Attacking Data Transforming Learners at Training Time
THIRTY-THIRD AAAI CONFERENCE ON ARTIFICIAL INTELLIGENCE / THIRTY-FIRST INNOVATIVE APPLICATIONS OF ARTIFICIAL INTELLIGENCE CONFERENCE / NINTH AAAI SYMPOSIUM ON EDUCATIONAL ADVANCES IN ARTIFICIAL INTELLIGENCE(2019)
摘要
While machine learning systems are known to be vulnerable to data-manipulation attacks at both training and deployment time, little is known about how to adapt attacks when the defender transforms data prior to model estimation. We consider the setting where the defender Bob first transforms the data then learns a model from the result; Alice, the attacker, perturbs Bob's input data prior to him transforming it. We develop a general-purpose "plug and play" framework for gradient-based attacks based on matrix differentials, focusing on ordinary least-squares linear regression. This allows learning algorithms and data transformations to be paired and composed arbitrarily: attacks can be adapted through the use of the chain rule-analogous to backpropagation on neural network parameters-to compositional learning maps. Best-response attacks can be computed through matrix multiplications from a library of attack matrices for transformations and learners. Our treatment of linear regression extends state-of-the- art attacks at training time, by permitting the attacker to affect both features and targets optimally and simultaneously. We explore several transformations broadly used across machine learning with a driving motivation for our work being autogressive modeling. There, Bob transforms a univariate time series into a matrix of observations and vector of target values which can then be fed into standard learners. Under this learning reduction, a perturbation from Alice to a single value of the time series affects features of several data points along with target values.
更多查看译文
关键词
data transforming learners,training time
AI 理解论文
溯源树
样例
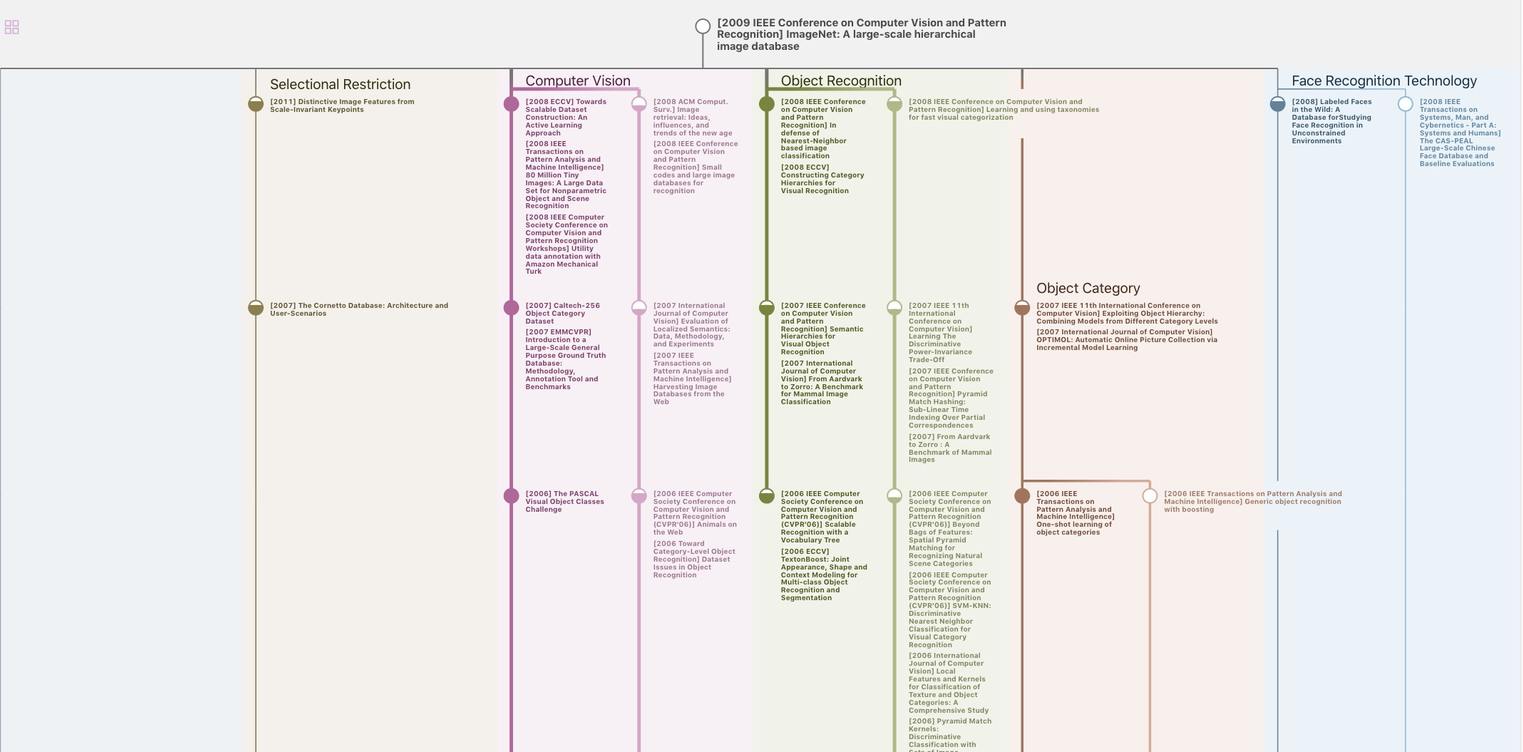
生成溯源树,研究论文发展脉络
Chat Paper
正在生成论文摘要