Extraction of urban built-up areas from nighttime lights using artificial neural network
GEOCARTO INTERNATIONAL(2020)
Abstract
The spatial distribution of urban areas at the national and regional scales is critical for urban planners and governments to design sustainable and environment-friendly future development plans. The nighttime lights (NTL) data provide an effective way to monitor the urban at different scales however is usually achieved by using empirical threshold-based algorithms. This study proposed a novel Artificial Neural Network (ANN) approach, using moderate resolution imageries as NTL, MODIS NDVI and land surface temperature data, to map urban areas. Both random and maximum dissimilarity distance algorithm sampling methods were considered and compared. The validation of the urban areas extracted from MDA-based ANN against the 2011 US national land cover data showed a reasonable quality (overall accuracy = 97.84; Kappa = 0.74) and achieved more accurate result than the threshold method. This study demonstrates that ANN can provide an effective, rapid, and accurate alternative in extracting urban built-up areas from NTL.
MoreTranslated text
Key words
Urban area,nighttime light,artificial neural network,maximum dissimilarity distance algorithm
AI Read Science
Must-Reading Tree
Example
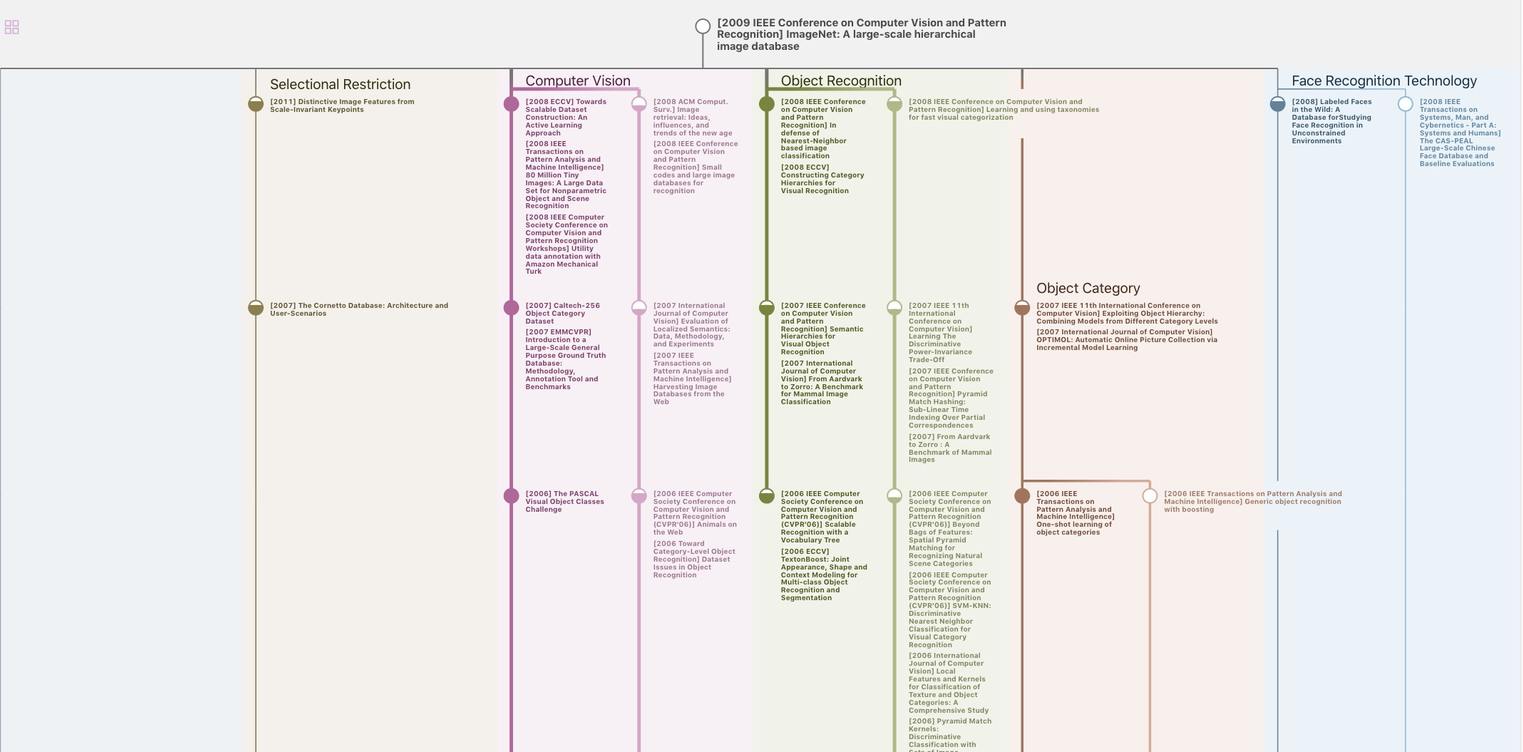
Generate MRT to find the research sequence of this paper
Chat Paper
Summary is being generated by the instructions you defined