Comparison of two gap-filling techniques for nitrous oxide fluxes from agricultural soil
CANADIAN JOURNAL OF SOIL SCIENCE(2019)
Abstract
Micrometeorological methods are ideally suited for continuous measurements of N2O fluxes, but gaps in the time series occur due to low-turbulence conditions, power failures, and adverse weather conditions. Two gap-filling methods including linear interpolation and artificial neural networks (ANN) were utilized to reconstruct missing N2O flux data from a corn-soybean-wheat rotation and evaluate the impact on annual N2O emissions from 2001 to 2006 at the Elora Research Station, ON, Canada. The single-year ANN method is recommended because this method captured flux variability better than the linear interpolation method (average R-2 of 0.41 vs. 0.34). Annual N2O emission and annual bias resulting from linear and single-year ANN were compatible with each other when there were few and short gaps (i.e., percentage of missing values <30%). However, with longer gaps (>20 d), the bias error in annual fluxes varied between 0.082 and 0.344 kg N2O-N ha(-1) for linear and 0.069 and 0.109 kg N2O-N ha(-1) for single-year ANN. Hence, the single-year ANN with lower annual bias and stable approach over various years is recommended, if the appropriate driving inputs (i.e., soil temperature, soil water content, precipitation, N mineral content, and snow depth) needed for the ANN model are available.
MoreTranslated text
Key words
gap filling,micrometeorological method,N2O flux,artificial neural networks,linear interpolation
AI Read Science
Must-Reading Tree
Example
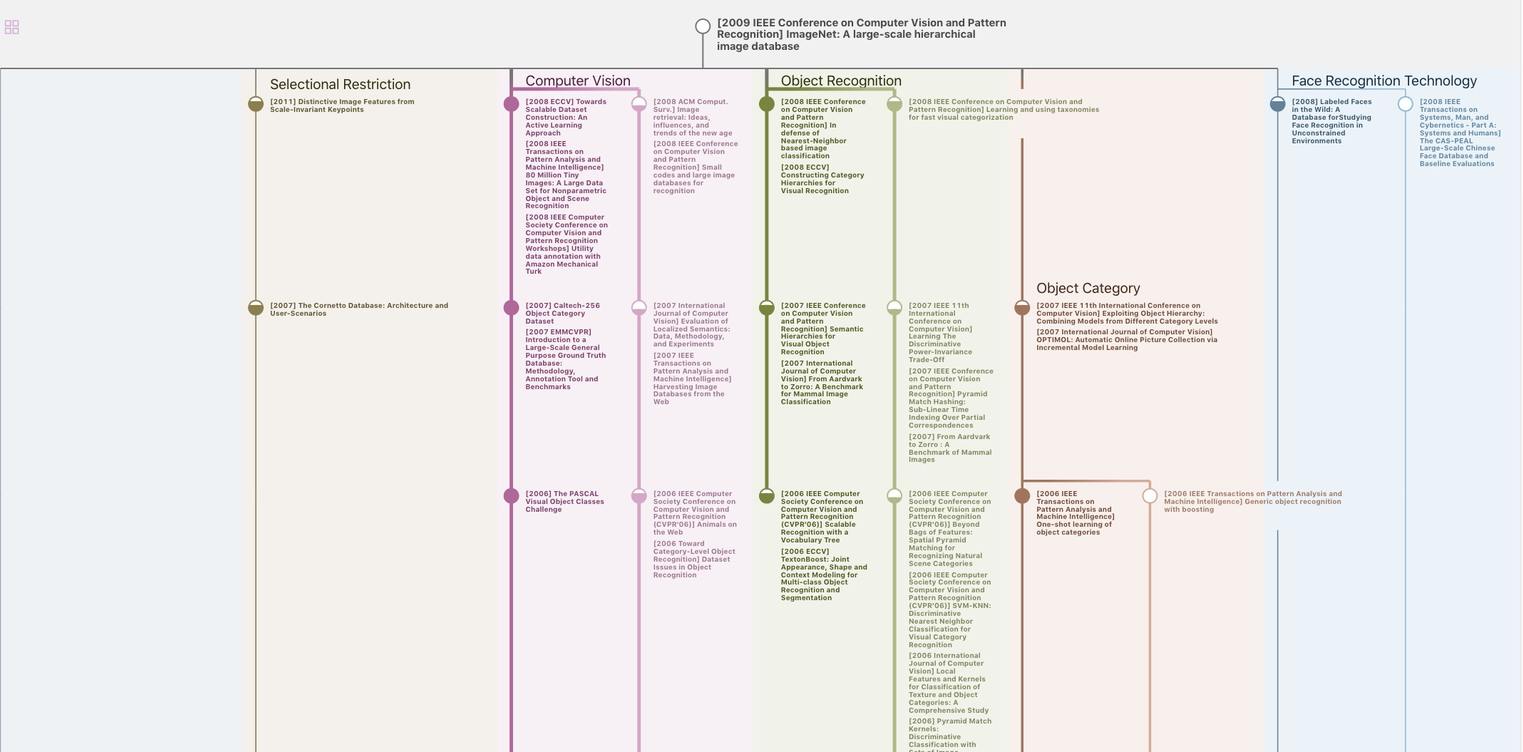
Generate MRT to find the research sequence of this paper
Chat Paper
Summary is being generated by the instructions you defined