Autonomous Feature Type Selection Based On Environment Using Expectation Maximization In Self-Localization
INTERNATIONAL JOURNAL OF ADVANCED ROBOTIC SYSTEMS(2018)
摘要
Self-localization in autonomous robots is one of the fundamental issues in the development of intelligent robots, and processing of raw sensory information into useful features is an integral part of this problem. In a typical scenario, there are several choices for the feature extraction algorithm, and each has its weaknesses and strengths depending on the characteristics of the environment. In this work, we introduce a localization algorithm that is capable of capturing the quality of a feature type based on the local environment and makes soft selection of feature types throughout different regions. A batch expectation-maximization algorithm is developed for both discrete and Monte Carlo localization models, exploiting the probabilistic pose estimations of the robot without requiring ground truth poses and also considering different observation types as blackbox algorithms. We tested our method in simulations, data collected from an indoor environment with a custom robot platform and a public data set. The results are compared with the individual feature types as well as naive fusion strategy.
更多查看译文
关键词
Localization, feature selection, expectation maximization, bag of words
AI 理解论文
溯源树
样例
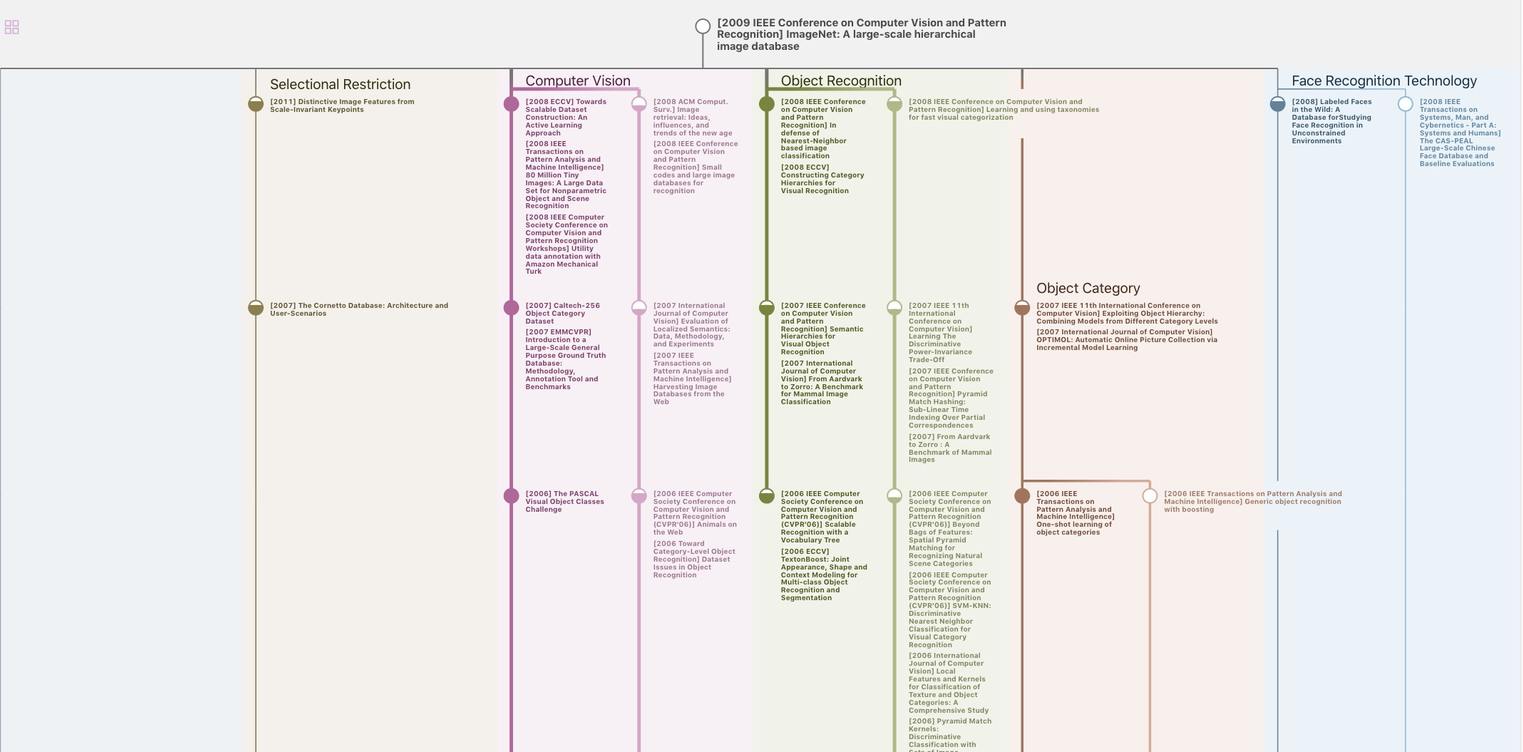
生成溯源树,研究论文发展脉络
Chat Paper
正在生成论文摘要