A 68 mw 2.2 Tops/w low bit-width and multiplierless DCNN object detection processor for visually impaired people
IEEE Transactions on Circuits and Systems for Video Technology(2018)
Abstract
Deep convolutional neural network (DCNN) object detection is a powerful solution in visual perception, but requires huge computation and communication cost. We proposed a fast and low power always-on object detection processor for visually impaired people to understand their surroundings. We designed an automatic DCNN quantization algorithm which successfully quantizes the data to 8-bit fix-points with 32 values and uses 5-bit indexes to represent them, reducing hardware cost by over 68% compared to the 16-bit DCNN with negligible accuracy loss. A specific hardware accelerator is designed, which uses reconfigurable process engines (PEs) to realize multi-layer pipelines to significantly reduce or eliminate the off-chip temporary data transfer. Lookup table is used to implement all multiplications in convolutions to reduce power significantly. The design is fabricated in SMIC 55nm technology and the post-layout simulation shows only 68 mw power at 1.1v voltage with 155 Gops performance, achieving 2.2 Top/w energy efficiency.
MoreTranslated text
Key words
Quantization (signal),Hardware,Object detection,Optimization,Indexes,Computational efficiency,Energy efficiency
AI Read Science
Must-Reading Tree
Example
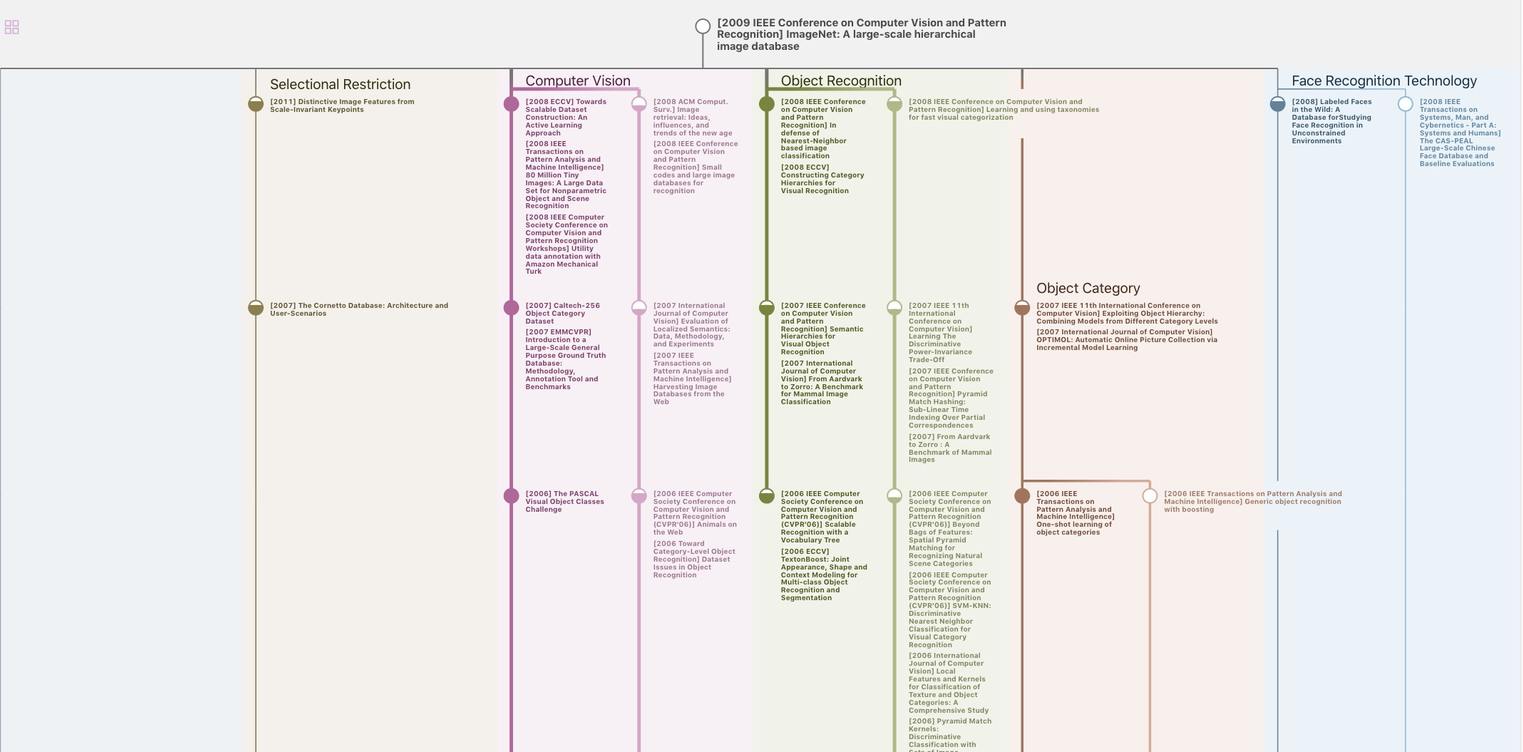
Generate MRT to find the research sequence of this paper
Chat Paper
Summary is being generated by the instructions you defined