Hypothesis Tests That Are Robust to Choice of Matching Method
arXiv: Methodology(2018)
摘要
A vast number of causal inference studies test hypotheses on treatment effects after treatment cases are matched with similar control cases. The quality of matched data is usually evaluated according to some metric, such as balance; however the same level of match quality can be achieved by different matches on the same data. Crucially, matches that achieve the same level of quality might lead to different results for hypothesis tests conducted on the matched data. Experimenters often specifically choose not to consider the uncertainty stemming from how the matches were constructed; this allows for easier computation and clearer testing, but it does not consider possible biases in the way the assignments were constructed. What we would really like to be able to report is that no matter which assignment we choose, as long as the match is sufficiently good, then the hypothesis test result still holds. In this paper, we provide methodology based on discrete optimization to create robust tests that explicitly account for this variation. For binary data, we give both fast algorithms to compute our tests and formulas for the null distributions of our test statistics under different conceptions of matching. For continuous data, we formulate a robust test statistic, and offer a linearization that permits faster computation. We apply our methods to real-world datasets and show that they can produce useful results in practical applied settings.
更多查看译文
关键词
matching,hypothesis tests
AI 理解论文
溯源树
样例
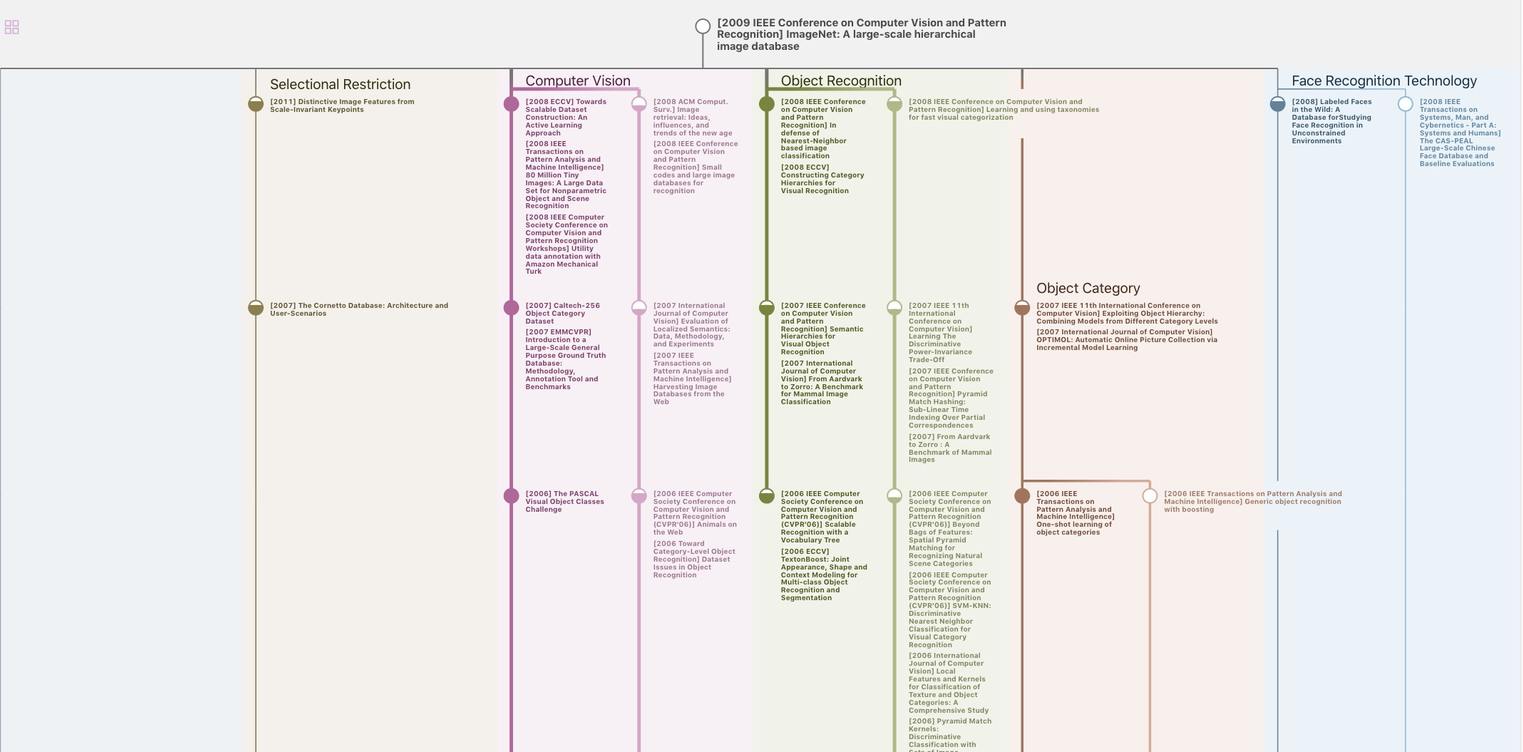
生成溯源树,研究论文发展脉络
Chat Paper
正在生成论文摘要