Quantitative prediction of the aged state of Ni-base superalloys using PCA and tensor regression
Acta Materialia(2019)
摘要
The microstructure of Ni-base superalloy components evolves and degrades during the operation of gas turbines. Since the remaining life depends on the degradation, it is highly desirable to have a quantitative descriptor of the aged state of the microstructure that can be linked to the operating conditions. In this paper, data analytics algorithms are used to develop such relationships. High-throughput aging experiments were performed to generate a dataset comprising multiple aged microstructure images. The digital images of the γ/γ′ phase are used as an indicator of the aged state and statistically evaluated using 2-point spatial correlation functions. To reduce the high-dimensional structural information so that a quantitative linkage can be made between aging conditions and the aged state, two algorithms were considered. The first algorithm involves two steps, first using conventional principal component analysis (PCA) to provide a lower dimension descriptor of the microstructure and then regression analysis to generate the linkage. The second algorithm, called tensor regression (TR), is a novel algorithm that merges the dimensionality reduction and model construction step into a single step. The output of the TR model is directly the statistical descriptors of the microstructure rather than the PC scores, thereby reducing the amount of information loss. Even though PCA provides an effective tool for visualization and classification of data, the model built based on the TR algorithm is shown to have stronger prediction capability.
更多查看译文
关键词
Ni-base superalloys,Aging,Process-structure relations,Principal component analysis,Tensor regression
AI 理解论文
溯源树
样例
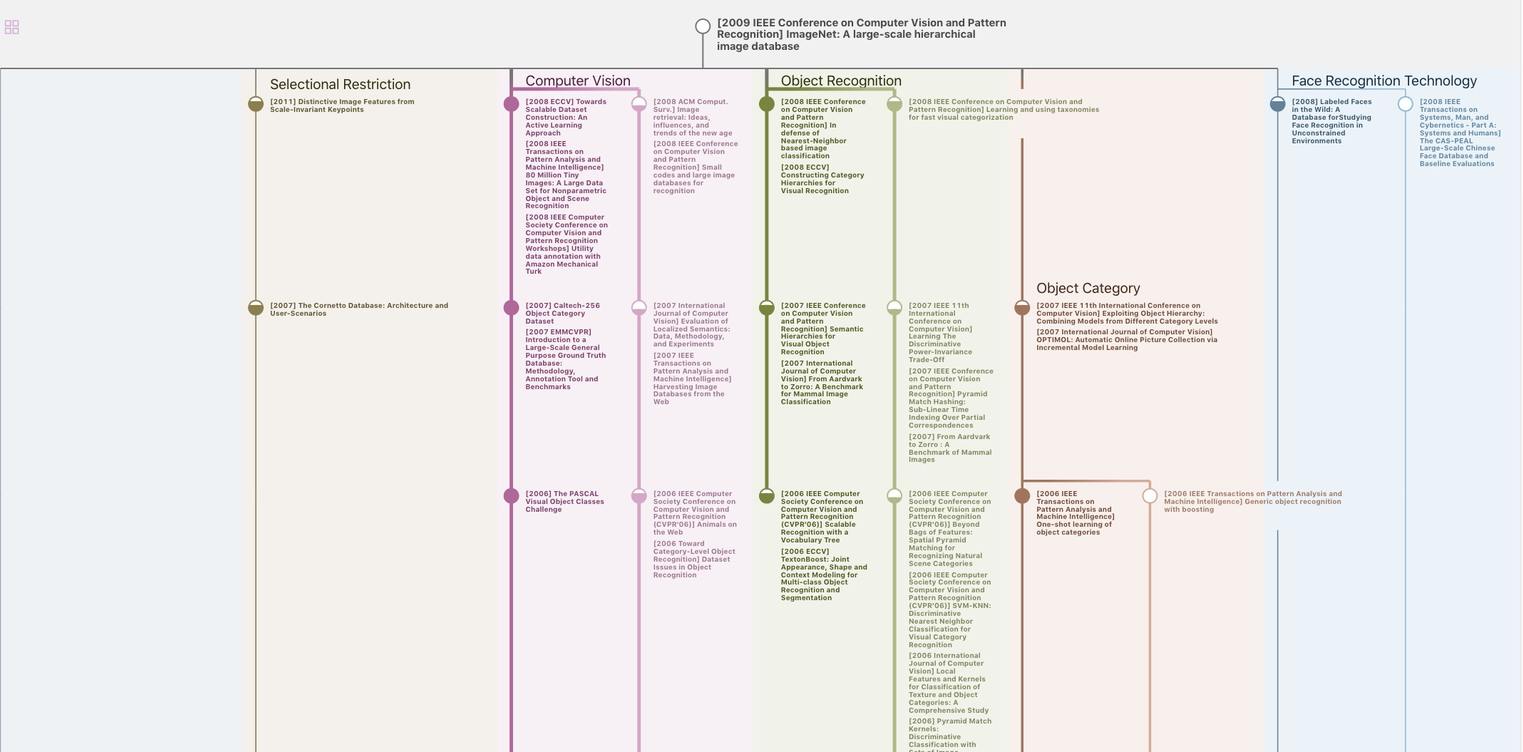
生成溯源树,研究论文发展脉络
Chat Paper
正在生成论文摘要