Impulsive Noise Detection In Ofdm-Based Systems: A Deep Learning Perspective
2019 IEEE 9TH ANNUAL COMPUTING AND COMMUNICATION WORKSHOP AND CONFERENCE (CCWC)(2019)
摘要
Efficient removal of impulsive noise (IN) from received signal is essential in many communication applications. In this paper, we propose a two stage IN mitigation approach for orthogonal frequency-division multiplexing (OFDM)-based communication systems. In the first stage, a deep neural network (DNN) is used to detect the instances of impulsivity. Then, the detected IN is blanked in the suppression stage to alleviate the harmful effects of outliers. Simulation results demonstrate the superior bit error rate (BER) performance of this approach relative to classic approaches such as blanking and clipping that use threshold to detect the IN. We demonstrate the robustness of the DNN-based approach under (i) mismatch between IN models considered for training and testing, and (ii) bursty impulsive environment when the receiver is empowered with interleaving techniques.
更多查看译文
关键词
Impulsive noise (IN), machine learning, deep neural network (DNN), orthogonal frequency-division multiplexing (OFDM), Detection, Mitigation
AI 理解论文
溯源树
样例
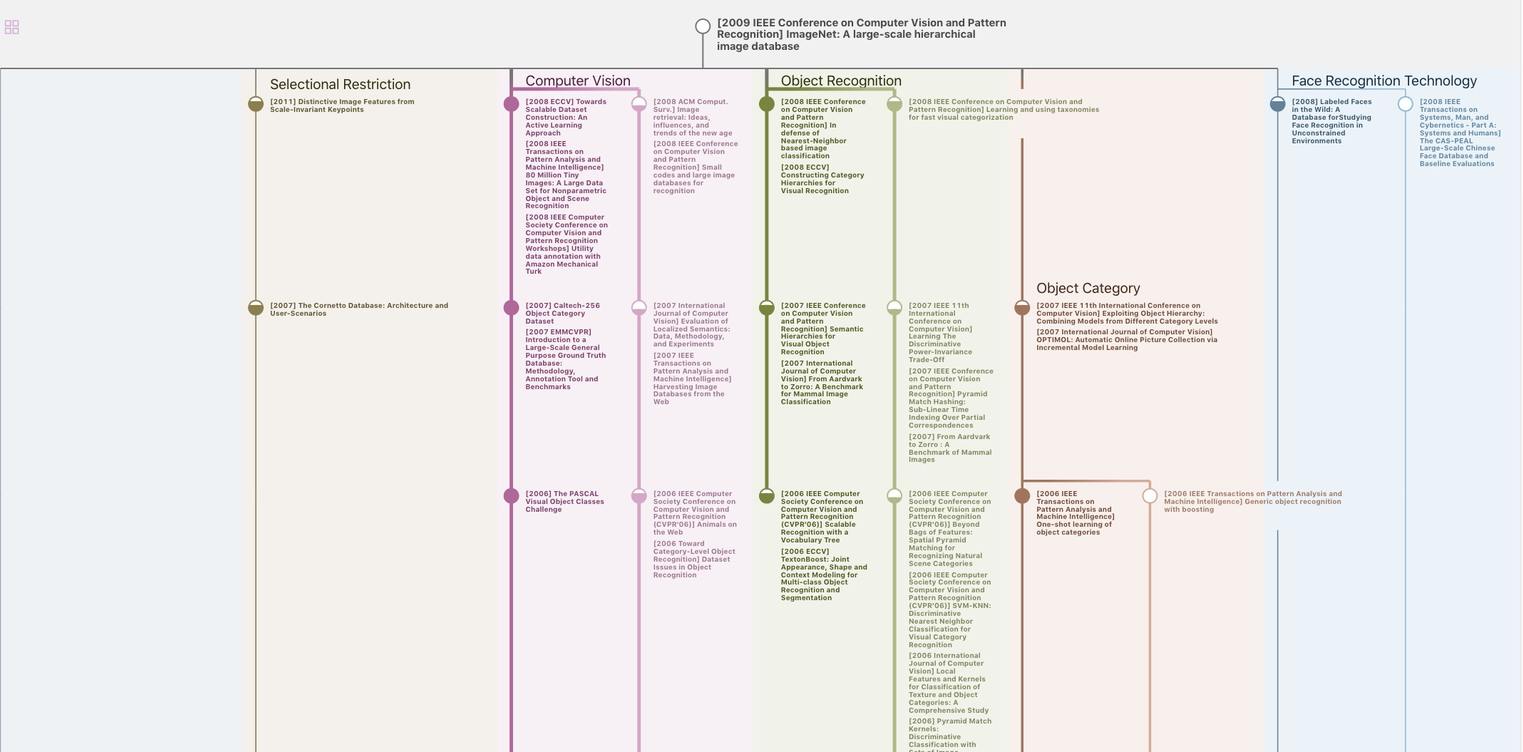
生成溯源树,研究论文发展脉络
Chat Paper
正在生成论文摘要