Modelling and mapping soil organic carbon stocks in Brazil
Geoderma(2019)
摘要
Brazil has extensive forests and savannas on deep weathered soils and plays a key role in the discussions about carbon sequestration, but the distribution of soil organic carbon (SOC) stocks up to 1 m depth has not been investigated in Brazil using machine learning techniques. In this study, we applied a methodological framework to optimize the prediction of SOC stocks for the entire Brazilian territory and determine how the environmental heterogeneity of Brazil influences the SOC stocks distribution. We used a legacy dataset of 8227 soil profiles which consisted of 37,693 samples. For each profile, the vertical distribution of SOC and bulk density were interpolated to standard depths (0–5, 5–15, 15–30, 30–60 and 60–100 cm) using mass preserving equal-area quadratic splines. The covariates database was composed of 74 variables including bioclimatic (temperature and precipitation) data, soil and biome maps, vegetation indexes and morphometric maps derived from a digital elevation model, with a 1 km spatial resolution. To obtain the best prediction performance, we tested four machine learning algorithms: Random Forests, Cubist, Generalized Linear Model Boosting and Support Vector Machines. Random Forests showed the best performance in predicting SOC stocks for all depths, with the highest performance at 30–60 cm for training (R2 = 0.32) and validation (R2 = 0.33); hence, it was selected for the spatial prediction of SOC stocks. The most important covariates selected by Random Forests using the recursive feature elimination were: soil class, sum of monthly mean temperature (SAMT), precipitation, slope height and vegetation indexes (NDVI, GPP). In total, Brazilian soils store approximately 71.3 PgC within the top 100 cm, where the first 0–30 cm contains almost 36 PgC. Approximately 31% of the total SOC stocks (22.2 PgC) occurs in protected areas (2.6 million km2), which are not subjected to land use pressure and carbon losses. Although the Amazon biome has the highest amount of stored SOC (36.1 PgC), its soils do not represent a good potential for carbon accumulation. Among soil classes, the Luvisols showed the lowest SOC density (6.45 kg m−2) and the Histosols presented the highest values (14.87 kg m−2). More than 57% of the total SOC was found in nutrient-poor, deep-weathered Ferralsols and Acrisols, which are the dominant soils in Brazil. The presented methodological framework covers all steps of prediction process, building maps with known accuracy and has great potential to be used in future soil carbon inventories at large scales. Concerning conservation issues, the results highlight the importance of nature reserves for protecting SOC in the long-term.
更多查看译文
关键词
Soil carbon stock,Machine learning,Spatial prediction,Protected areas,Random Forests
AI 理解论文
溯源树
样例
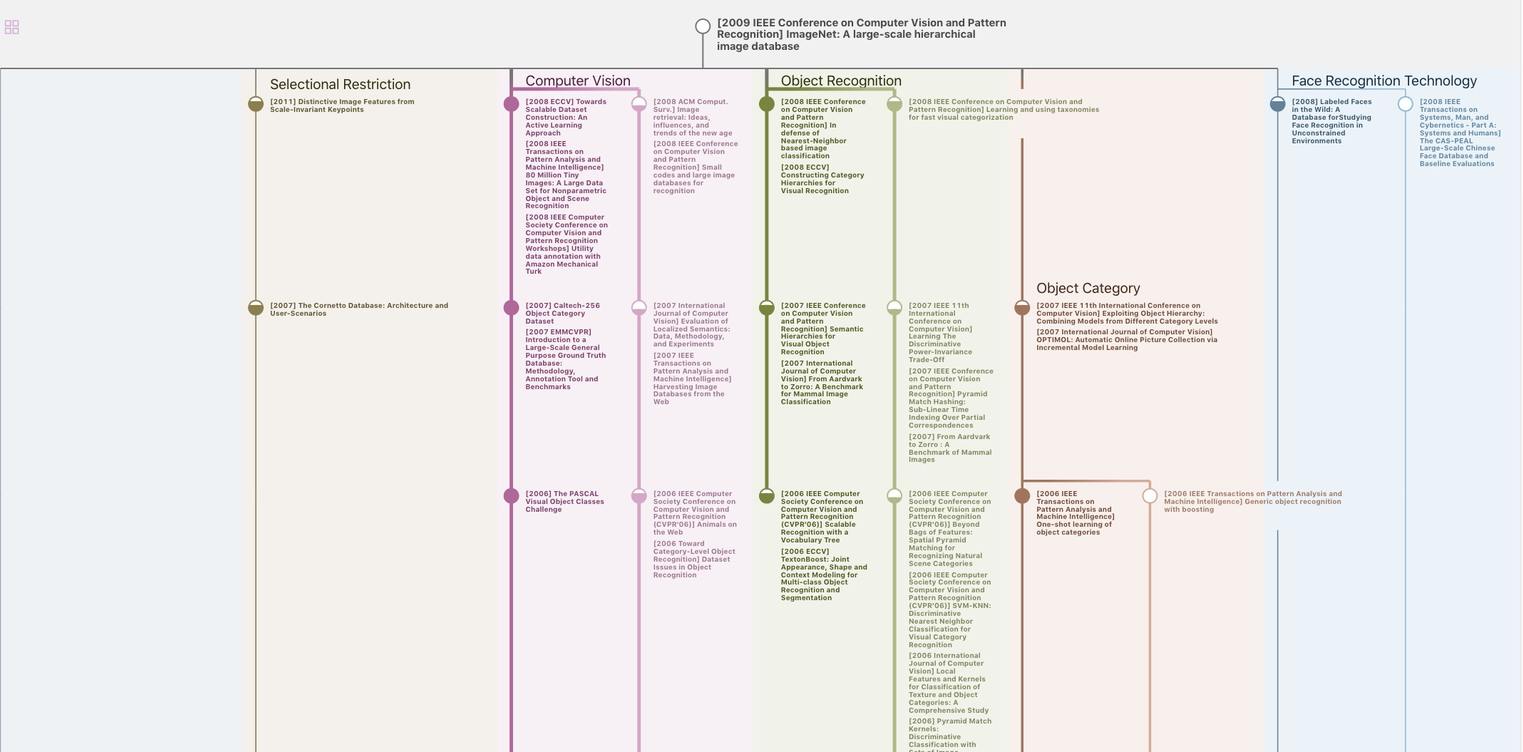
生成溯源树,研究论文发展脉络
Chat Paper
正在生成论文摘要