Bias-variance trade-off in portfolio optimization under expected shortfall with $ ewcommand{e}{{ m e}} {ell_2}$ regularization
JOURNAL OF STATISTICAL MECHANICS-THEORY AND EXPERIMENT(2019)
摘要
The optimization of a large random portfolio under the expected shortfall risk measure with an l(2) regularizer is carried out by analytical calculation for the case of uncorrelated Gaussian returns. The regularizer reins in the large sample fluctuations and the concomitant divergent estimation error, and eliminates the phase transition where this error would otherwise blow up. In the data-dominated region, where the number N of different assets in the portfolio is much less than the length T of the available time series, the regularizer plays a negligible role even if its strength eta is large, while in the opposite limit, where the size of samples is comparable to, or even smaller than the number of assets, the optimum is almost entirely determined by the regularizer. We construct the contour map of estimation error on the N/T versus eta plane and find that for a given value of the estimation error the gain in N/T due to the regularizer can reach a factor of about four for a sufficiently strong regularizer.
更多查看译文
关键词
quantitative finance,risk measure and management,cavity and replica method
AI 理解论文
溯源树
样例
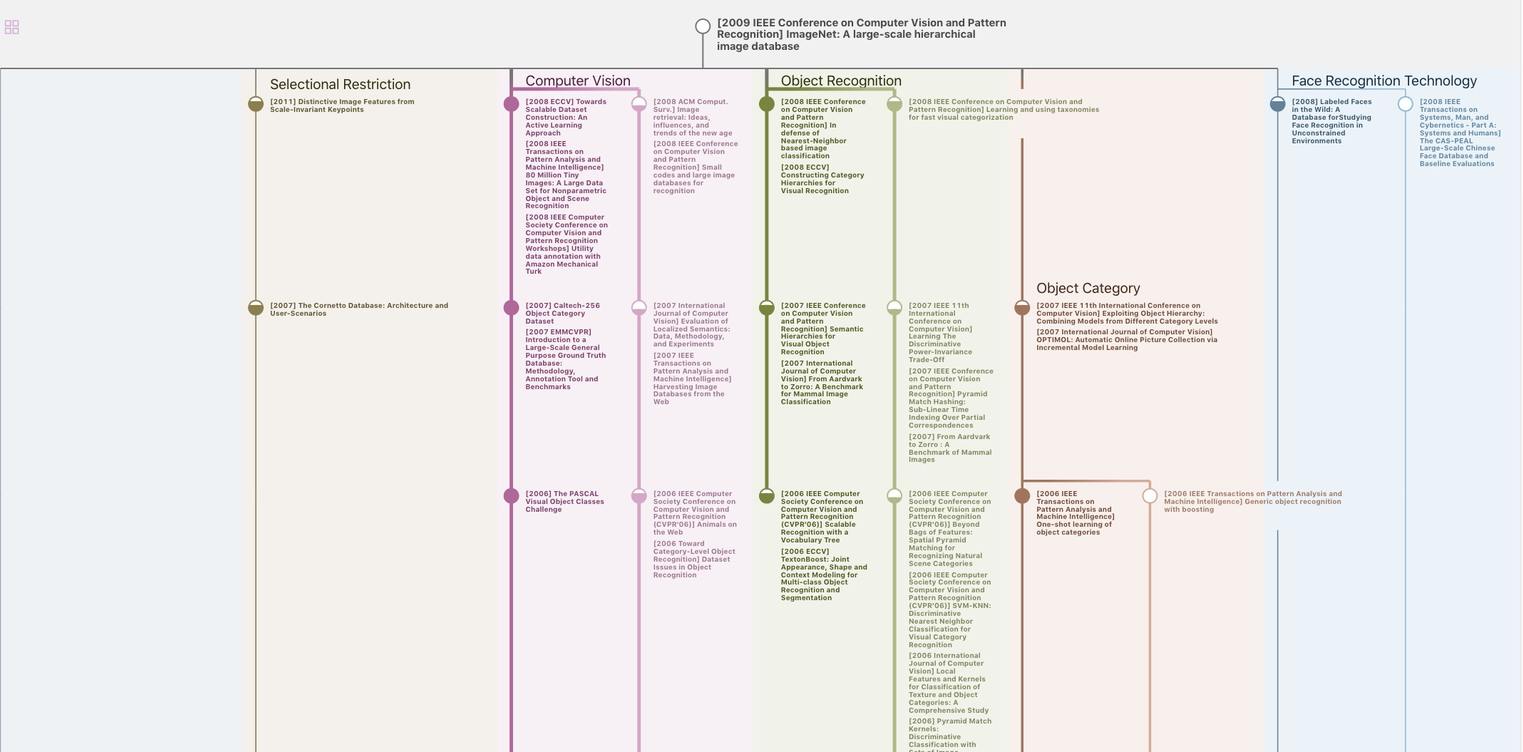
生成溯源树,研究论文发展脉络
Chat Paper
正在生成论文摘要