Arrhythmia detection by extracting hybrid features based on refined Fuzzy entropy (FuzEn) approach and employing machine learning techniques
WAVES IN RANDOM AND COMPLEX MEDIA(2020)
摘要
Cardiac arrhythmias are disturbances in the rhythm of the heart manifested by irregularity or by abnormally fast rates ('tachycardia') or abnormally slow rates ('bradycardias'). In the past researchers extracted different features extracting strategies to detect the arrhythmia. Since, signals acquired from subjects suffered with arrhythmia are multivariate, highly nonlinear, nonstationary, time variant and very complex. Traditional features extraction approaches could not capture full dynamics to detect these signals. In this study, we extracted hybrid features based on refined sample entropy (SampEn) and Fuzzy entropy (FuzEn) computed on mean, standard deviation and variance in combination with traditional features. The machine learning support vector machines with its kernels, decision tree, KNN and ensemble methods were employed to detect the arrhythmia. The 10-fold cross-validation was used for training/validation of data. The Support Vector Machine and its kernels, Fine KNN, and Ensemble Boosted Tree give the highest performance with an overall accuracy of 100%, AUC of 1.00. An accuracy of 95.5% and 100% was obtained using SVM coarse Gaussian, 97% and 100% using Fine KNN, 95.5% and 97% using Decision tree with traditional and hybrid features respectively. The results revealed that SVM coarse Gaussian, Coarse KNN, Decision tree methods outer performed using hybrid features than the traditional feature extracting strategy.
更多查看译文
关键词
Refined Fuzzy entropy (FuzEn),arrhythmia,Support Vector Machine (SVM),Decision tree (DT),K-nearest neighbours (KNN),ensemble methods
AI 理解论文
溯源树
样例
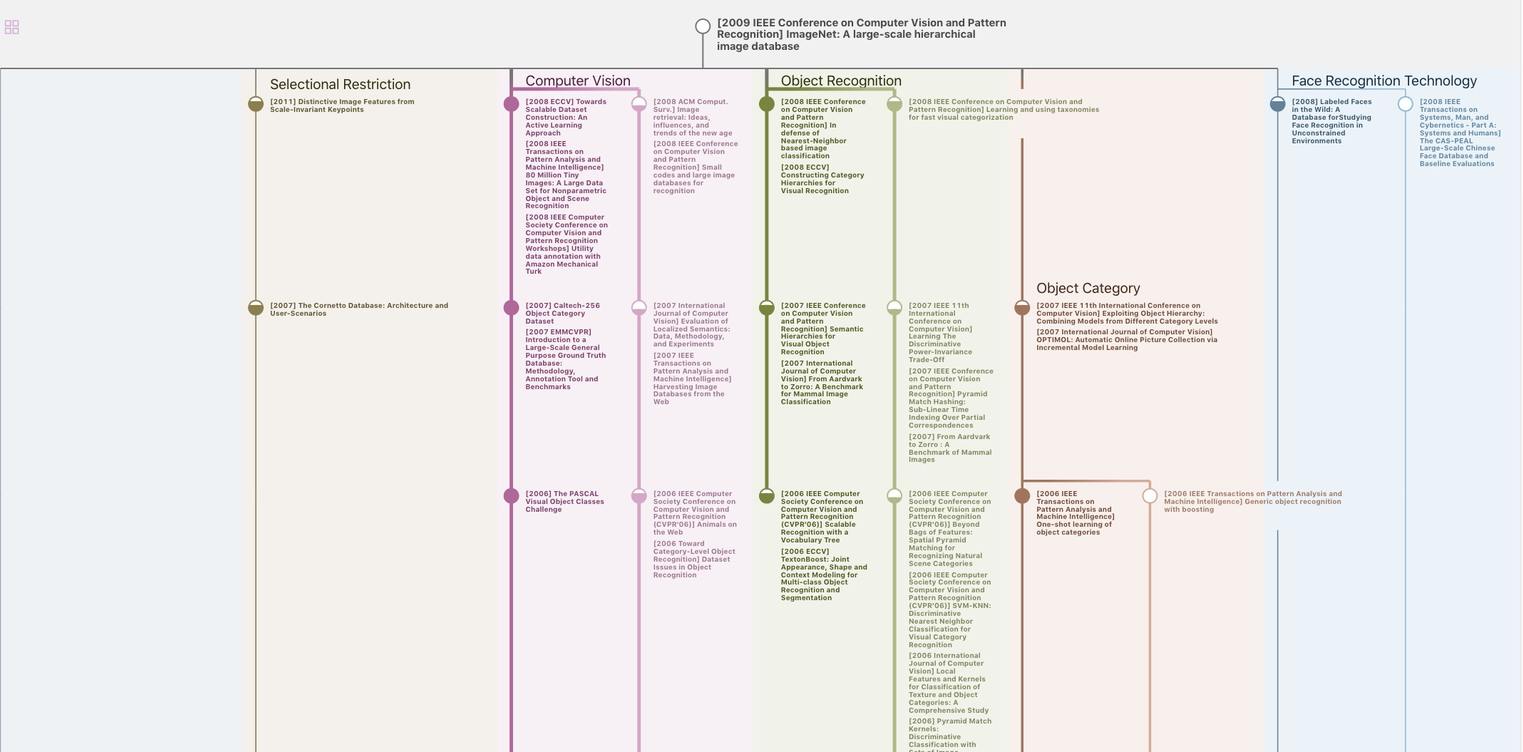
生成溯源树,研究论文发展脉络
Chat Paper
正在生成论文摘要