Lazy-CFR: fast and near optimal regret minimization for extensive games with imperfect information.
arXiv: Learning(2018)
摘要
Counterfactual regret minimization (CFR) is the most popular algorithm on solving two-player zero-sum extensive games with imperfect information and achieves state-of-the-art performance in practice. However, the performance of CFR is not fully understood, since empirical results on the regret are much better than the upper bound proved in cite{zinkevich2008regret}. Another issue is that CFR has to traverse the whole game tree in each round, which is time-consuming in large scale games. In this paper, we present a novel technique, lazy update, which can avoid traversing the whole game tree in CFR, as well as a novel analysis on the regret of CFR with lazy update. Our analysis can also be applied to the vanilla CFR, resulting in a much tighter regret bound than that in cite{zinkevich2008regret}. Inspired by lazy update, we further present a novel CFR variant, named Lazy-CFR. Compared to traversing $O(|mathcal{I}|)$ information sets in vanilla CFR, Lazy-CFR needs only to traverse $O(sqrt{|mathcal{I}|})$ information sets per round while keeping the regret bound almost the same, where $mathcal{I}$ is the class of all information sets. As a result, Lazy-CFR shows better convergence result compared with vanilla CFR. Experimental results consistently show that Lazy-CFR outperforms the vanilla CFR significantly.
更多查看译文
AI 理解论文
溯源树
样例
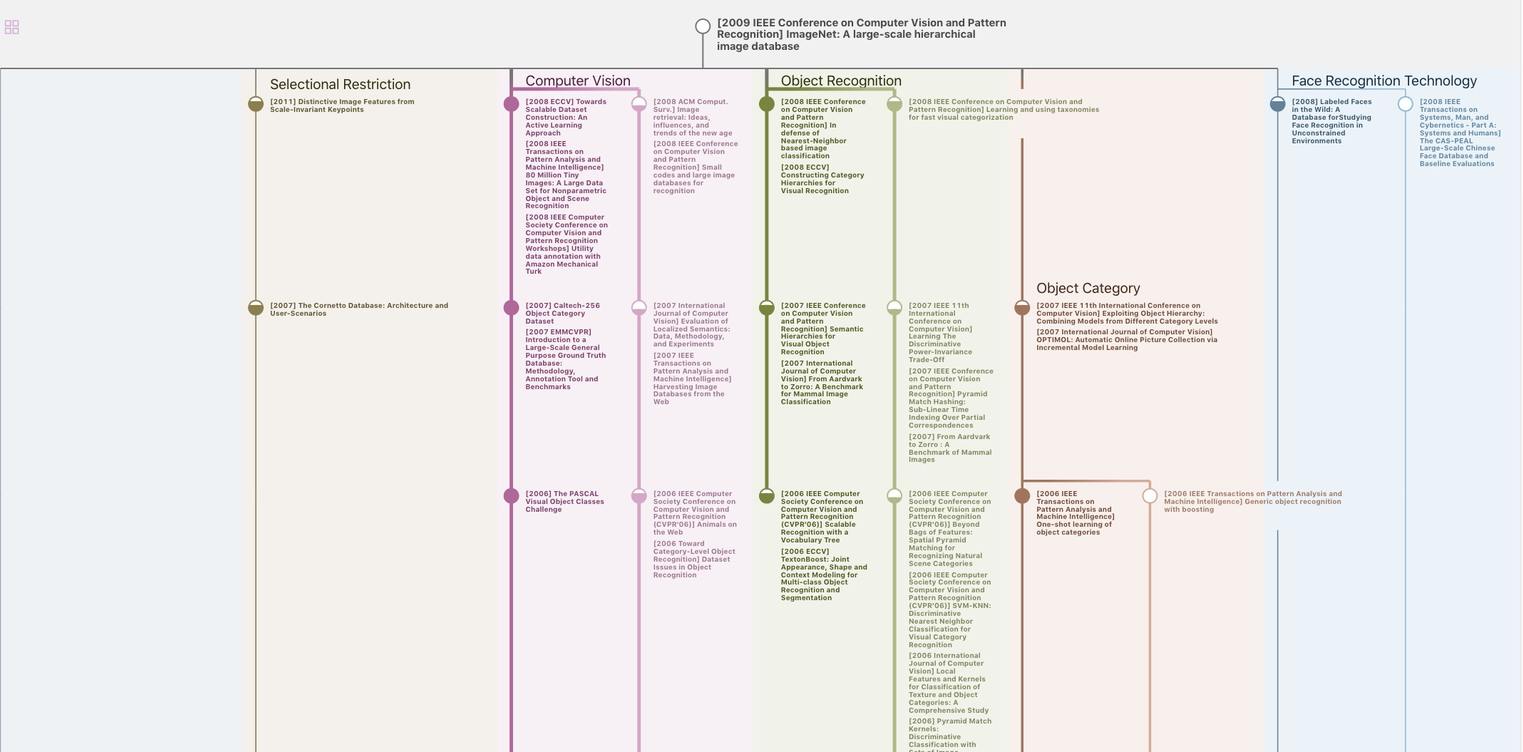
生成溯源树,研究论文发展脉络
Chat Paper
正在生成论文摘要