Anomaly Detection for Power System Generation Control based on Hierarchical DBSCAN
North American Power Symposium(2018)
摘要
The generation level of a generator in power grid is under control of multiple control loops such as governor action, Automatic Generation Control (AGC), and manual control. These control loops are vulnerable to cyber attacks and could become potential targets for the malicious adversary. In this paper, we investigated the detection of abnormal generation controls induced by cyber attacks. One data-driven anomaly detection methodology is presented based on the behavior conformity of generation units located in the same balancing authority. A semi supervised clustering algorithm with Hierarchical Density based Spatial Clustering of Application with Noise (HDBSCAN) is proposed for the detection model training. In our previous work, we obtained a synthetic dataset that covers scenarios including normal operation, generation control under ramp attack, switching attack, AGC integrity attacks, etc. and it is used to evaluate the proposed clustering algorithm. Experimental results show that the proposed algorithm provides better detection accuracy than K-means clustering and can distinguish not only between normal and abnormal generation controls but also among various anomaly scenarios.
更多查看译文
关键词
power system generation control,hierarchical DBSCAN,generation level,power grid,multiple control loops,governor action,manual control,cyber attacks,potential targets,malicious adversary,abnormal generation,data-driven anomaly detection methodology,generation units,semisupervised clustering algorithm,detection model training,ramp attack,AGC integrity attacks,detection accuracy,anomaly scenarios,automatic generation control,hierarchical density
AI 理解论文
溯源树
样例
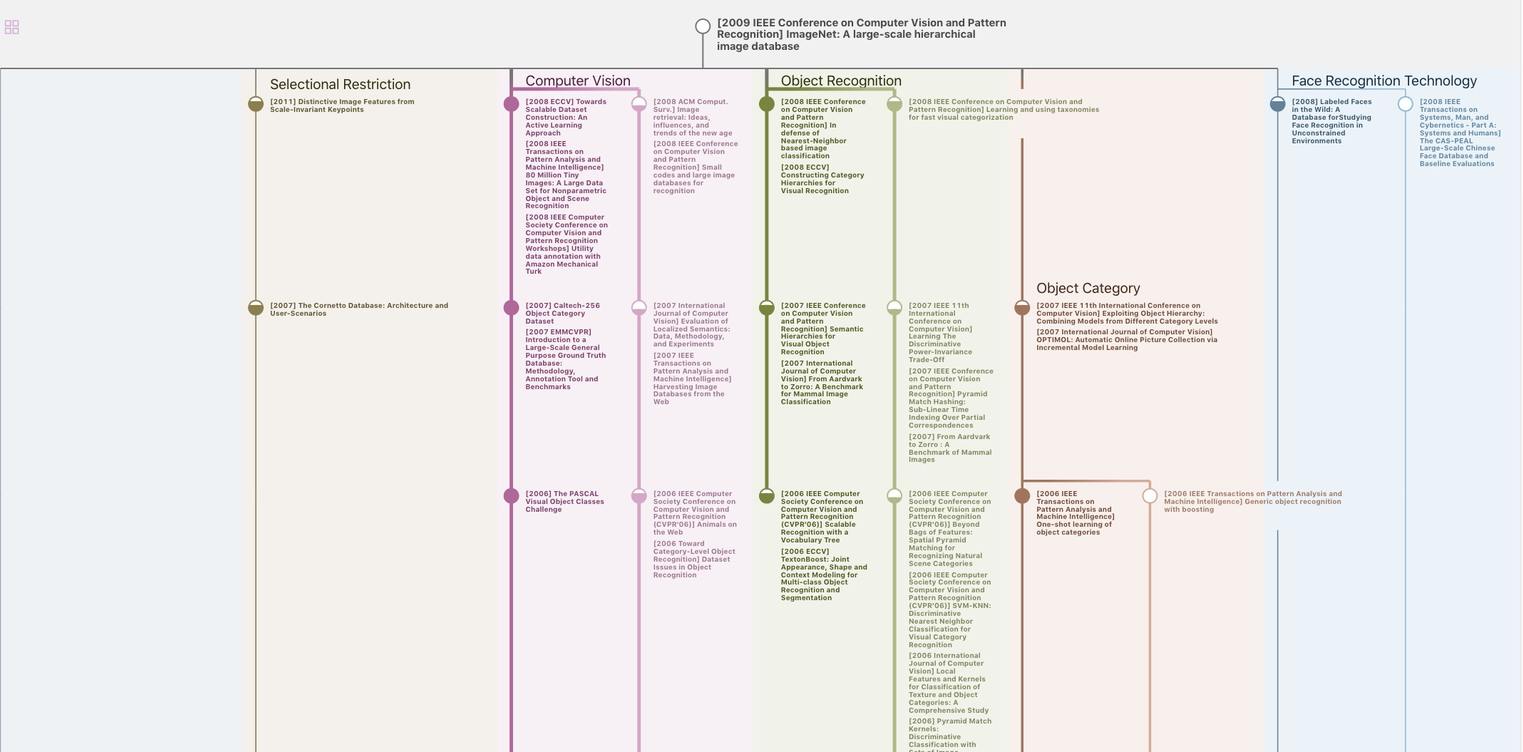
生成溯源树,研究论文发展脉络
Chat Paper
正在生成论文摘要