Aligning And Updating Cadaster Maps With Aerial Images By Multi-Task, Multi-Resolution Deep Learning
COMPUTER VISION - ACCV 2018, PT V(2018)
Abstract
A large part of the world is already covered by maps of buildings, through projects such as OpenStreetMap. However when a new image of an already covered area is captured, it does not align perfectly with the buildings of the already existing map, due to a change of capture angle, atmospheric perturbations, human error when annotating buildings or lack of precision of the map data. Some of those deformations can be partially corrected, but not perfectly, which leads to misalignments. Additionally, new buildings can appear in the image. Leveraging multi-task learning, our deep learning model aligns the existing building polygons to the new image through a displacement output, and also detects new buildings that do not appear in the cadaster through a segmentation output. It uses multiple neural networks at successive resolutions to output a displacement field and a pixel-wise segmentation of the new buildings from coarser to finer scales. We also apply our method to buildings height estimation, by aligning cadaster data to the rooftops of stereo images. The code is available at https://github.com/Lydorn/mapalignment.
MoreTranslated text
Key words
Alignment, Registration, Multi-task, Multi-resolution
AI Read Science
Must-Reading Tree
Example
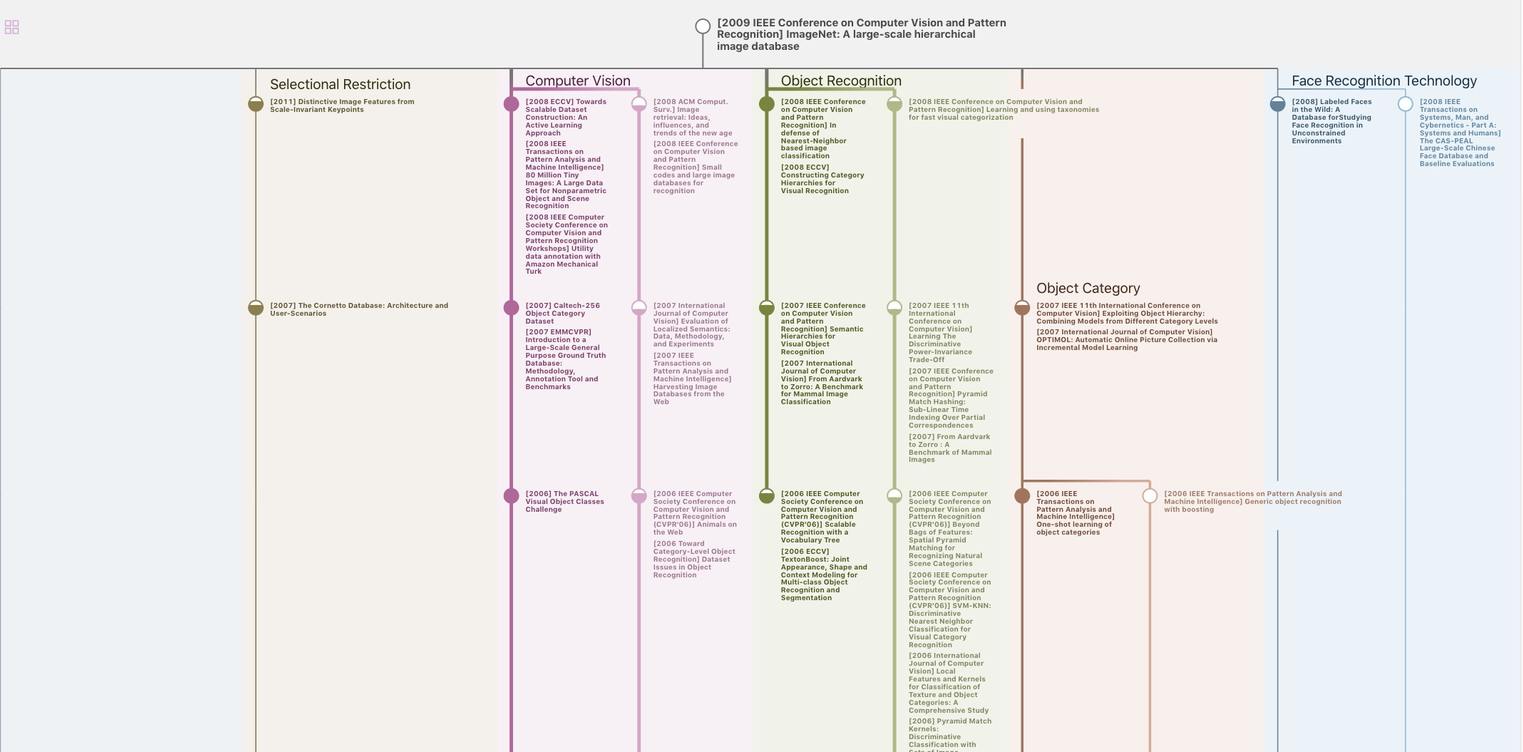
Generate MRT to find the research sequence of this paper
Chat Paper
Summary is being generated by the instructions you defined