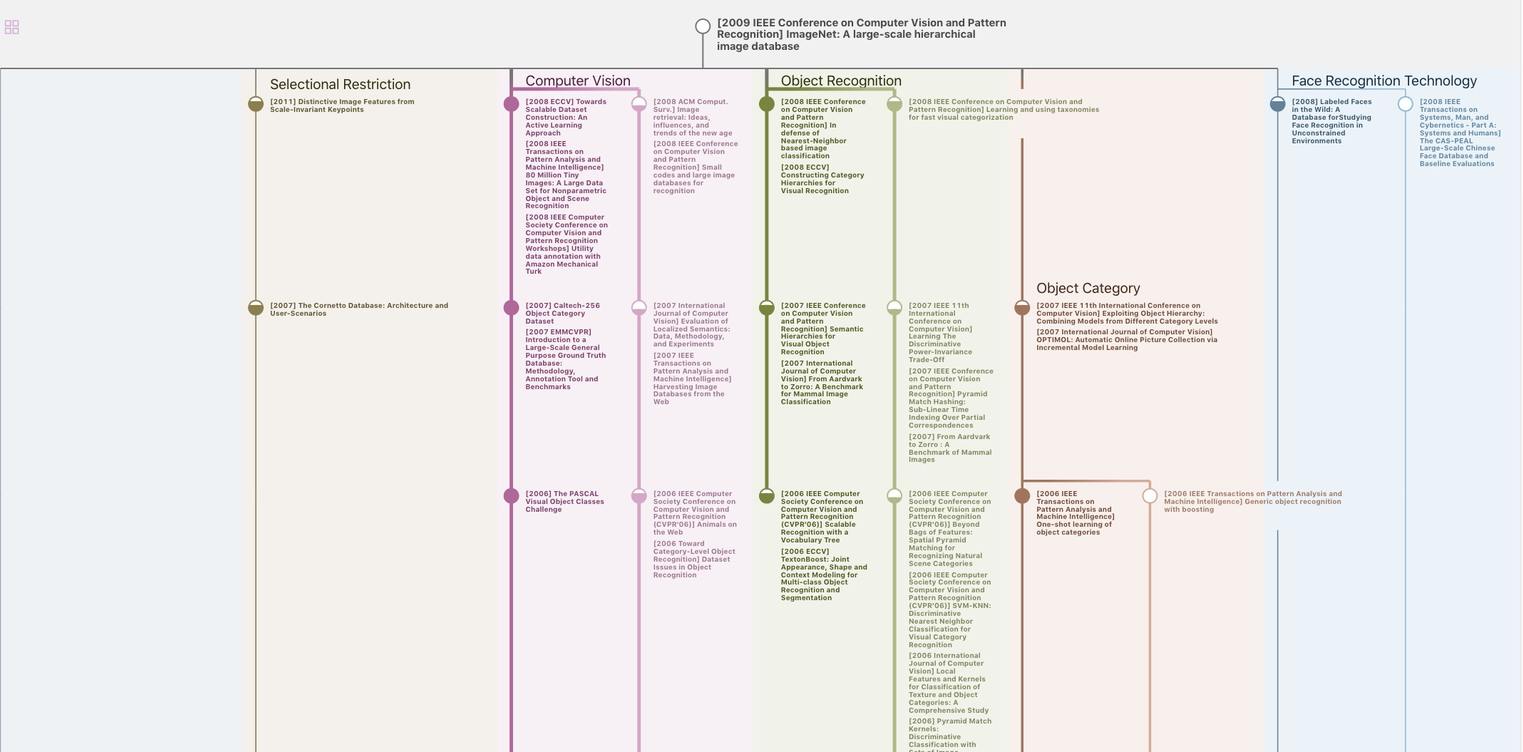
生成溯源树,研究论文发展脉络
Chat Paper
正在生成论文摘要