Generic Paper and Plastic Recognition by Fusion of NIR and VIS Data and Redundancy-Aware Feature Ranking
PROCEEDINGS OF THE FUTURE TECHNOLOGIES CONFERENCE (FTC) 2018, VOL 1(2019)
摘要
Near infrared (NIR) spectroscopy is used in many applications to gather information about chemical composition of materials. For paper waste sorting, a small number of scores computed from NIR-spectra and assuming more or less unimodal clustered data, a pixel classifier can still be crafted by hand using knowledge about chemical properties and a reasonable amount of intuition. Additional information can be gained by visual data (VIS). However, it is not obvious what features, e.g. based on color, saturation, textured areas, are finally important for successfully separating the paper classes in feature space. Hence, a rigorous feature analysis becomes inevitable. We have chosen a generic machine-learning approach to successfully fuse NIR and VIS information. By exploiting a classification tree and a variety of additional visual features, we could increase the recognition rate to 78% for 11 classes, compared to 63% only using NIR scores. A modified feature ranking measure, which takes redundancies of features into account, allows us to analyze the importance of features and reduce them effectively. While some visual features like color saturation and hue showed to be important, some NIR scores could even be dropped. Finally, we generalize this approach to analyze raw NIR-spectra instead of score values and apply it to plastic waste sorting.
更多查看译文
关键词
Near Infrared (NIR) Spectroscopy,Waste sorting,Visual Features (VIS),CART,Feature ranking,Machine-learning
AI 理解论文
溯源树
样例
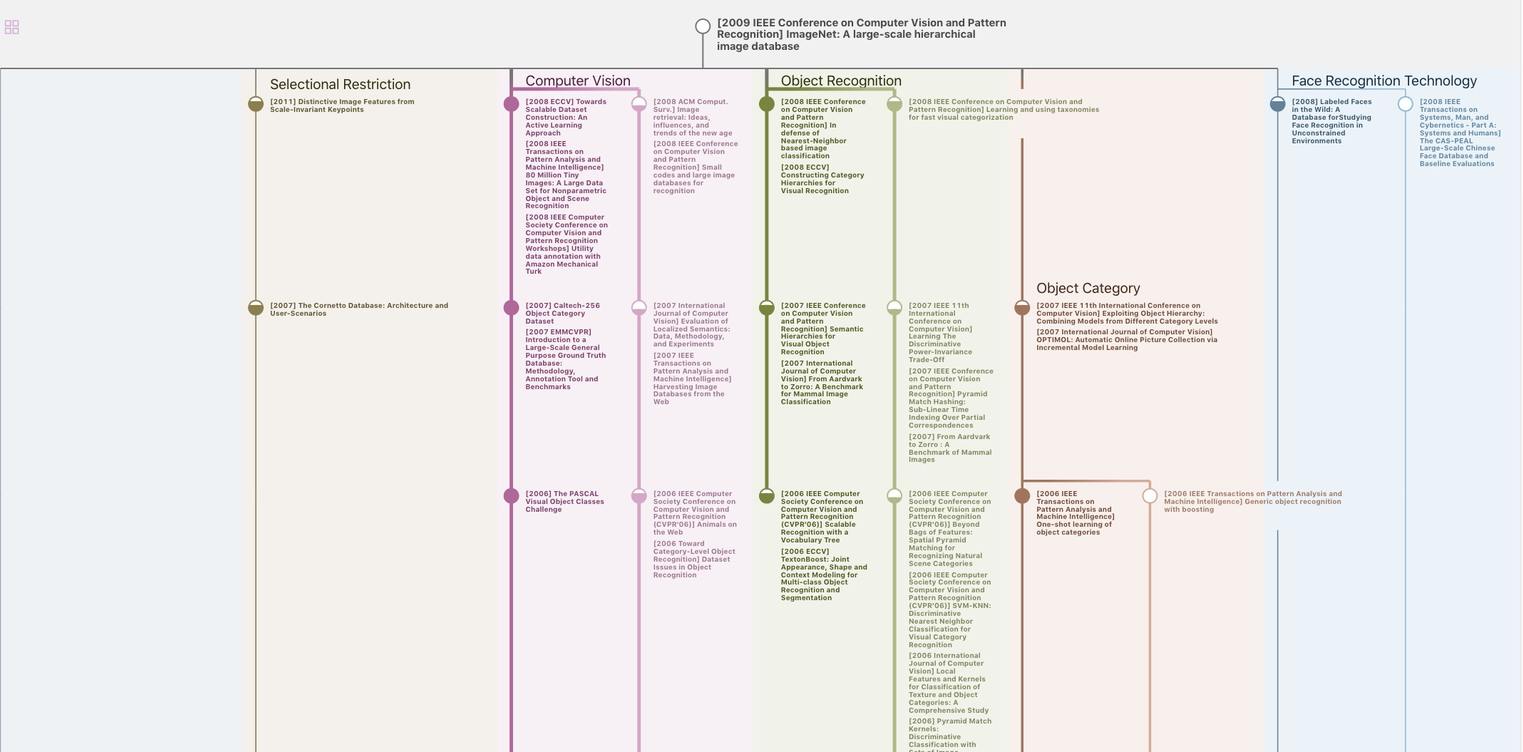
生成溯源树,研究论文发展脉络
Chat Paper
正在生成论文摘要