Weakly Supervised Learning Of Object-Part Attention Model For Fine-Grained Image Classification
2018 IEEE 18TH INTERNATIONAL CONFERENCE ON COMMUNICATION TECHNOLOGY (ICCT)(2018)
摘要
Fine-grained classification is challengeable due to the small inter-class variance and large intra-class distance between fine-grained categories. The key to solve this problem is to locate the discriminative part in the image. In this paper we propose a weakly supervised method, which only need image-level label for fine-grained classification. In our model, the convolutional neural network (CNN) can location the discriminative region through attention, and automatically focus on subtler features by zooming the discriminative region and feeding it to the next CNN. A Squeeze and Excitation (SE) module is employed for channel-wise attention, and a spatial constrain loss is utilized to keep the diversity of located part. We conduct experiments on CUB-2011-200, Stanford Dogs, and Stanford Cars datasets to evaluate the performance of our model. The experimental results demonstrate the effectiveness of the proposed method as compared other methods.
更多查看译文
关键词
fine-grained classification, visual attention, weakly supervised
AI 理解论文
溯源树
样例
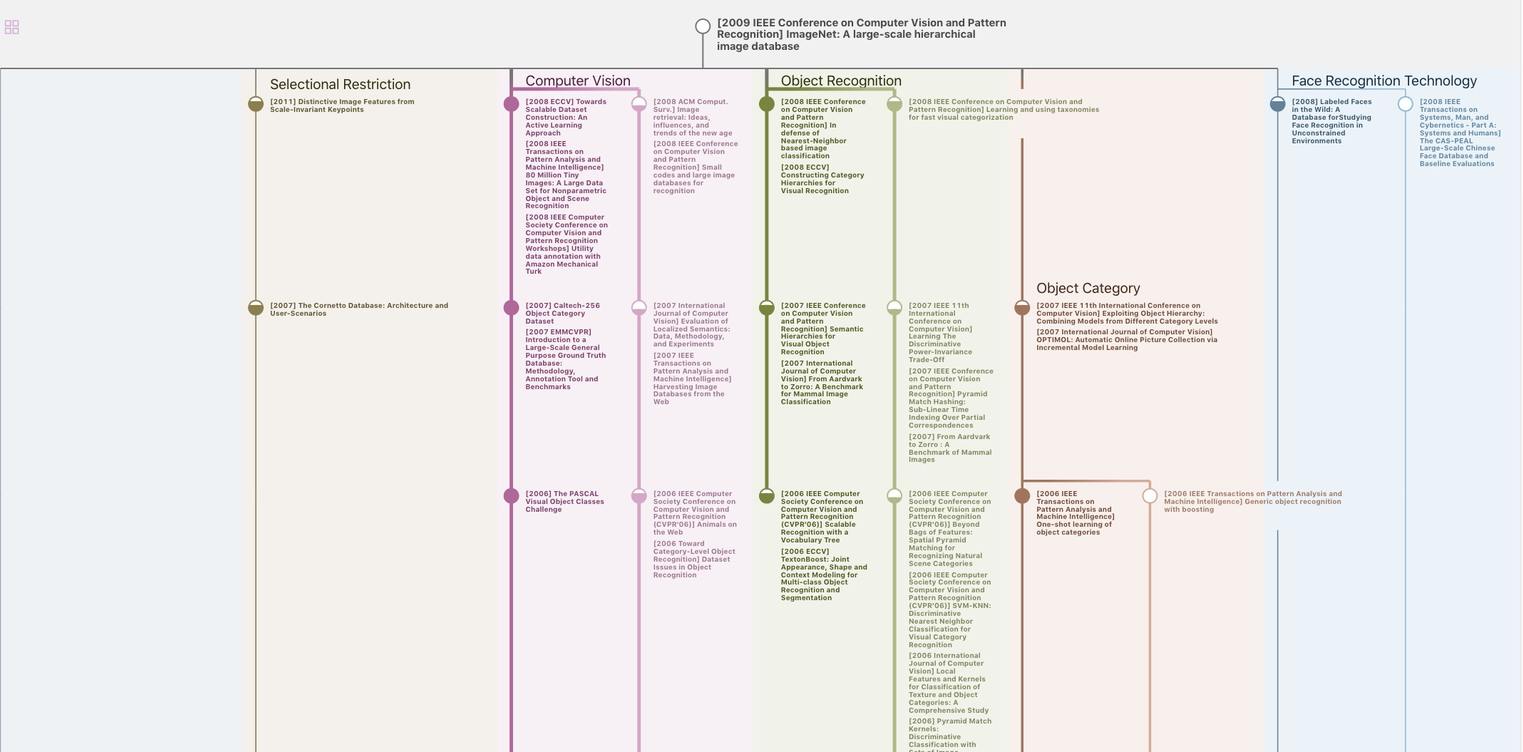
生成溯源树,研究论文发展脉络
Chat Paper
正在生成论文摘要