Deep Learning based Channel Estimation and Equalization Scheme for FBMC/OQAM Systems
IEEE Wireless Communications Letters(2019)
摘要
Filter bank multicarrier (FBMC) modulation is a promising candidate modulation method for future communication systems. However, FBMC system cannot directly use channel estimation methods proposed for orthogonal frequency-division multiplexing (OFDM) system due to its inherent imaginary interference. In this letter, we propose a channel estimation and equalization scheme based on deep learning (DL-CE) for FBMC systems. In the DL-CE scheme, the channel state information (CSI) and the constellation demapping method are learned by a deep neural networks (DNN) model, and then the distorted frequency-domain sequences are equalized implicitly to obtain binary bits directly. Simulation results show that the proposed DL-CE scheme achieves state-of-the-art performance on channel estimation and equalization.
更多查看译文
关键词
Channel estimation,Interference,Quadrature amplitude modulation,Neurons,Training,Deep learning,OFDM
AI 理解论文
溯源树
样例
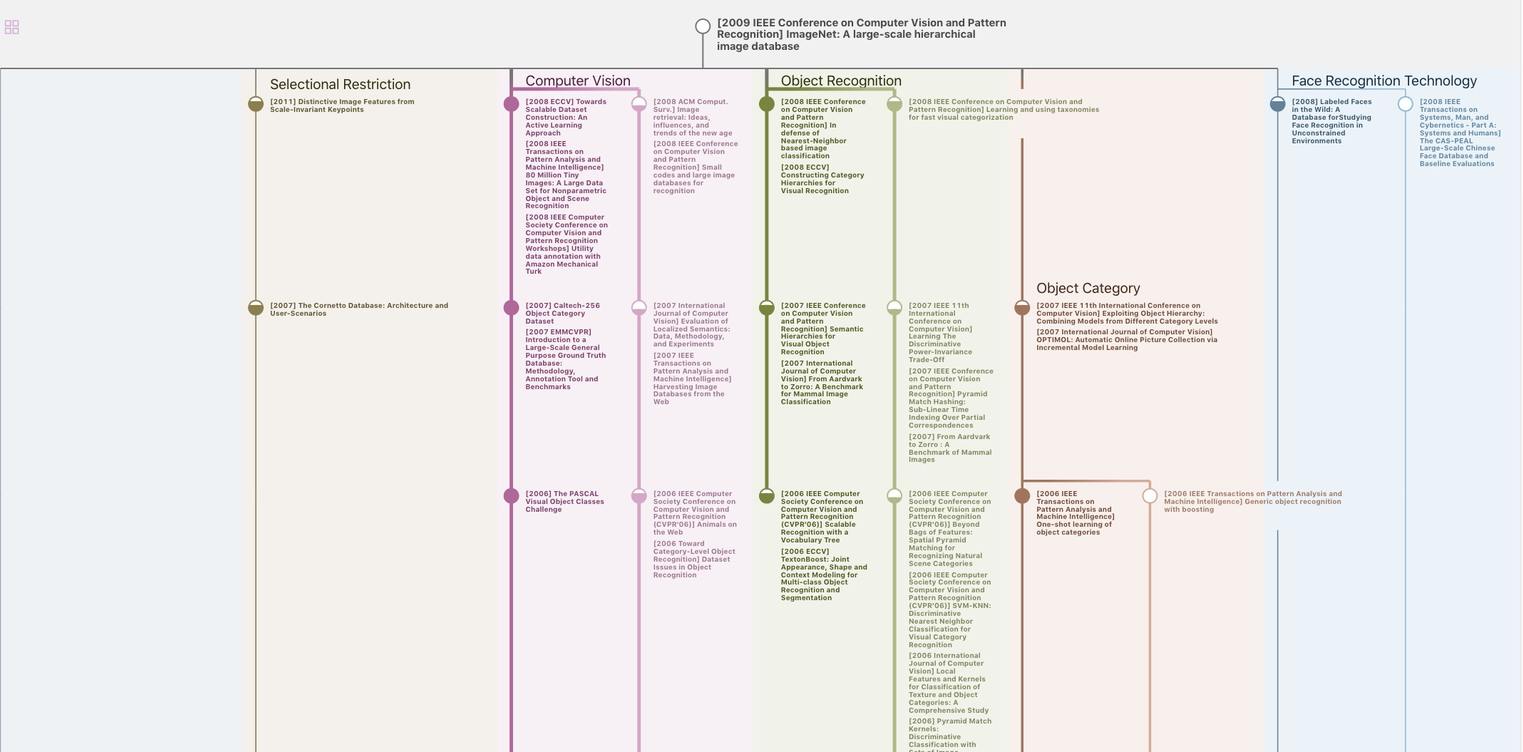
生成溯源树,研究论文发展脉络
Chat Paper
正在生成论文摘要