Short-term Power Load Probability Density Forecasting Based on PCA-QRF
2018 2nd IEEE Conference on Energy Internet and Energy System Integration (EI2)(2018)
Abstract
Power load probability density forecasting generates the probability and fluctuation range of forecasting value, which can offer more decision-making information for the security, reliability and economic operation of power system. A scientific and reasonable usage of multi-source, heterogeneous and massive power load and meteorological data can efficiently improve the accuracy of power load forecasting, but it also render the model training more complicated. This will lead us to a short-term power load probability density forecasting model based on principal component analysis and quantile regression forests. The principal component analysis, by means of linear transformation, turns the correlation input variables into heterogenous principal component, which can reduce the complexity and improve the forecasting accuracy. Quantile regression forests algorithm can obtain the conditional distribution of forecasting value and it can also, by means of kernel density estimation, obtain the power load probability density forecasting result at any time.
MoreTranslated text
Key words
short-term power load forecasting,probability density,principal component analysis,quantile regression forests,kernel density estimation
AI Read Science
Must-Reading Tree
Example
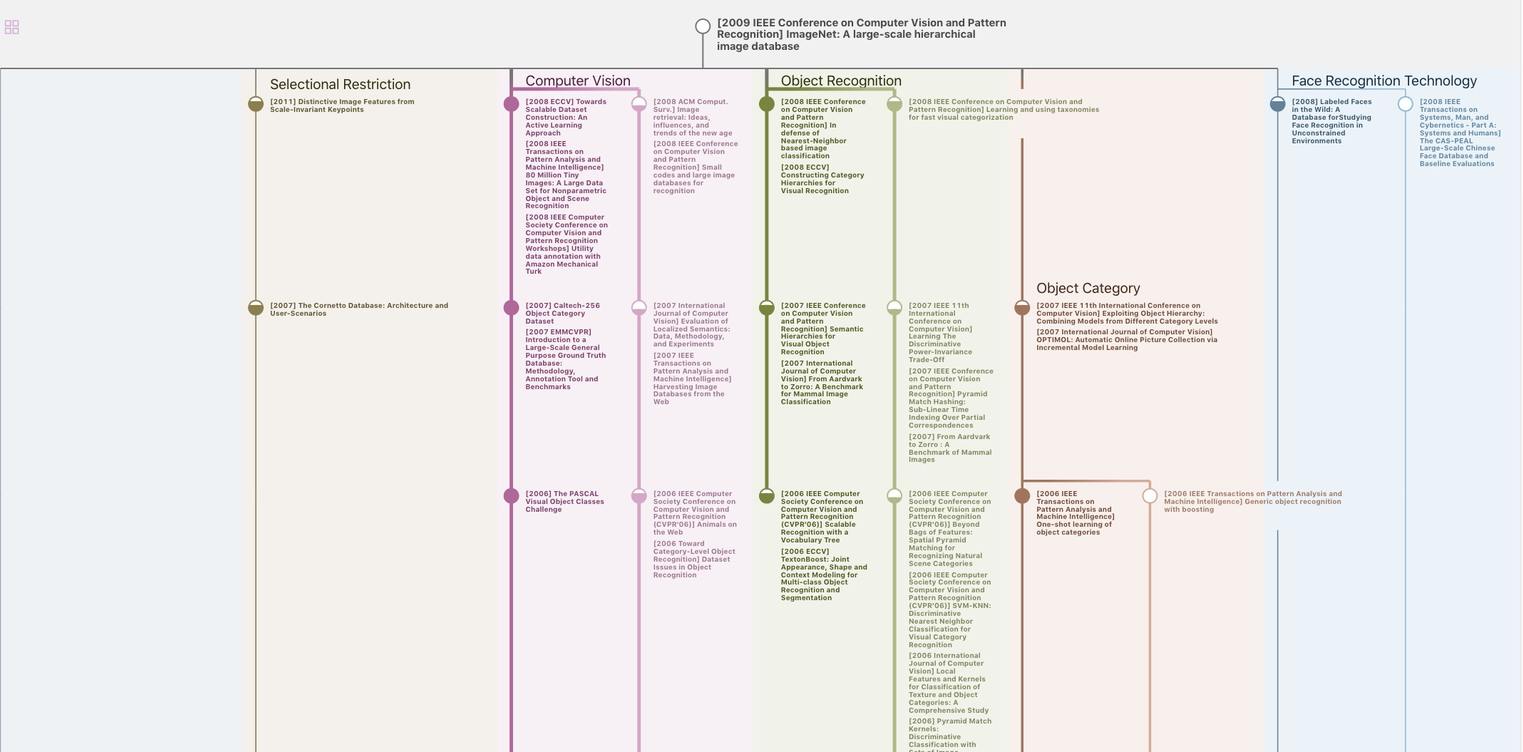
Generate MRT to find the research sequence of this paper
Chat Paper
Summary is being generated by the instructions you defined